Modeling the ferroelectric phase transition in barium titanate with DFT accuracy and converged sampling
arXiv (Cornell University)(2023)
摘要
The accurate description of the structural and thermodynamic properties of ferroelectrics has been one of the most remarkable achievements of Density Functional Theory (DFT). However, running large simulation cells with DFT is computationally demanding, while simulations of small cells are often plagued with non-physical effects that are a consequence of the system's finite size. Therefore, one is often forced to use empirical models that describe the physics of the material in terms of effective interaction terms, that are fitted using the results from DFT, to perform simulations that do not suffer from finite size effects. In this study we use a machine-learning (ML) potential trained on DFT, in combination with accelerated sampling techniques, to converge the thermodynamic properties of Barium Titanate (BTO) with first-principles accuracy and a full atomistic description. Our results indicate that the predicted Curie temperature depends strongly on the choice of DFT functional and system size, due to the presence of emergent long-range directional correlations in the local dipole fluctuations. Our findings demonstrate how the combination of ML models and traditional bottom-up modeling allow one to investigate emergent phenomena with the accuracy of first-principles calculations and the large size and time scales afforded by empirical models.
更多查看译文
关键词
ferroelectric phase transition,barium titanate,dft
AI 理解论文
溯源树
样例
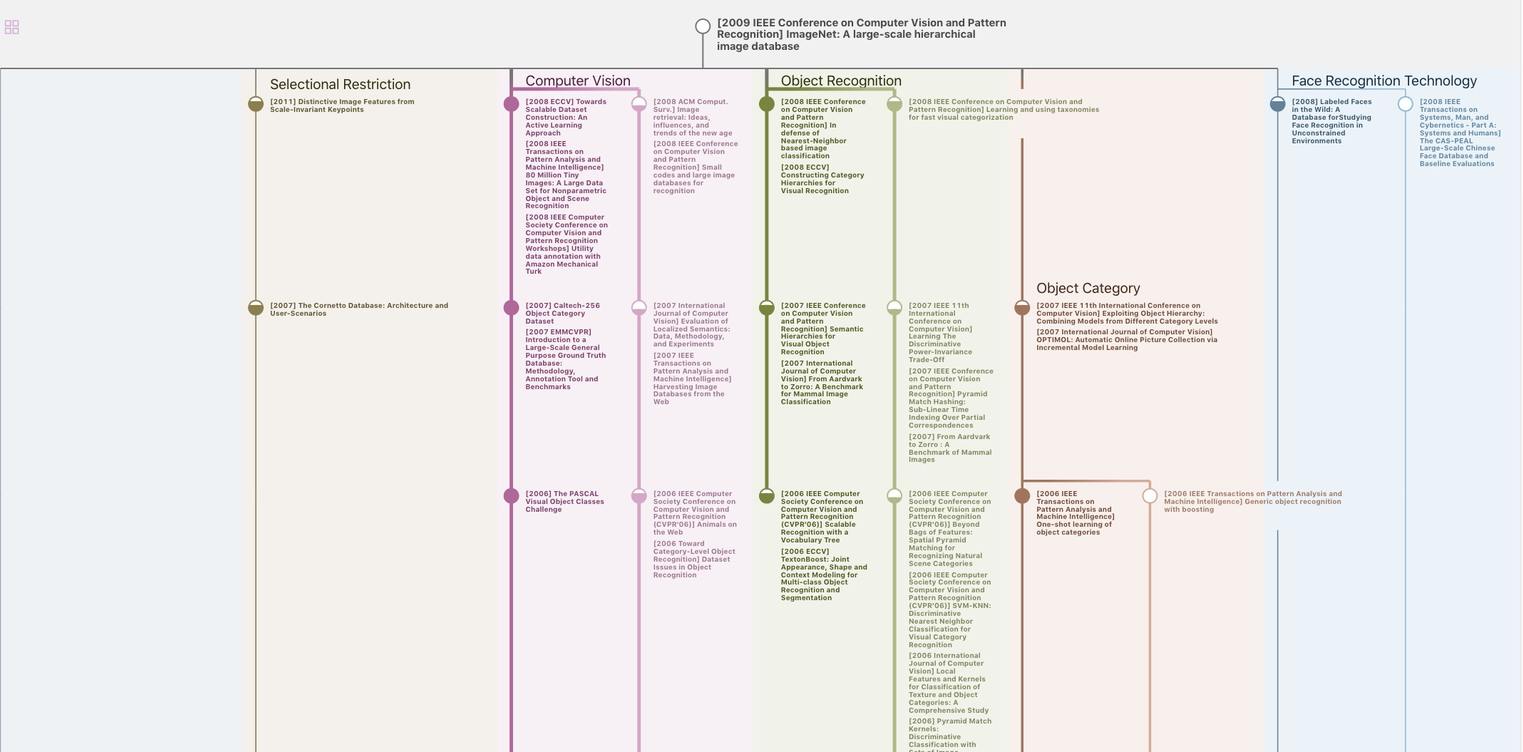
生成溯源树,研究论文发展脉络
Chat Paper
正在生成论文摘要