Comparative study of a newly proposed machine learning classification to detect damage occurrence in structures
ENGINEERING APPLICATIONS OF ARTIFICIAL INTELLIGENCE(2024)
摘要
Over the past two decades, an increasing number of large-scale structures have been built around the world. Constructing these structures has been a time consuming and highly expensive process. Thus, providing a structural health monitoring system to guarantee their proper functionality is important. In recent years, the advancement of technology and artificial intelligence methods based on signal processing and machine learning has attracted the attention of researchers. The challenges currently exist in the field of structural health moni-toring to identify and classify damages to achieve high accuracy in a health-monitoring program. The presence of noise in measurement, various exciting load types, and varying environmental conditions cause difficulty in the practical identification and classification of damage in structures. Recent studies have employed finite element modeling to test the effectiveness of proposed methods for identifying damages in structures. However, detecting damage in real-world structures as mentioned above, presents unique difficulties, and the effectiveness of the proposed methods for damage detection in real-world structures remains uncertain. In order to improve the performance of damage detection methods and increase the accuracy of these methods as much as possible, the most important action is to identify damage sensitive data in the structure. The next challenge is to choose a high performance algorithm for damage identification and classification. One of the advanced algorithms, which has a very high ability to extract the desired features from the measured data, is the XGBoost algorithm. This algorithm has recently attracted the attention of researchers and has been used in different fields. So far, the ability of this algorithm has not been examined in the field of damage detection in order to extract desirable features. This article deals with the identification, classification, and severity of damages in the SMC benchmark bridge, which is an existing megastructure in the real world, as well as the IASC-ASCE benchmark structure, whose responses were taken under applied loads in the laboratory environment. First, using the XGBoost algorithm, the impor-tance of the features extracted from the sensors' data is evaluated, and then the features, which are effective in the damage detection process, are selected. The results of this algorithm indicate that only by selecting 6 features from a large volume of data, the best performance can be achieved and selecting more does not help increase efficiency. In the next step, the Stacking method, which is a hybrid machine learning algorithm for damage classification, is evaluated and compared with some conventional machine learning algorithms that have been used in previous studies. The Stacking method stands out as the top performer with an average accuracy rate of 93.1%, leading to the conclusion that it is the most effective approach. Finally, by applying the presented al-gorithm to the two mentioned structures, its validation is appraised.
更多查看译文
关键词
Structural health monitoring,Damage detection,Hybrid machine learning algorithm,XGBoost,Stacking method
AI 理解论文
溯源树
样例
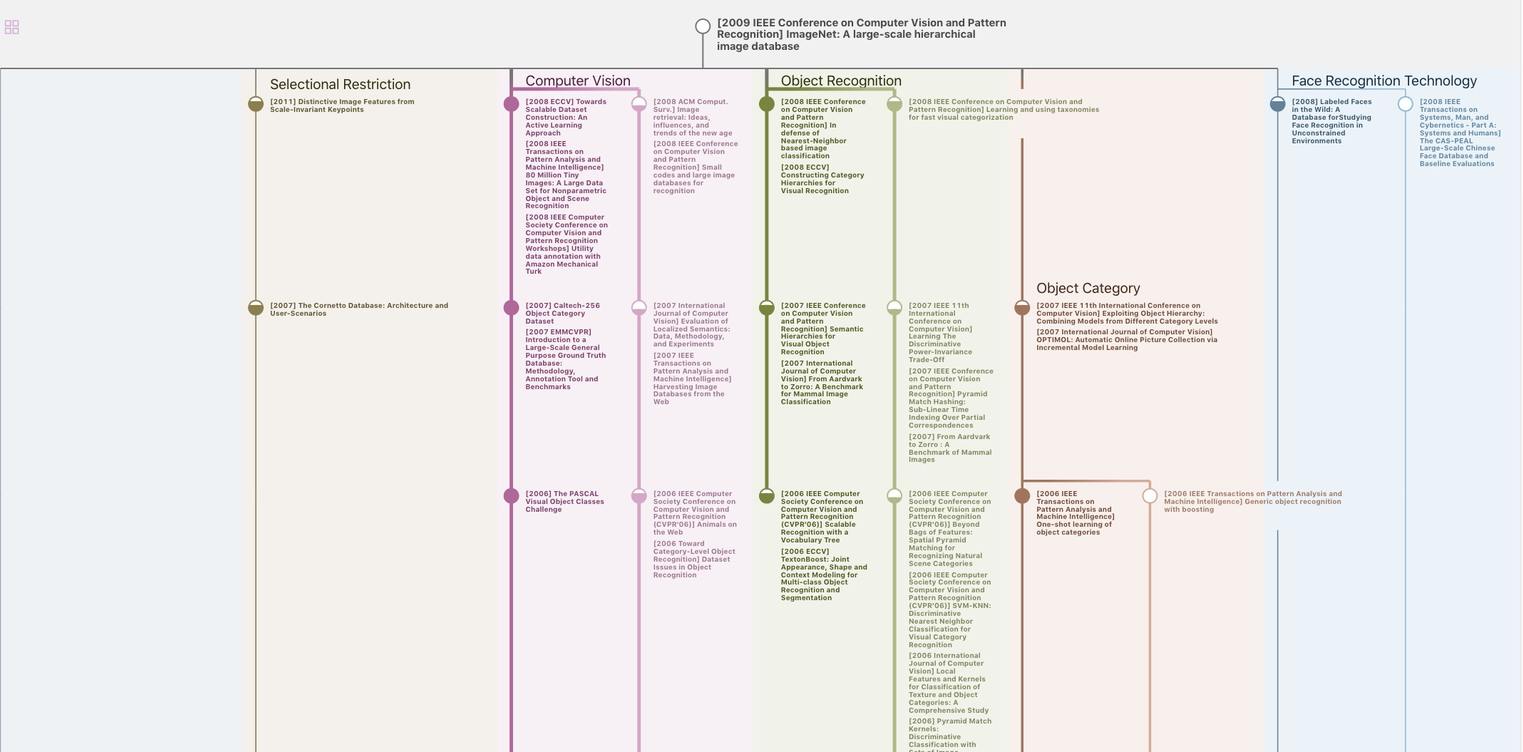
生成溯源树,研究论文发展脉络
Chat Paper
正在生成论文摘要