An improved artificial neural network fit of the ab initio potential energy surface points for HeH+ + H2 and its ensuing rigid rotors quantum dynamics
Artificial Intelligence Chemistry(2023)
摘要
Artificial neural networks (ANN) have been shown for the last several years to be a versatile tool for fitting ab initio potential energy surfaces. We have demonstrated recently how a 60-neuron ANN could successfully fit a four-dimensional ab initio potential energy surface for the rigid rotor HeH+ - rigid rotor H2 system with a root-mean-squared deviation (RMSD) of 35 cm−1. We show in the present study how a (40, 40) neural network with two hidden layers could achieve a better fit with an RMSD of 5 cm−1. Through a follow-up quantum dynamical study of HeH+(j1)-H2(j2) collisions, it is shown that the two fits lead to slightly different rotational excitation and de-excitation cross sections but are comparable to each other in terms of magnitude and dependence on the relative translational energy of the collision partners. When averaged over relative translational energy, the two sets of results lead to rate coefficients that are nearly indistinguishable at higher temperatures thus demonstrating the reliability of the ANN method for fitting ab initio potential energy surfaces. On the other hand, we also find that the de-excitation rate coefficients obtained using the two different ANN fits differ significantly from each other at low temperatures. The consequences of these findings are discussed in our conclusions.
更多查看译文
关键词
Artificial neural network,Potential energy surface,Rotational (de)excitation,Quantum dynamics
AI 理解论文
溯源树
样例
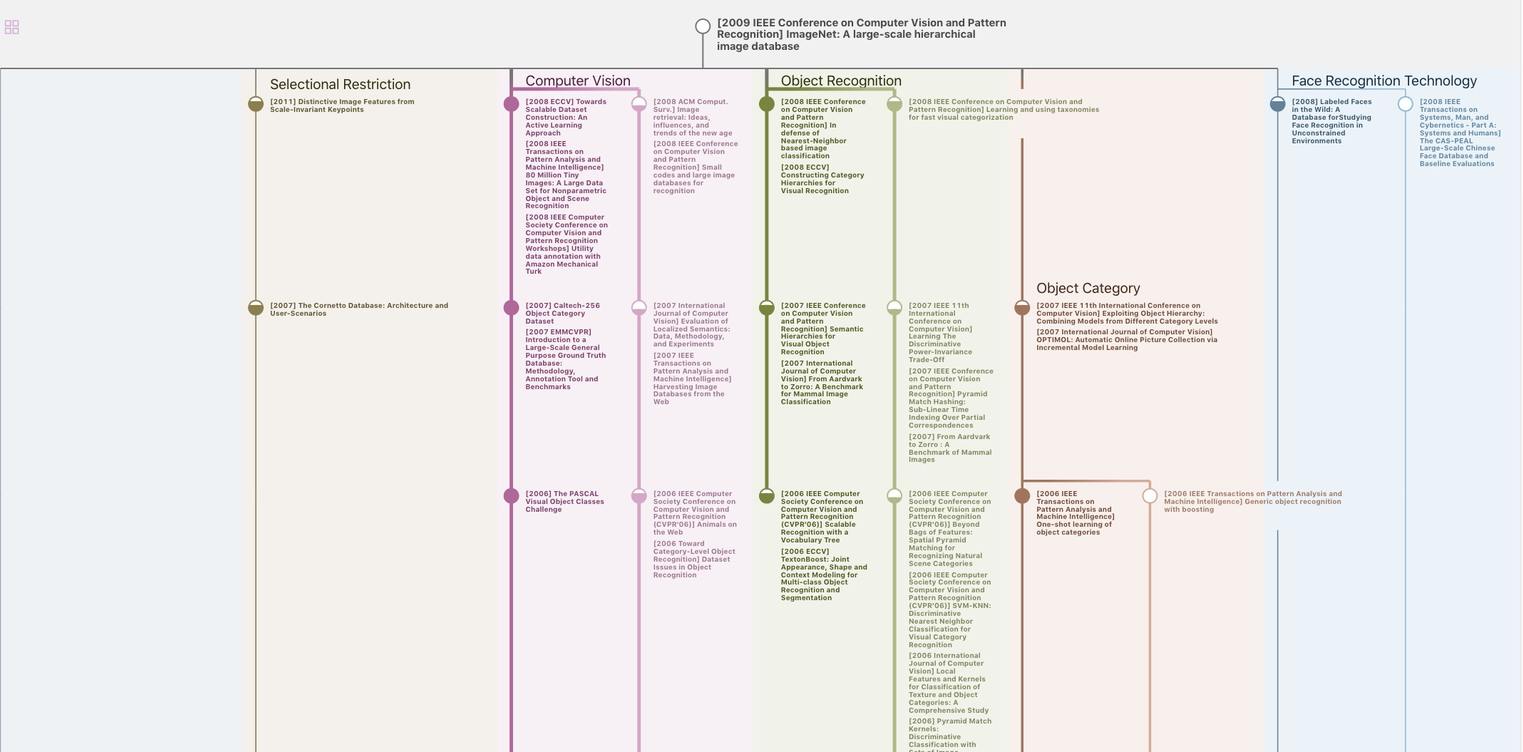
生成溯源树,研究论文发展脉络
Chat Paper
正在生成论文摘要