Physics-informed multi-state temporal frequency network for RUL prediction of rolling bearings
RELIABILITY ENGINEERING & SYSTEM SAFETY(2024)
摘要
Accurate prediction of remaining useful life (RUL) has been a key issue in the field of Prognostic and Health Management (PHM), which aims at predictive maintenance to improve equipment reliability and safety. Aiming at the problem that the existing RUL prediction methods are weak in perceiving long-term features and poor in capturing periodic dependence, which leads to inaccurate and seriously lagging RUL prediction results of rolling bearings, this article proposes a physics-informed bearing RUL prediction approach, namely multi-state temporal frequency network (MSTFN). Firstly, a physics-informed dynamic adaptive inverse discrete Fourier transform (IDFT) frequency domain block is constructed, which maps the spectral information from the known domain to the spectral interval of the unknown domain for extracting the periodic features of the sequence. Secondly, a residual self-attention multi-state gated control unit (RSA-MSGCU) is proposed, which incorporates a novel multi-state hierarchical division mechanism in memory cells to enhance the medium- and long-term feature perception capability. Based on RSA-MSGCU, a trend prediction time domain block is built to extract the trend features of the sequence. Finally, the periodic and trend features are fused to achieve compatibility between the IDFT physical model and the data-driven model and then make the final RUL prediction.
更多查看译文
关键词
Physics-informed,Inverse discrete Fourier transform,Frequency adaptive mechanisms,Hierarchical division mechanism
AI 理解论文
溯源树
样例
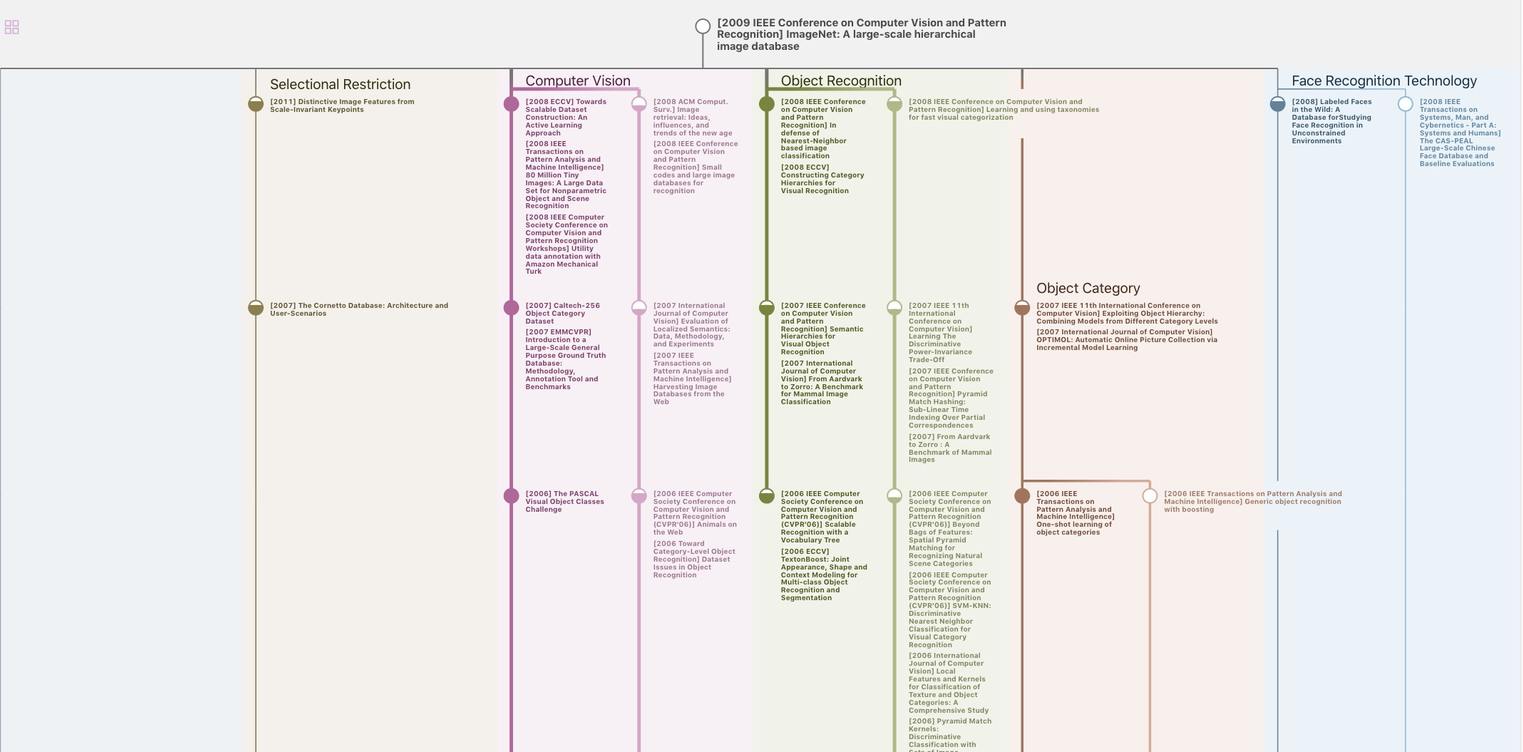
生成溯源树,研究论文发展脉络
Chat Paper
正在生成论文摘要