Knowledge-enhanced reinforcement learning for multi-machine integrated production and maintenance scheduling
Computers & Industrial Engineering(2023)
摘要
Machines deteriorate as they perform production operations, leading to increased production cost rates. When the deterioration reaches a certain level, the machine may break down, which disrupts production and requires costly Corrective Maintenance (CM) to restore operation. Preventive Maintenance (PM) can improve machine health but requires production downtime and expenses. Thus, to balance the increased production cost from degradation against the maintenance cost, it is important to jointly optimize production and maintenance scheduling. This paper aims to address the joint optimization problem for a multi-machine system to achieve the optimal overall business reward under incomplete information and system production demand constraints. We propose a novel method called Knowledge Enhanced Reinforcement Learning (KERL), which adopts a centralized multi-agent actor-critic architecture. KERL enhances the performance of Reinforcement Learning (RL) for multi-machine production and maintenance scheduling by leveraging the prior knowledge of the constraint to determine the production decisions and handle the cooperation among machines in the system. The performance of KERL is evaluated in both deterministic and stochastic case studies and is compared to three baseline methods. Results show that KERL achieves a higher overall business reward than baseline methods and learns to avoid failures in the stochastic environment.
更多查看译文
关键词
Deep reinforcement learning,Knowledge enhanced,Integrated production and maintenance,optimization,Multi-machine system,Stochastic degradation,Condition-dependent cost
AI 理解论文
溯源树
样例
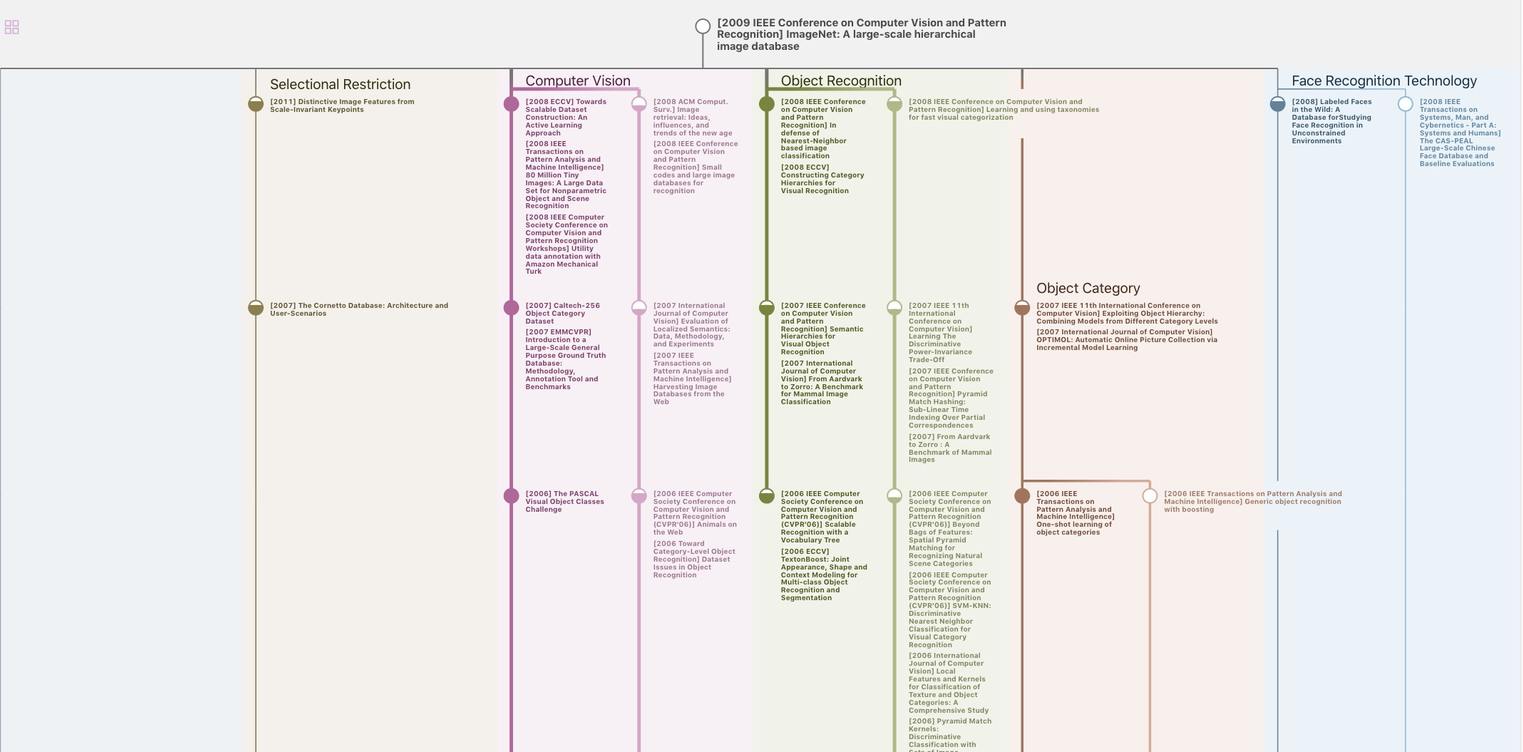
生成溯源树,研究论文发展脉络
Chat Paper
正在生成论文摘要