A Model-Driven Machine Learning Approach for Personalized Kidney Graft Risk Prediction
medrxiv(2023)
摘要
Graft failure after renal transplantation is a multifactorial process. Predicting the risk of graft failure accurately is imperative since such knowledge allows for identifying patients at risk and treatment personalization. In this study, we were interested in predicting the temporal evolution of graft function (expressed as estimated glomerular filtration rate; eGFR) based on pretransplant data and early post-operative graft function. Toward this aim, we developed a tailored approach that combines a dynamic GFR mathematical model and machine learning while taking into account the corresponding parameter uncertainty. A cohort of 892 patients was used to train the algorithm and a cohort of 847 patients for validation. Our analysis indicates that an eGFR threshold exists that allows for classifying high-risk patients. Using minimal inputs, our approach predicted the graft outcome with an accuracy greater than 80% for the first and second years after kidney transplantation and risk predictions were robust over time. ### Competing Interest Statement The authors have declared no competing interest. ### Funding Statement Haralampos Hatzikirou has received funding from the Volkswagenstiftung and the Life? program (96732). Haralampos Hatzikirou has received funding from the Bundes Ministerium fur Bildung und Forschung under the grant agreement No. 031L0237C (MiEDGE project/ERACOSYSMED). Finally, Haralampos Hatzikirou acknowledges the funding of the FSU grant 2021-2023 grant from Khalifa University. Wilfried Gwinner has received funding under the frame of ERACoSysMed-2, the ERA-Net for Systems Medicine in clinical research and medical practice (project ROCKET, JTC2_29). ### Author Declarations I confirm all relevant ethical guidelines have been followed, and any necessary IRB and/or ethics committee approvals have been obtained. Yes The details of the IRB/oversight body that provided approval or exemption for the research described are given below: Data collection and analysis were performed with prior informed consent of the patients and with the approval of the Hannover Medical School Ethics review board (no 2765) that decides on all patient studies, according to national laws and based on the Declaration of Helsinki and regarding transplant patients, on the Declaration of Istanbul. I confirm that all necessary patient/participant consent has been obtained and the appropriate institutional forms have been archived, and that any patient/participant/sample identifiers included were not known to anyone (e.g., hospital staff, patients or participants themselves) outside the research group so cannot be used to identify individuals. Yes I understand that all clinical trials and any other prospective interventional studies must be registered with an ICMJE-approved registry, such as ClinicalTrials.gov. I confirm that any such study reported in the manuscript has been registered and the trial registration ID is provided (note: if posting a prospective study registered retrospectively, please provide a statement in the trial ID field explaining why the study was not registered in advance). Yes I have followed all appropriate research reporting guidelines, such as any relevant EQUATOR Network research reporting checklist(s) and other pertinent material, if applicable. Yes All data produced in the present study are available upon reasonable request to the authors
更多查看译文
AI 理解论文
溯源树
样例
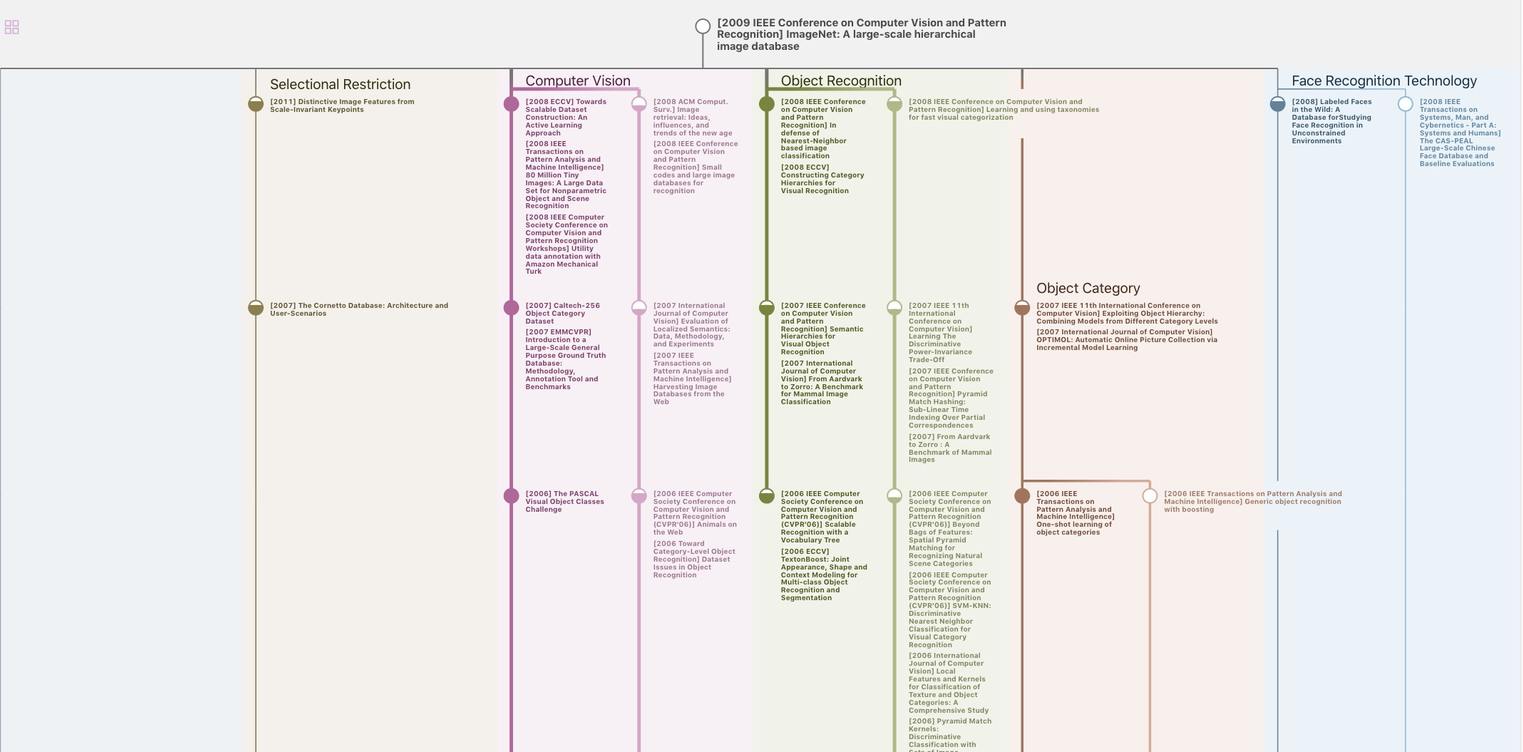
生成溯源树,研究论文发展脉络
Chat Paper
正在生成论文摘要