A lightweight RGB superposition effect adjustment network for low-light image enhancement and denoising
ENGINEERING APPLICATIONS OF ARTIFICIAL INTELLIGENCE(2024)
摘要
In low-light conditions, under-exposure causes certain kinds of image quality degradations, including intensive noise, biased color and degraded contrast. And during the enhancement process, it is also easy to amplify noise or cause overexposure. To overcome these problems, in this paper, an unsupervised learning approach, which is a lightweight RGB superposition effect adjustment network for low light image enhancement (SEA-Net), is proposed. The detail feature module in the proposed network can predict the appropriate saturation and brightness of the color by adjusting the distance among the R, G and B values of the pixels, and then use image multi-level feature fusion to control the overall contrast of the image. The proposed refining extraction operation algorithm uses Hadamard product to amplify the features of image color information, which can effectively avoid color deviation in the enhanced image. The network removes noise by decomposing the image and reconstructing it. To prevent image overexposure, the network effectively suppresses pixel value overflow and avoid image information loss. Because only low-light images are required for training, the proposed loss functions guide network to generate more realistic images with better saturation and contrast. Despite its light weight, this method shows faster running time and more favorable performance than other competitors. In reality, it can be applied to improve the performance of advanced image tasks under low-light conditions, such as nighttime facial recognition.
更多查看译文
关键词
Denoising,Low-light enhancement,Lightweight network,Feature fusion,Unsupervised learning
AI 理解论文
溯源树
样例
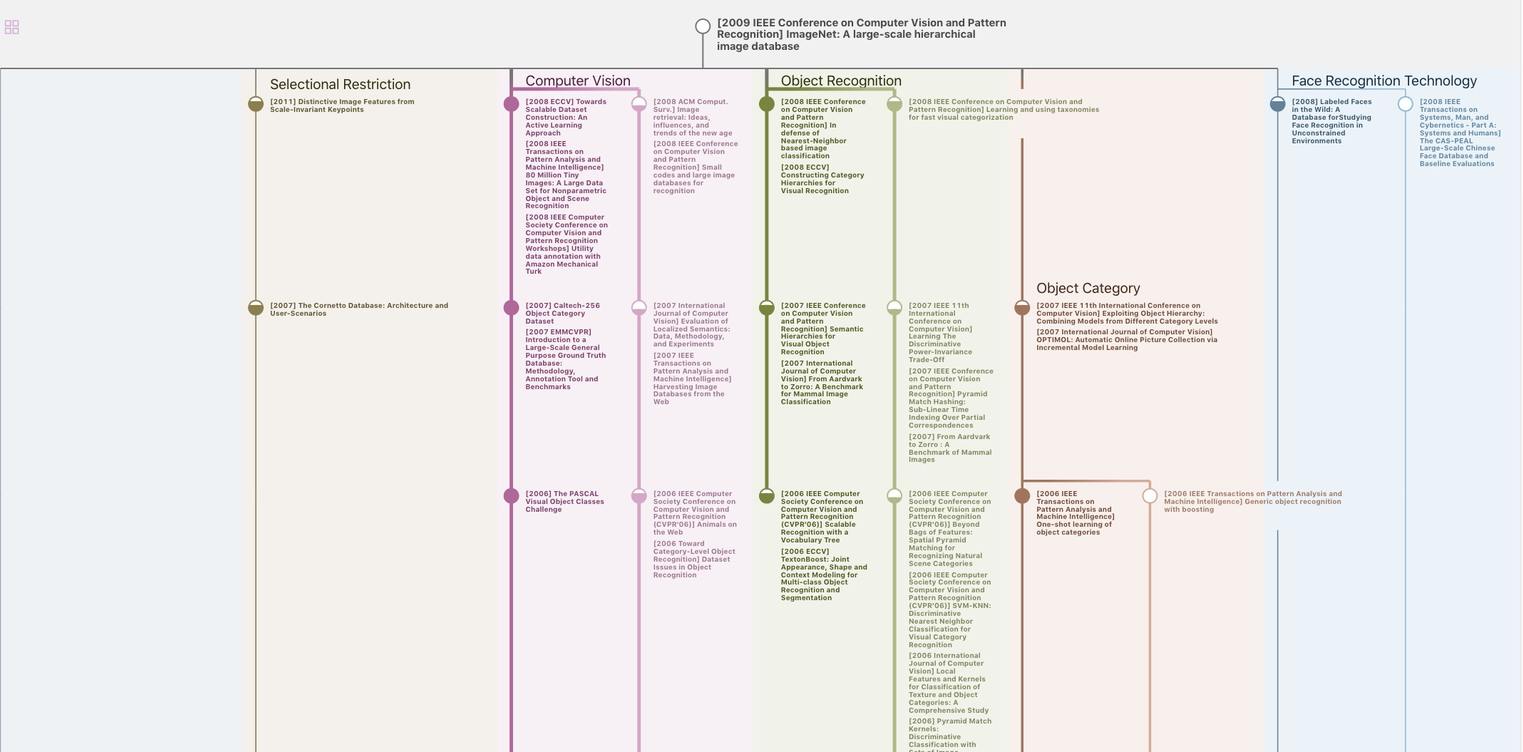
生成溯源树,研究论文发展脉络
Chat Paper
正在生成论文摘要