Multiple imputation assuming missing at random: auxiliary imputation variables that only predict missingness can increase bias due to data missing not at random
medRxiv (Cold Spring Harbor Laboratory)(2023)
摘要
Epidemiological studies often have missing data, which are commonly handled by multiple imputation (MI). MI is valid (given correctly-specified models) if data are missing at random, conditional on the observed data, but not (unless additional information is available) if data are missing not at random (MNAR). In this paper we explore a previously-suggested strategy, namely, including an auxiliary variable predictive of missingness but not the missing data in the imputation model, when data are MNAR. We quantify, algebraically and by simulation, the magnitude of additional bias of the MI estimator, over and above any bias due to data MNAR, from including such an auxiliary variable. We demonstrate that where missingness is caused by the outcome, additional bias can be substantial when the outcome is partially observed. Furthermore, if missingness is caused by the outcome and the exposure, additional bias can be even larger, when either the outcome or exposure is partially observed. When using MI, it is important to identify, through a combination of data exploration and considering plausible casual diagrams and missingness mechanisms, the auxiliary variables most predictive of the missing data (in addition to all variables required for the analysis model and/or to minimise bias due to MNAR).
### Competing Interest Statement
The authors have declared no competing interest.
### Funding Statement
This work is directly funded by the UK Medical Research Council (grant no MR/V020641/1). Elinor Curnow, Jon Heron, Rosie Cornish, and Kate Tilling work in the Medical Research Council Integrative Epidemiology Unit at the University of Bristol which is supported by the UK Medical Research Council (grant no MC\_UU\_00032/02) and the University of Bristol. James Carpenter is also supported by the UK Medical Research Council (grant no MC\_UU\_00004/04). The UK Medical Research Council and the Wellcome Trust (grant no 217065/Z/19/Z), and the University of Bristol currently provide core funding for ALSPAC. Data collection is funded from a wide range of sources: a comprehensive list of grants funding is available on the ALSPAC website (http://www.bristol.ac.uk/alspac/external/documents/grant-acknowledgements.pdf). This publication is the work of the authors, who will serve as guarantors for the contents of this paper.
### Author Declarations
I confirm all relevant ethical guidelines have been followed, and any necessary IRB and/or ethics committee approvals have been obtained.
Yes
The details of the IRB/oversight body that provided approval or exemption for the research described are given below:
Ethical approval was obtained from the Avon Longitudinal Study of Parents and Children Ethics and Law Committee and local research ethics committees. Informed consent for the use of data collected via questionnaires and clinics was obtained from participants following the recommendations of the Avon Longitudinal Study of Parents and Children Ethics and Law Committee at the time.
I confirm that all necessary patient/participant consent has been obtained and the appropriate institutional forms have been archived, and that any patient/participant/sample identifiers included were not known to anyone (e.g., hospital staff, patients or participants themselves) outside the research group so cannot be used to identify individuals.
Yes
I understand that all clinical trials and any other prospective interventional studies must be registered with an ICMJE-approved registry, such as ClinicalTrials.gov. I confirm that any such study reported in the manuscript has been registered and the trial registration ID is provided (note: if posting a prospective study registered retrospectively, please provide a statement in the trial ID field explaining why the study was not registered in advance).
Yes
I have followed all appropriate research reporting guidelines, such as any relevant EQUATOR Network research reporting checklist(s) and other pertinent material, if applicable.
Yes
Stata code to verify algebraic results, and also to generate and analyse the data as per the simulation studies is included in Supplementary Material, Section S6. Stata code to perform the real data analysis is included in Supplementary Material, Section S7. The real data are not publicly available due to privacy restrictions. Requests to access these datasets should be directed to alspac-data@bristol.ac.uk.
更多查看译文
关键词
auxiliary imputation variables,multiple imputation,missingness,bias,data
AI 理解论文
溯源树
样例
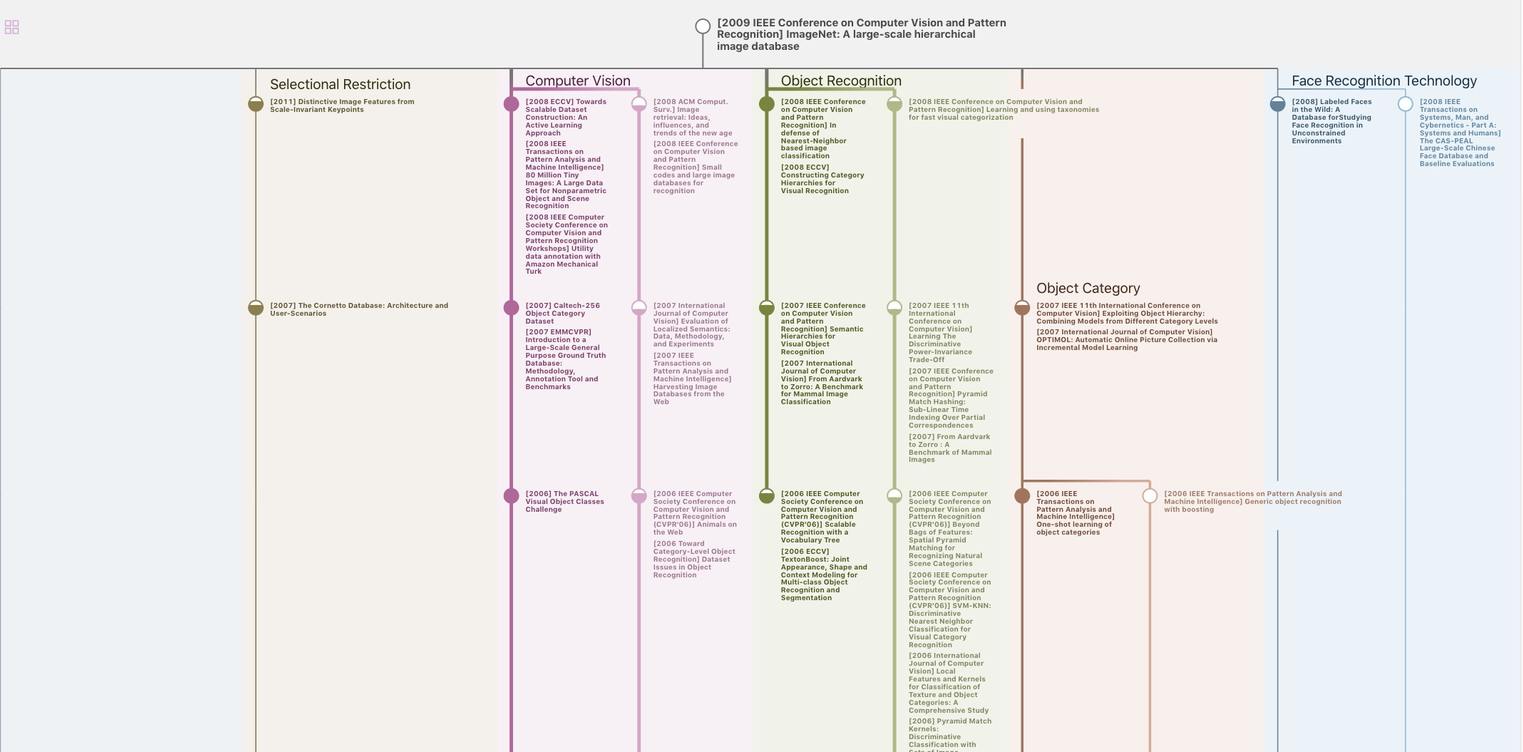
生成溯源树,研究论文发展脉络
Chat Paper
正在生成论文摘要