Forecasting regional-level COVID-19 hospitalisation in England as an ordinal variable using the machine learning method
medRxiv (Cold Spring Harbor Laboratory)(2023)
摘要
Background COVID-19 causes substantial pressure on healthcare, with many healthcare systems now needing to prepare for and mitigate the consequences of surges in demand caused by multiple overlapping waves of infections. Therefore, public health agencies and health system managers also now benefit from short-term forecasts for respiratory infections that allow them to manage services better. However, the availability of easily implemented effective tools for generating precise forecasts at the individual regional level still needs to be improved. Methods We extended prior work on influenza to forecast regional COVID-19 hospitalisations in England for the period from 19th March 2020 to 31st December 2022, treating the number of hospital admissions in each region as an ordinal variable. We further developed the XGBoost model used previously to forecast influenza to enable it to exploit the ordering information in ordinal hospital admission levels. We incorporated different types of data as predictors: epidemiological data including weekly region COVID-19 cases and hospital admissions, weather conditions and mobility data for multiple categories of locations (e.g., parks, workplaces, etc). The impact of different discretisation methods and the number of ordinal levels was also considered. Results We find that the inclusion of weather data consistently increases the accuracy of our forecasts compared with models that rely only on the intrinsic epidemiological data, but only by a small amount. Mobility data brings about a more substantial increase in our forecasts. When both weather and mobility data are used in addition to the epidemiological data, the results are very similar to the model with only epidemiological data and mobility data. Conclusion Accurate ordinal forecasts of COVID-19 hospitalisations can be obtained using XGBoost and mobility data. While uniform ordinal levels show higher apparent accuracy, we recommend N-tile ordinal levels which contain far richer information.
### Competing Interest Statement
The authors have declared no competing interest.
### Funding Statement
The authors acknowledge funding from the MRC Centre for Global Infectious Disease Analysis (MR/R015600/1), jointly funded by the UK Medical Research Council (MRC) and the UK Foreign, Commonwealth & Development Office (FCDO), under the MRC/FCDO Concordat agreement, and also part of the EDCTP2 programme supported by the European Union. S.R. acknowledges the support from Wellcome Trust Investigator Award (UK, 200861/Z/16/Z). KOK acknowledges funding from HMRF (INF-CUHK-1). RL acknowledges funding from Nanjing Medical University Talents Start-up Grants (NMUR20220001).
### Author Declarations
I confirm all relevant ethical guidelines have been followed, and any necessary IRB and/or ethics committee approvals have been obtained.
Yes
I confirm that all necessary patient/participant consent has been obtained and the appropriate institutional forms have been archived, and that any patient/participant/sample identifiers included were not known to anyone (e.g., hospital staff, patients or participants themselves) outside the research group so cannot be used to identify individuals.
Yes
I understand that all clinical trials and any other prospective interventional studies must be registered with an ICMJE-approved registry, such as ClinicalTrials.gov. I confirm that any such study reported in the manuscript has been registered and the trial registration ID is provided (note: if posting a prospective study registered retrospectively, please provide a statement in the trial ID field explaining why the study was not registered in advance).
Yes
I have followed all appropriate research reporting guidelines, such as any relevant EQUATOR Network research reporting checklist(s) and other pertinent material, if applicable.
Yes
The source code and data used to produce the results and analyses presented in this manuscript and supporting figures and tables are available on a Git repository: https://github.com/VVVVivi/COVID\_hosp\_forecasting.git. Supporting figures and tables are provided in the same GitHub repository as extended data.
[https://github.com/VVVVivi/COVID\_hosp\_forecasting.git][1]
[1]: https://github.com/VVVVivi/COVID_hosp_forecasting.git
更多查看译文
关键词
forecasting,regional-level regional-level,machine learning,england
AI 理解论文
溯源树
样例
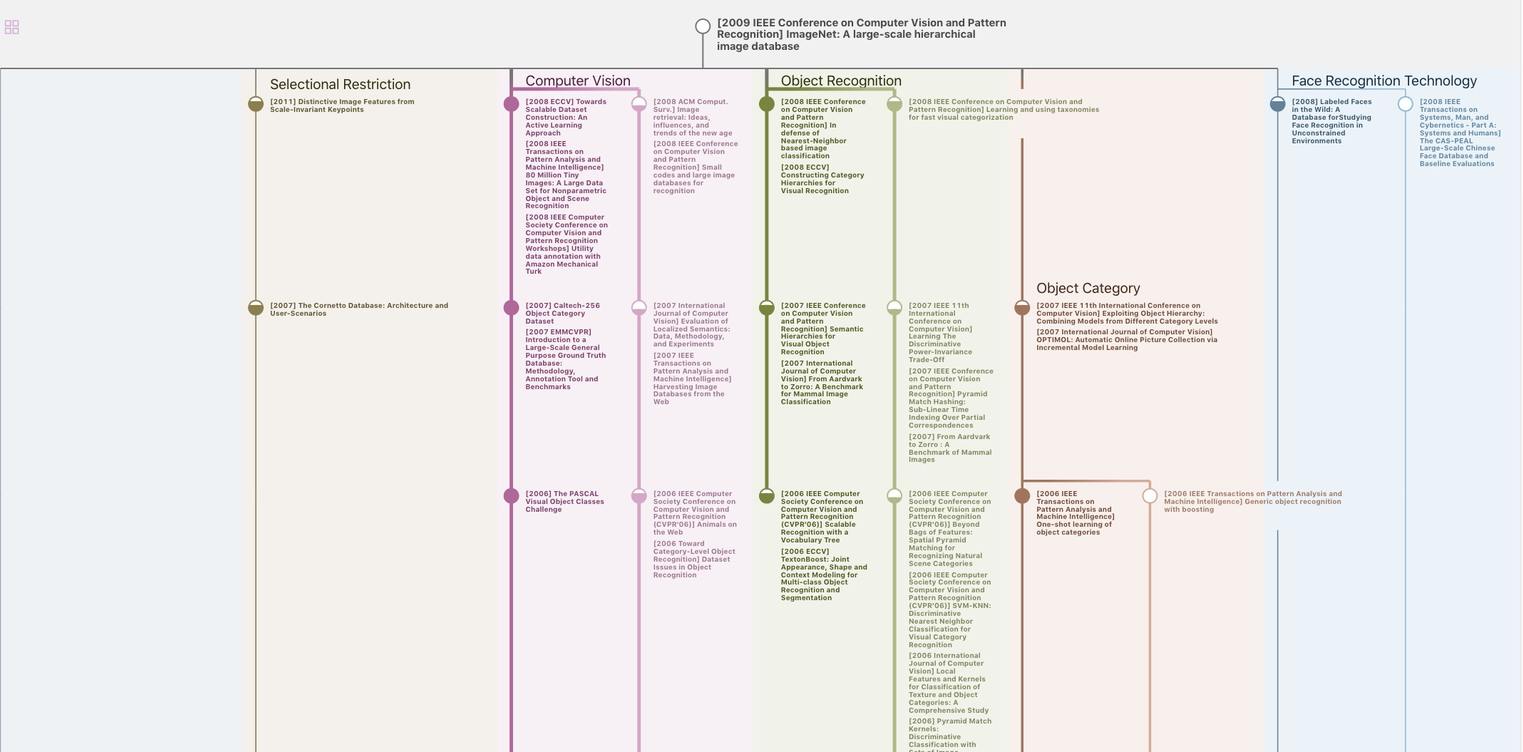
生成溯源树,研究论文发展脉络
Chat Paper
正在生成论文摘要