Understanding structure-processing relationships in metal additive manufacturing via featurization of microstructural images
COMPUTATIONAL MATERIALS SCIENCE(2024)
摘要
Understanding and predicting accurate property-structure-processing relationships for additively manufactured components is important for both forward and inverse design of robust, reliable parts and assemblies. While direct mapping of process parameters to properties is sometimes plausible, it is often rendered difficult due to poor microstructural control. Exploring the direct relationship between processing conditions and microstructural features can thus provide significant physical insights and aid the overall design process. Here, we develop an automated high-throughput framework to simulate an uncertainty-aware additive manufacturing (AM) pro-cess, characterize microstructural images, and extract meaningful features/descriptors. A kinetic Monte Carlo (KMC) based model of the AM process is used to simulate microstructural evolution for a diverse set of exper-imentally relevant processing conditions. We perform a parametric study to explore the relationship between microstructural features and processing conditions. Our results indicate that a many-to-one mapping can exist between processing conditions and typical descriptors; therefore, multiple descriptors are thus necessary to unambiguously represent microstructural images. Our work provides crucial quantitative and qualitative in-formation that would aid in the selection of features for microstructural images. Featurized microstructures could then be utilized to build data-driven models for predictive control of microstructures and thereby properties of additively manufactured components.
更多查看译文
关键词
Additive manufacturing,Featurization,Machine Learning,Inverse design
AI 理解论文
溯源树
样例
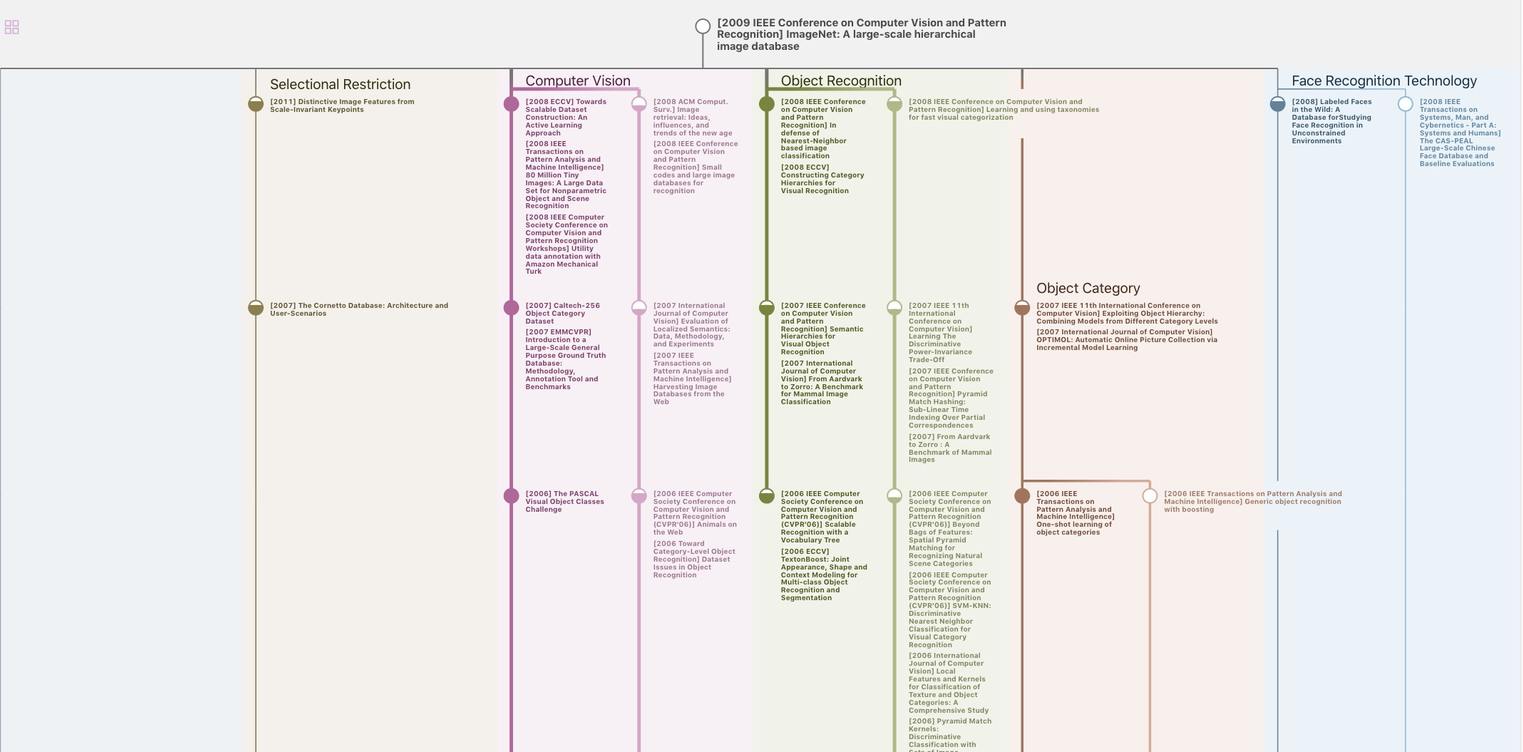
生成溯源树,研究论文发展脉络
Chat Paper
正在生成论文摘要