SKEDS - An external knowledge supported logistic regression approach for document-level sentiment classification
EXPERT SYSTEMS WITH APPLICATIONS(2024)
摘要
Due to the enormous amount of user-generated content being generated on the web, labeling such data is a time-consuming and expensive endeavor. As a result, we have limited annotated data and the vast majority of data are unlabeled. Analysis reveals that extracting (external) knowledge from unlabeled data and integrating it with knowledge extracted from labeled data is a beneficial task for text information processing, in particular text classification. In this paper, we present a hybrid approach for classifying sentiments that employs external knowledge, which is categorized as either general-purpose sentiment knowledge or domain-related knowledge. General-purpose sentiment knowledge is extracted from sentiment lexicons, whereas domain-related knowledge is extracted from unlabeled data from the same or related domains. Similar domains for a given domain are identified based on their similarity score in terms of overlapping features. The proposed approach utilizes both forms of external knowledge and combines them with logistic regression to train an improved classification model. The classification model uses the conventional gradient descent algorithm for optimization, and its convergence analysis indicates that it is convex and converges to the global optimum. The proposed approach is empirically evaluated and compared to three baselines and one state-of-the-art method using standard performance evaluation metrics on a multi-domain sentiment dataset. The experiment results are encouraging, demonstrating that the proposed approach considerably outperforms the baseline approaches and outperforms the state-of-the-art approach by up to 2% in terms of both f-score and accuracy.
更多查看译文
关键词
Text mining,Machine learning,Logistic regression,Sentiment analysis,Knowledge-based system
AI 理解论文
溯源树
样例
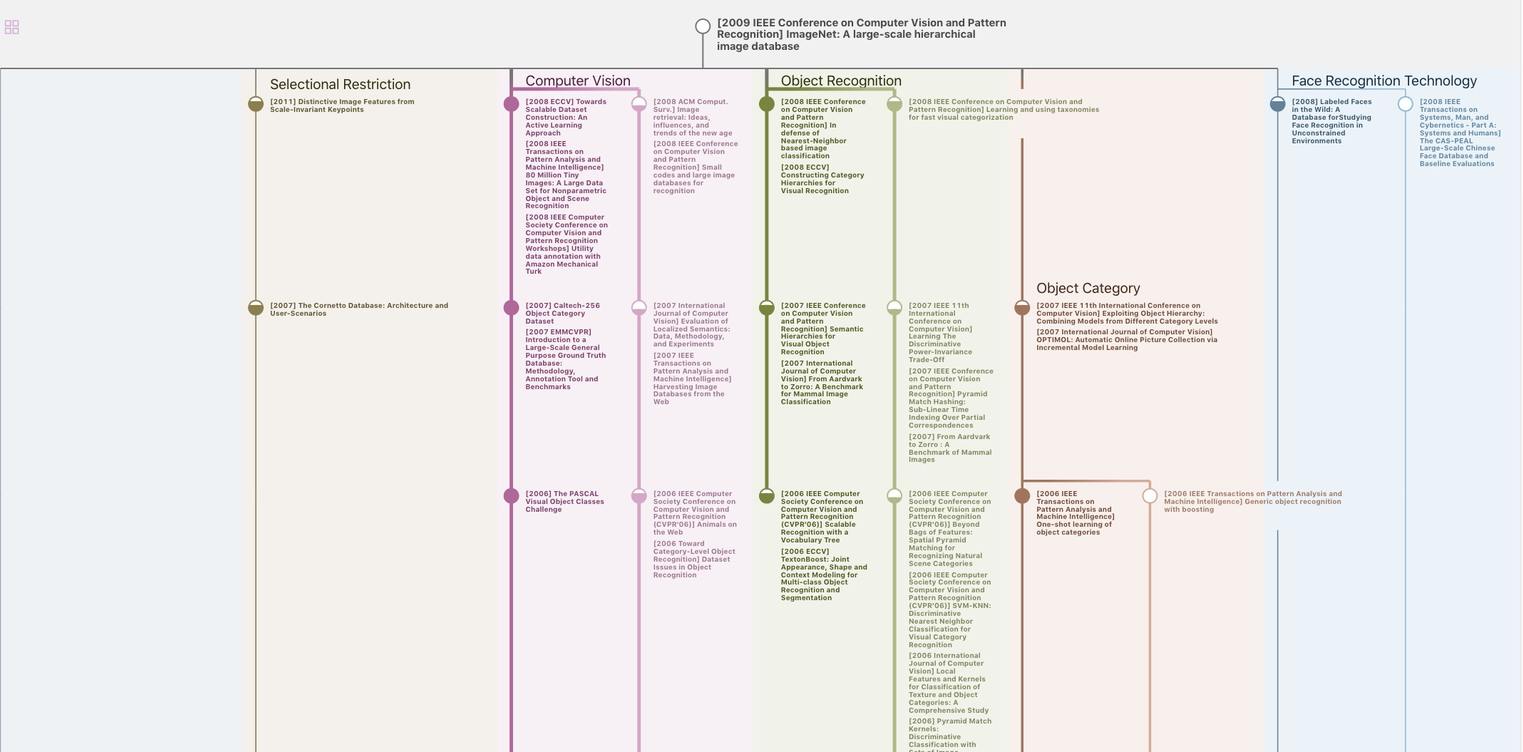
生成溯源树,研究论文发展脉络
Chat Paper
正在生成论文摘要