CE-VNE: Constraint escalation virtual network embedding algorithm assisted by graph convolutional networks
JOURNAL OF NETWORK AND COMPUTER APPLICATIONS(2024)
摘要
With the advent of Industry 5.0, access devices are becoming more and more demanding on the network. For example, in areas such as financial transactions, drone control and telemedicine, the requirements for network response times, i.e. latency, are high. However, in traditional network architectures, the control and data forwarding functions of network devices (e.g. routers, switches, etc.) are tightly coupled, which limits the construction of low-latency networks. The advent of Network Virtualisation (NV) technology can overcome this problem. However, existing Virtual Network Embedding (VNE) algorithms still have some shortcomings. To address the problem that manually extracted network features are sparse and trivial, we use GCN to automatically extract network features, which can efficiently extract spatial topological features of the network. For the problem that the running time of algorithms in large-scale networks is still too long, we accelerate the learning process using a parallel-trained Reinforcement Learning (RL) algorithm, which can shorten the running time of algorithms. For the problem of large fluctuations in the average latency due to changes in the intensity of competition for network resources, we use a constraint escalation strategy to mitigate this phenomenon. Combining the techniques mentioned above, we propose a constraint escalation virtual network embedding algorithm assisted by Graph Convolutional Networks (GCN), called CE-VNE. Extensive simulation results show that the algorithm CE-VNE provides varying degrees of improvement over other algorithms in terms of long-term average gain, latency, acceptance rate, and benefit-cost ratio. Notably, in the later stages of VNE, the latency of our proposed algorithm is on average 26.8% lower than other algorithms.
更多查看译文
关键词
Virtual network embedding,Graph convolutional network,Multi-level feedback queuing,Low latency
AI 理解论文
溯源树
样例
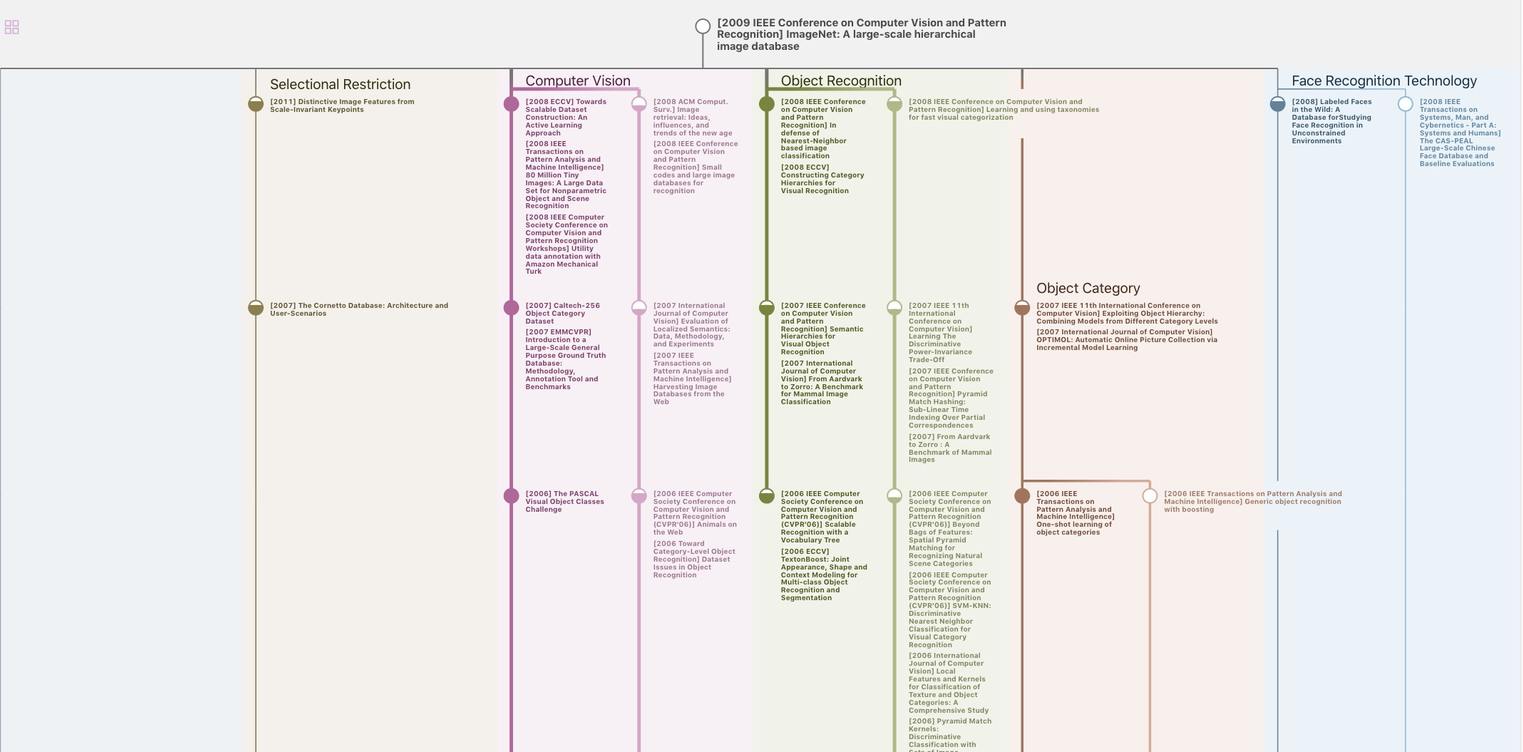
生成溯源树,研究论文发展脉络
Chat Paper
正在生成论文摘要