Fast attribute reduction via inconsistent equivalence classes for large-scale data
International Journal of Approximate Reasoning(2023)
摘要
Feature selection, also known as attribute reduction, plays a crucial role in machine learning and data mining tasks. Rough set theory-based feature selection methods have gained popularity due to their ability to handle imprecise and inconsistent data, ease of implementation, and generation of highly interpretable results. However, these methods still suffer from high computational cost when dealing with large-scale datasets with high dimensions. To overcome this shortcoming, we propose a fast attribute reduction method based on inconsistent equivalence classes. The presented method can accelerate those attribute reduction algorithms whose importance measures used can be computed using only inconsistent equivalence classes. Our proposed method improves attribute reduction efficiency through three key aspects: 1) transforming the original dataset into an equivalently simplified version with fewer samples, 2) accelerating the computation of core attributes, and 3) expediting the forward selection process by removing redundant objects and attributes. Experimental results demonstrate the high computational efficiency of our proposed method.
更多查看译文
关键词
Rough set,Attribute reduction,Granular computing,Hash table,Big data
AI 理解论文
溯源树
样例
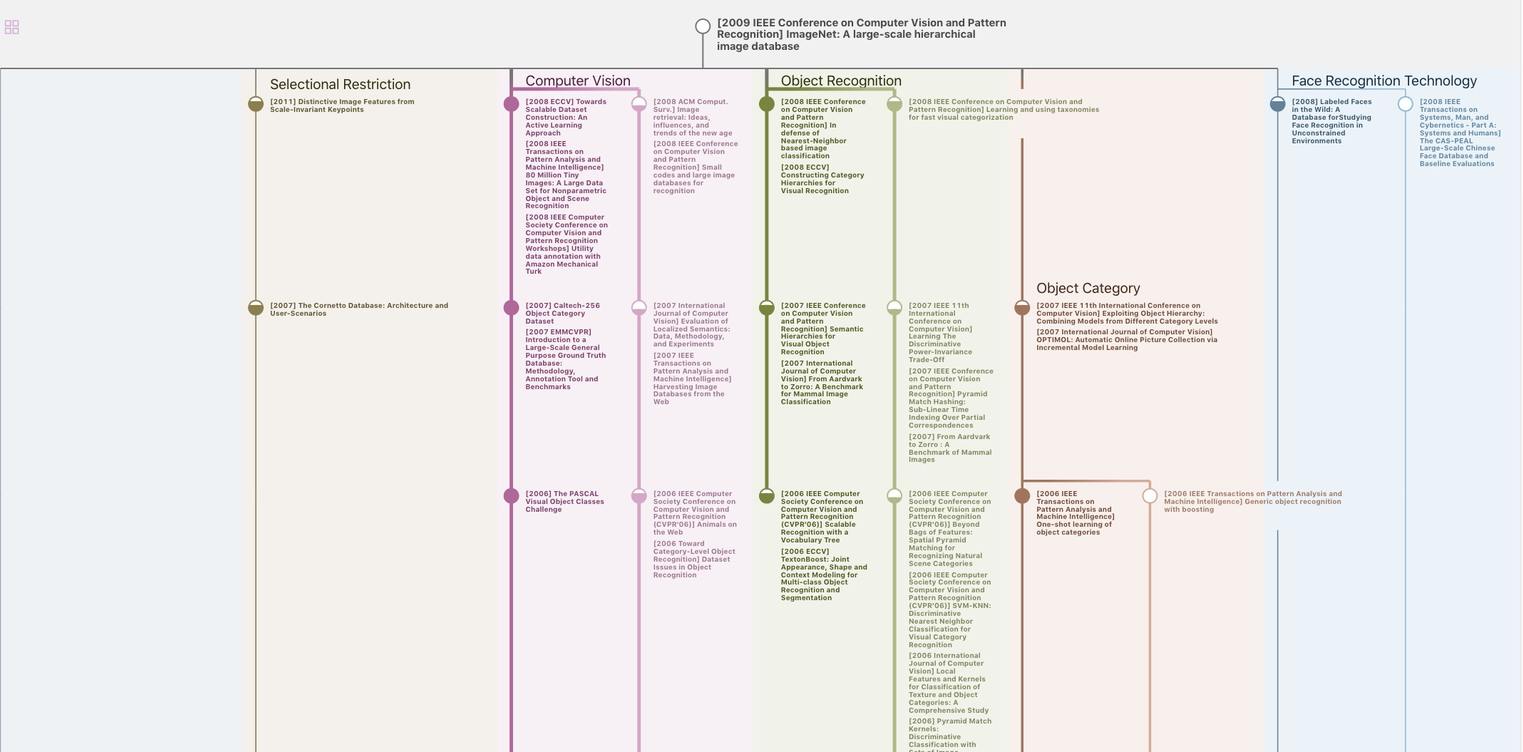
生成溯源树,研究论文发展脉络
Chat Paper
正在生成论文摘要