Constrained Reweighting of Distributions: An Optimal Transport Approach
ENTROPY(2024)
摘要
We commonly encounter the problem of identifying an optimally weight-adjusted version of the empirical distribution of observed data, adhering to predefined constraints on the weights. Such constraints often manifest as restrictions on the moments, tail behavior, shapes, number of modes, etc., of the resulting weight-adjusted empirical distribution. In this article, we substantially enhance the flexibility of such a methodology by introducing a nonparametrically imbued distributional constraint on the weights and developing a general framework leveraging the maximum entropy principle and tools from optimal transport. The key idea is to ensure that the maximum entropy weight-adjusted empirical distribution of the observed data is close to a pre-specified probability distribution in terms of the optimal transport metric, while allowing for subtle departures. The proposed scheme for the re-weighting of observations subject to constraints is reminiscent of the empirical likelihood and related ideas, but offers greater flexibility in applications where parametric distribution-guided constraints arise naturally. The versatility of the proposed framework is demonstrated in the context of three disparate applications where data re-weighting is warranted to satisfy side constraints on the optimization problem at the heart of the statistical task-namely, portfolio allocation, semi-parametric inference for complex surveys, and ensuring algorithmic fairness in machine learning algorithms.
更多查看译文
关键词
complex surveys,demographic parity,entropy,optimal transport,portfolio allocation
AI 理解论文
溯源树
样例
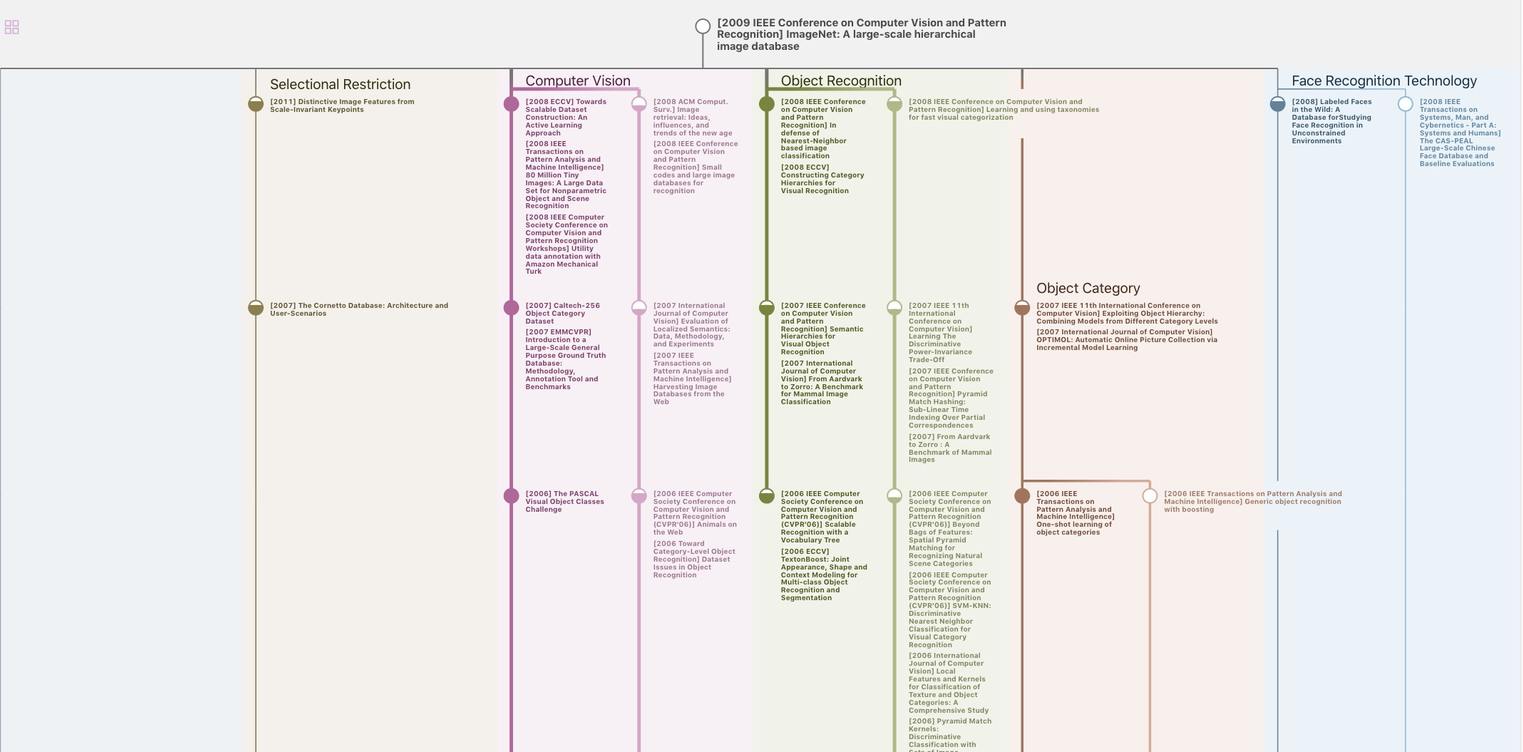
生成溯源树,研究论文发展脉络
Chat Paper
正在生成论文摘要