Enhancing Fairness and Accuracy in Type 2 Diabetes Prediction through Data Resampling
medRxiv (Cold Spring Harbor Laboratory)(2023)
摘要
Machine learning (ML) methodologies have gained significant traction in the realm of healthcare due to their capacity to enhance diagnosis, treatment, and patient outcomes. Nevertheless, mitigating bias within these models is imperative to ensure equitable healthcare regardless of demographic factors such as age, gender, and ethnicity. This study explores the effectiveness of various sampling strategies for balancing imbalanced datasets in the context of improving the accuracy of type 2 diabetes prediction. The investigation leverages multiple ML classifiers and applies them to the inherently imbalanced Behavioral Risk Factor Surveillance System (BRFSS) datasets. Three distinct ML algorithms, namely Logistic Regression, Random Forest, and Multilayer Perceptron, are assessed on both the original and resampled datasets. The study reveals that dataset balancing through undersampling and oversampling techniques significantly enhances the models’ sensitivity and balanced accuracy by at least 52% and 15%. However, it is observed that certain methods such as SMOTE, ADASYN, Tomek Links, Edited Nearest Distance, and Near Miss do not notably improve model sensitivity. Furthermore, this pattern of performance enhancement holds consistent when tested across multiple years of datasets (2021, 2019, 2017, and 2015). The analysis underscores that models trained on raw, imbalanced datasets exhibit subpar sensitivity across various subgroups, particularly among the White population (Sensitivity 0.17). The adoption of subgroup-based resampling techniques effectively ameliorates sensitivity and balanced accuracy by at least 45% and 10% respectively. Notably, the study identifies blood pressure, kidney disease, cholesterol levels, and BMI are the most important indicators of type 2 diabetes. This research underscores the potential of the resampling technique as a promising approach to developing more equitable, balanced, and accurate ML models, especially when addressing different disparities in healthcare outcomes.
### Competing Interest Statement
The authors have declared no competing interest.
### Funding Statement
This study did not receive any funding
### Author Declarations
I confirm all relevant ethical guidelines have been followed, and any necessary IRB and/or ethics committee approvals have been obtained.
Yes
The details of the IRB/oversight body that provided approval or exemption for the research described are given below:
[https://www.cdc.gov/brfss/annual\_data/annual\_data.htm][1]
I confirm that all necessary patient/participant consent has been obtained and the appropriate institutional forms have been archived, and that any patient/participant/sample identifiers included were not known to anyone (e.g., hospital staff, patients or participants themselves) outside the research group so cannot be used to identify individuals.
Yes
I understand that all clinical trials and any other prospective interventional studies must be registered with an ICMJE-approved registry, such as ClinicalTrials.gov. I confirm that any such study reported in the manuscript has been registered and the trial registration ID is provided (note: if posting a prospective study registered retrospectively, please provide a statement in the trial ID field explaining why the study was not registered in advance).
Yes
I have followed all appropriate research reporting guidelines, such as any relevant EQUATOR Network research reporting checklist(s) and other pertinent material, if applicable.
Yes
All data produced are publicly available online at [www.cdc.gov][2]
[https://www.cdc.gov/brfss/annual\_data/annual\_data.htm][1]
[1]: https://www.cdc.gov/brfss/annual_data/annual_data.htm
[2]: http://www.cdc.gov
更多查看译文
关键词
more equitable predictions,fairness,diabetes
AI 理解论文
溯源树
样例
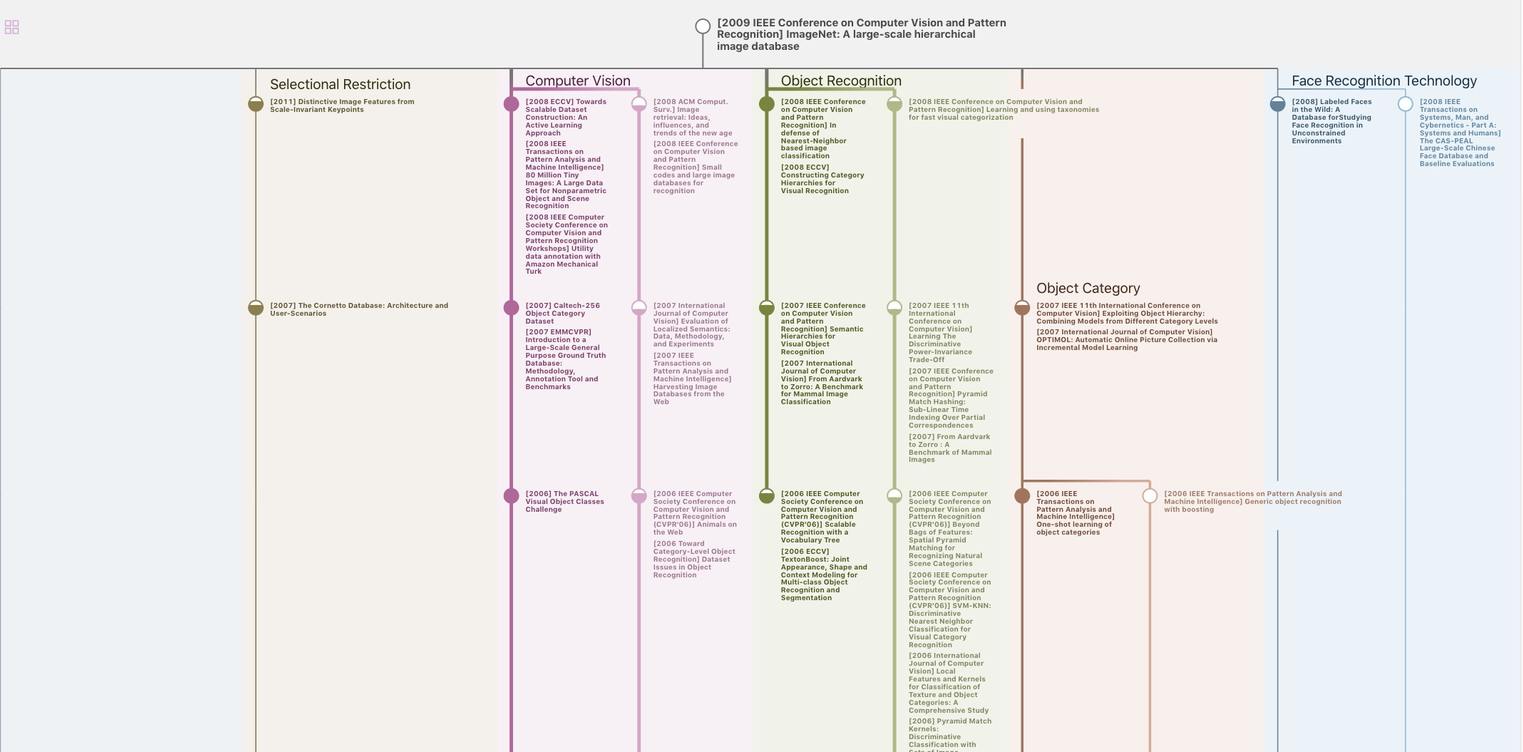
生成溯源树,研究论文发展脉络
Chat Paper
正在生成论文摘要