Optimal combination weight interval-valued carbon price forecasting model based on adaptive decomposition method
Journal of Cleaner Production(2023)
摘要
Accurate carbon price predictions are of utmost significance in curbing greenhouse gas emissions and advancing environmentally sustainable, low-carbon development. As carbon price time series are influenced by time intervals, point value predictions often fall short in capturing the inherent variability within these intervals. Hence, this paper introduces an interval decomposition and integration framework for carbon price prediction based on the interval feedback variational mode decomposition (IFVMD) algorithm and the optimal combination weight prediction model. First, the IFVMD algorithm decomposes the inverted interval-valued sequence through a self-adaptive determination of the decomposition mode number. It then restructures the decomposed modes into interval trends and residuals, scientifically reducing data volatility. Subsequently, within the proposed optimal combination weight model, the Cuckoo Search Optimization algorithm is employed to calculate the weights of subsequences predicted by two models, which merges model features and realizes model complementation. Finally, the results of the prediction are derived by reconstructing the trend and residual interval. The Interval Mean Absolute Percentage Errors (IMAPE) of the model's prediction results are 2.3754%, 3.3773%, and 3.2169%, respectively. These values indicate that the proposed interval combination forecasting technique demonstrates excellent prediction accuracy and stability, and the performance of the proposed model is superior compared to the baseline models. This optimal combination strategy has been proven to be an effective approach for forecasting nonlinear interval-based carbon price time series.
更多查看译文
关键词
carbon price forecasting model,adaptive decomposition method,interval-valued
AI 理解论文
溯源树
样例
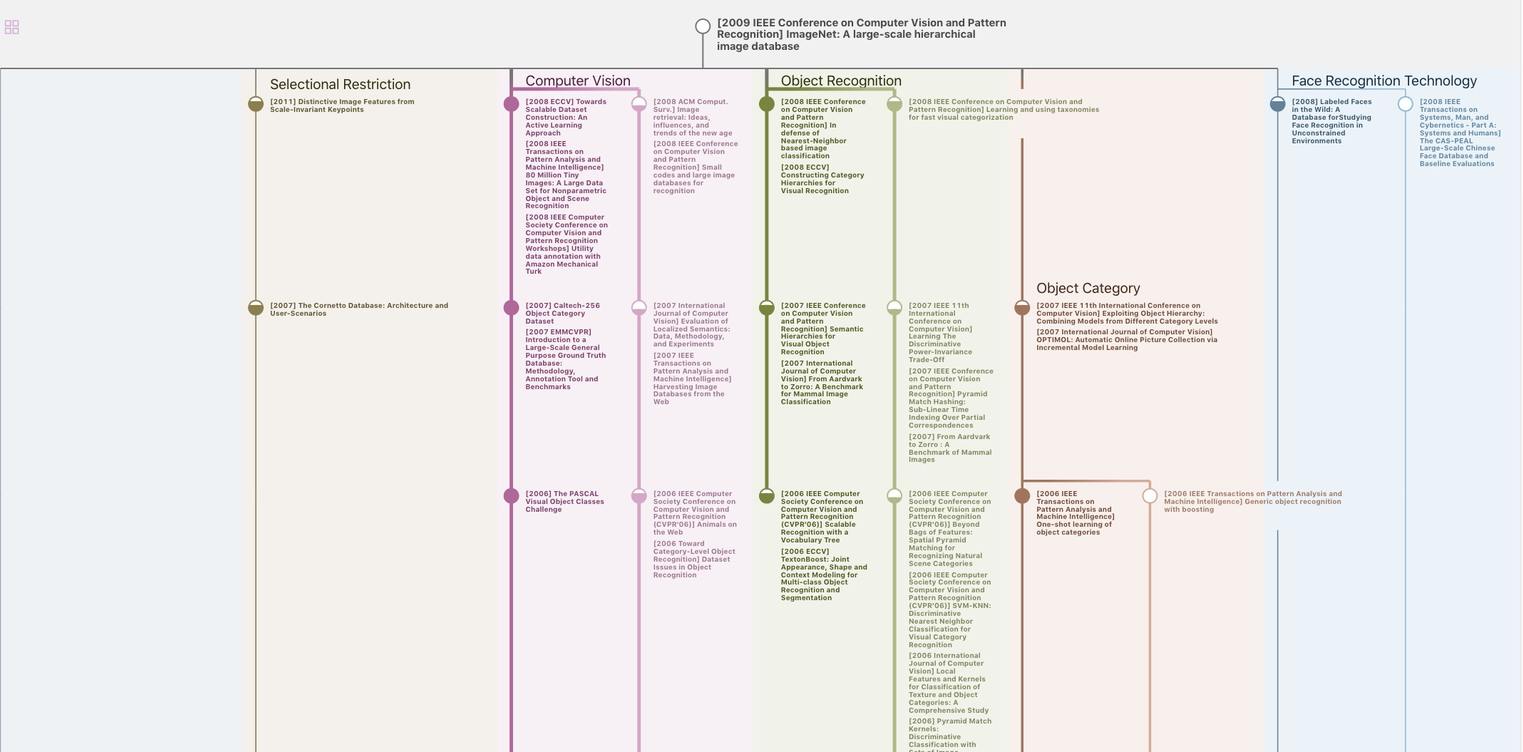
生成溯源树,研究论文发展脉络
Chat Paper
正在生成论文摘要