FDGR-Net: Feature Decouple and Gated Recalibration Network for medical image landmark detection
Expert Systems with Applications(2024)
摘要
Medical image landmark detection can automatically determine the pre-set position coordinates in medical images, so as to further assist disease diagnosis and treatment planning. Using Convolutional Neural Network (CNN) to estimate the heatmap of the landmark has become the mainstream method. However, some landmarks are not obvious and there are local similarities between different landmarks in the medical images. Existing methods usually directly map the image features to all the landmarks, without considering these two challenges. In this paper, we propose a Feature Decouple and Gated Recalibration Network (FDGR-Net) for the medical image landmark detection. The FDGR-Net first establishes independent pathways to decouple all landmark features into single landmark features. In this process, a dynamic multi-kernel convolution is designed as the decouple basic component to improve the feature robustness of the not obvious landmarks. Then, a novel Gated Feature Recalibration Module (GFRM) is proposed, which includes a retention gate and an elimination gate. The retention gate can further strengthen the information of the target landmark and the elimination gate can eliminate the interference of non-target landmarks. The proposed method is validated on one public dataset and two in-house datasets, and its performance is superior to the existing advanced method. The Mean Radial Error (MRE) of the proposed method can reach 3.04, 5.51 and 1.45 in the Knee dataset, Hand dataset and Head dataset, respectively.
更多查看译文
关键词
Medical image,Landmark detection,Gated network,Multi-kernel convolution,Convolutional neural network
AI 理解论文
溯源树
样例
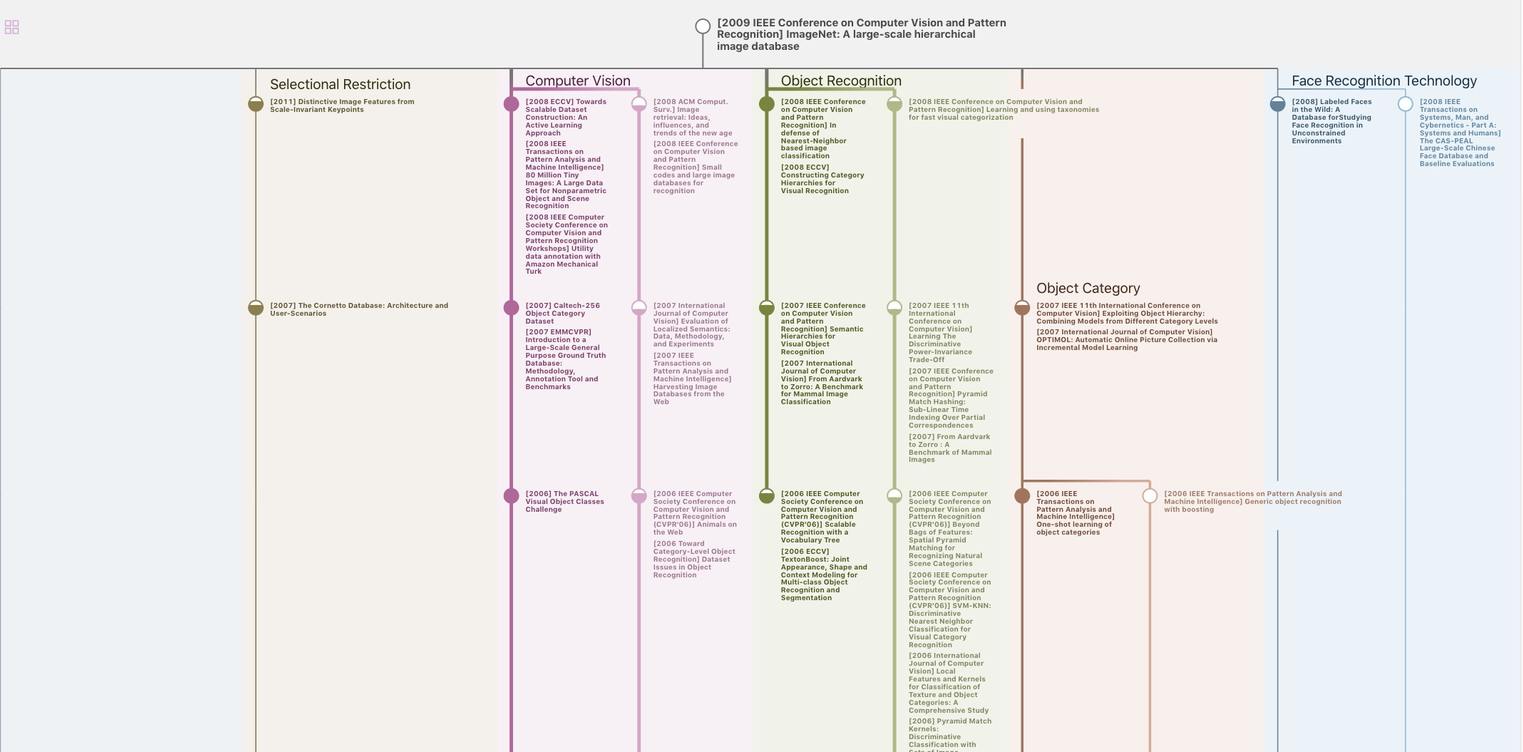
生成溯源树,研究论文发展脉络
Chat Paper
正在生成论文摘要