Building Less-Flawed Metrics: Understanding and Creating Better Measurement and Incentive Systems
Patterns(2023)
摘要
Metrics are useful for measuring systems and motivating behaviors in academia as well as in public policy, medicine, business, and other systems. Unfortunately, naive application of metrics to a system can distort the system and even undermine the original goal. There are two interrelated problems to overcome in building better metrics in academia and elsewhere. The first, specifying evaluable metrics that correspond to the goals, is well recognized but still often ignored. The second, minimizing perverse effects that undermine the metric or that enable people to game the rewards, is less recognized but is critical. This perspective discusses designing metrics, beginning with design considerations and processes; the presentation of specific strategies for mitigating perverse impacts, including secrecy, randomization, diversification, and post hoc specification; and continuing with important desiderata and tradeoffs involved with examples of how they can complement each other or differ. Finally, this perspective presents a comprehensive process integrating these ideas.
更多查看译文
关键词
DSML 3: Development/Pre-production: Data science output has been rolled out/validated across multiple domains/problems
AI 理解论文
溯源树
样例
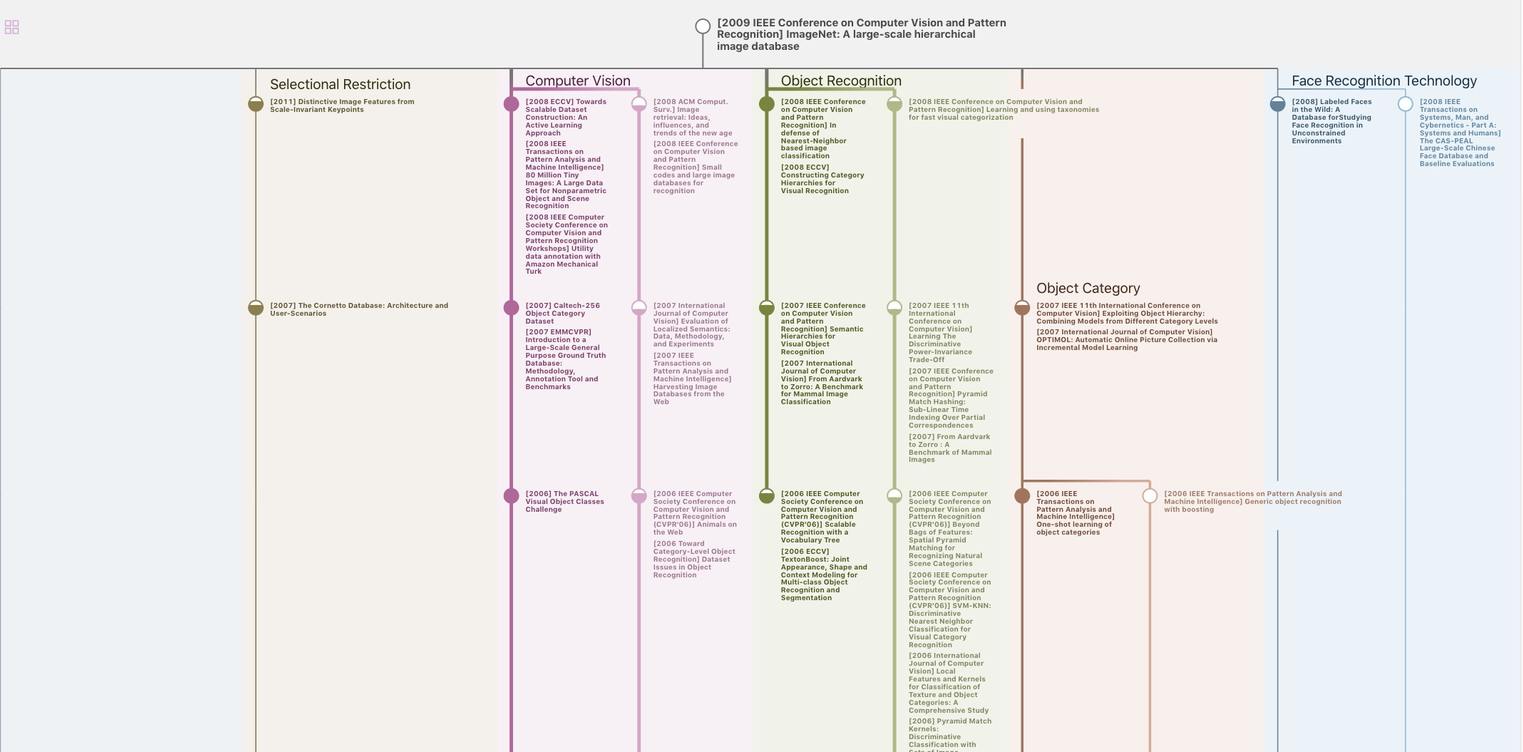
生成溯源树,研究论文发展脉络
Chat Paper
正在生成论文摘要