Hybrid flow-shop scheduling in collaborative manufacturing with a multi-crossover-operator genetic algorithm
Journal of Industrial Information Integration(2023)
摘要
Collaborative manufacturing systems have become a key component of Industry 4.0, supported by Industrial Information Integration Engineering (IIIE) applications. Efficient scheduling and coordination of manufacturing tasks are vital for these systems, directly impacting factory operational efficiency. One of the most prominent optimization problems in collaborative manufacturing is the hybrid flow shop scheduling problem with multiprocessor task (HFSPMT). This study proposes an improved genetic algorithm with multi-crossover-operator, called MCO-GA. MCO-GA introduces a novel crossover operator named SX, which demonstrates superior convergence efficiency compared to classical crossover operators. Furthermore,MCO-GA utilizes a probability selection method to autonomously choose between classical crossover operators and SX during the crossover stage. The effectiveness of MCO-GA is demonstrated through its successful application in solving a scheduling problem in a real-life wood manufacturing factory. Comparing MCO-GA with the state-of-the-art metaheuristic algorithms (HSA, OBL_HSA, and MGLS), it is observed that MCO-GA achieves significantly better average results in over 60% of instances. Additionally, MCO-GA outperforms OBL_HSA and MGLS in terms of computation time. These results highlight the effectiveness and efficiency of MCO-GA in solving the HFSPMT problem and its potential for improving scheduling and coordination in collaborative manufacturing systems.
更多查看译文
关键词
Collaborative manufacturing, HFSP with multiprocessor task, Genetic algorithm, Multiple crossover operator
AI 理解论文
溯源树
样例
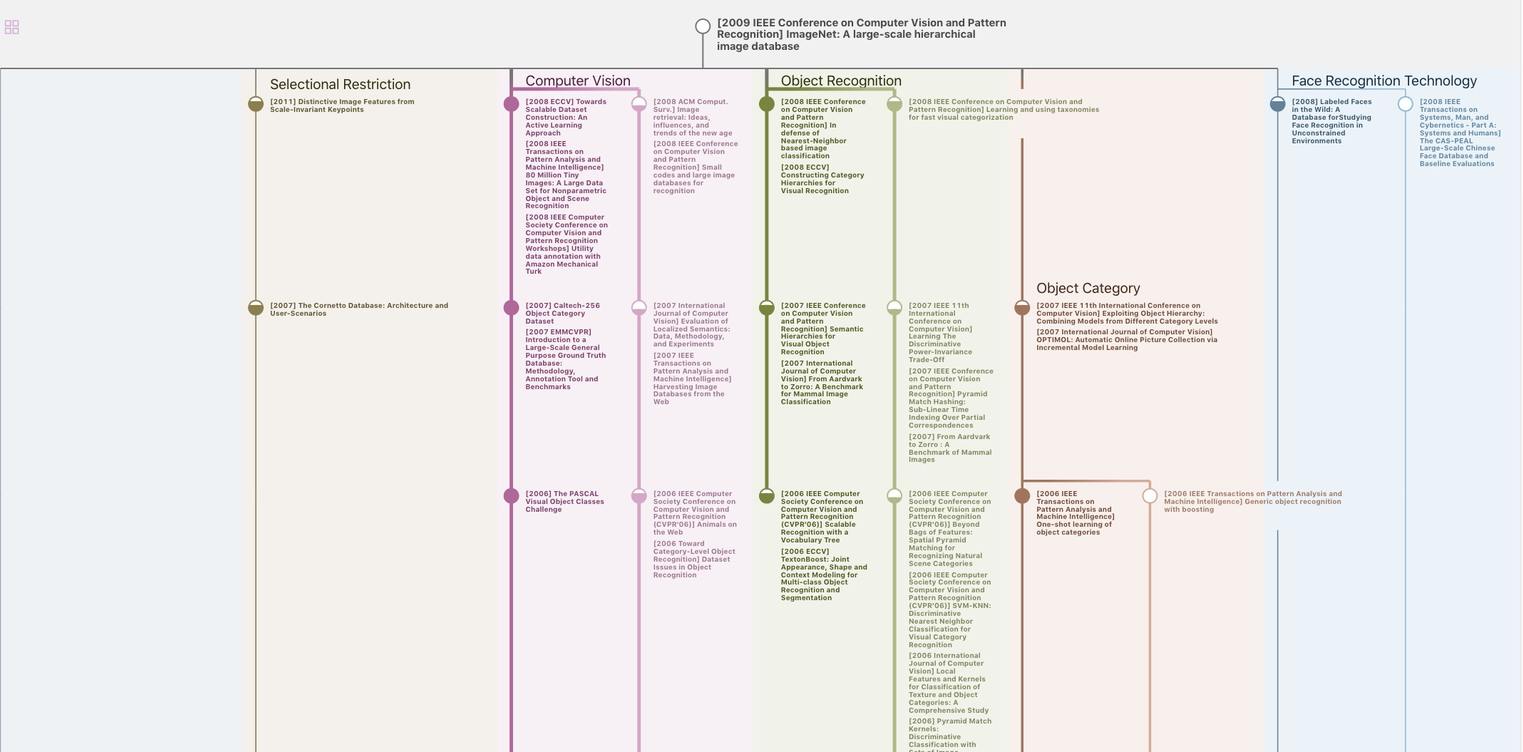
生成溯源树,研究论文发展脉络
Chat Paper
正在生成论文摘要