A comprehensive evaluation of MR-radiomics role in NAR score prediction in locally advanced rectal cancer
The Royal College of Radiologists Open(2023)
摘要
Neoadjuvant Rectal Score (NAR score) is a short-term endpoint developed for clinical trials involving rectal cancer. It can be used as an early evaluation of neoadjuvant treatment response. In this study, we aim to develop a machine learning model based on radiomics features extracted from T2w magnetic resonance images (MRI) obtained pre-operatively to predict for two different binary outcomes, NAR>16 and NAR<8. 112 unique patients who had undergone neoadjuvant chemoradiation (NAC) followed by total mesorectal excision (TME) in National Cancer Centre Singapore were enrolled in this study. Radiomics features were extracted from three different types of segments that were contoured on the T2w MRI before and after NAC. The segments comprised of gross tumor volume (GTV), clinical tumor volume (CTV) and CTV + GTV (total). From which, five different clinico-radiomics models were constructed namely, 1) clinical + GTV, 2) clinical + CTV, 3) clinical + CTV + GTV, 4) clinical + total and 5) clinical + GTV + CTV + total models. The radiomics features extracted from the pre-MRI were also compared with the delta-radiomics approach in this study. This gives a total of ten clinico-radiomics model for each outcome. A nested 10-fold cross validation approach with the AUC metric were used to determine the optimal model. Calibration curves and feature importance were also calculated for the best performing clinico-radiomics models for each outcome and approach. The best NAR<8 pre-MRI and delta clinico-radiomics model models improve the AUC of the clinical model from 0.827 ± 0.206 to 0.868 ± 0.142 and 0.878 ± 0.111 respectively. Both increases are statistically significant (P = 0.032 and P = 0.022). The best NAR>16 pre-MRI and delta clinico-radiomics model models improve the AUC of the clinical model from 0.622 ± 0.146 to 0.749 ± 0.124 and 0.687 ± 0.150 respectively. Only the NAR>16 pre-MRI clinio-radiomics showed a statistically significant increase in AUC (P = 0.041). Out of the four models, only the two NAR>16 clinico-radiomics model are well calibrated. The results also show that the GTV and CTV features are the most important features for NAR<8 model, while the CTV + GTV features are the main driver of NAR>16 pre-MRI clinico-radiomics model. We have carried out for the first time a comprehensive evaluation of MR radiomics models by considering radiomics feature extracted from various segments, pre-MRI versus delta-radiomics and five machine learning algorithms. Our results show that MR radiomics models can value-add to clinical parameters in predicting the NAR score, and it is worthwhile to consider several permutations of the clinico-radiomics models to arrive at the best performing model.
更多查看译文
关键词
advanced rectal cancer,nar score prediction,rectal cancer,mr-radiomics
AI 理解论文
溯源树
样例
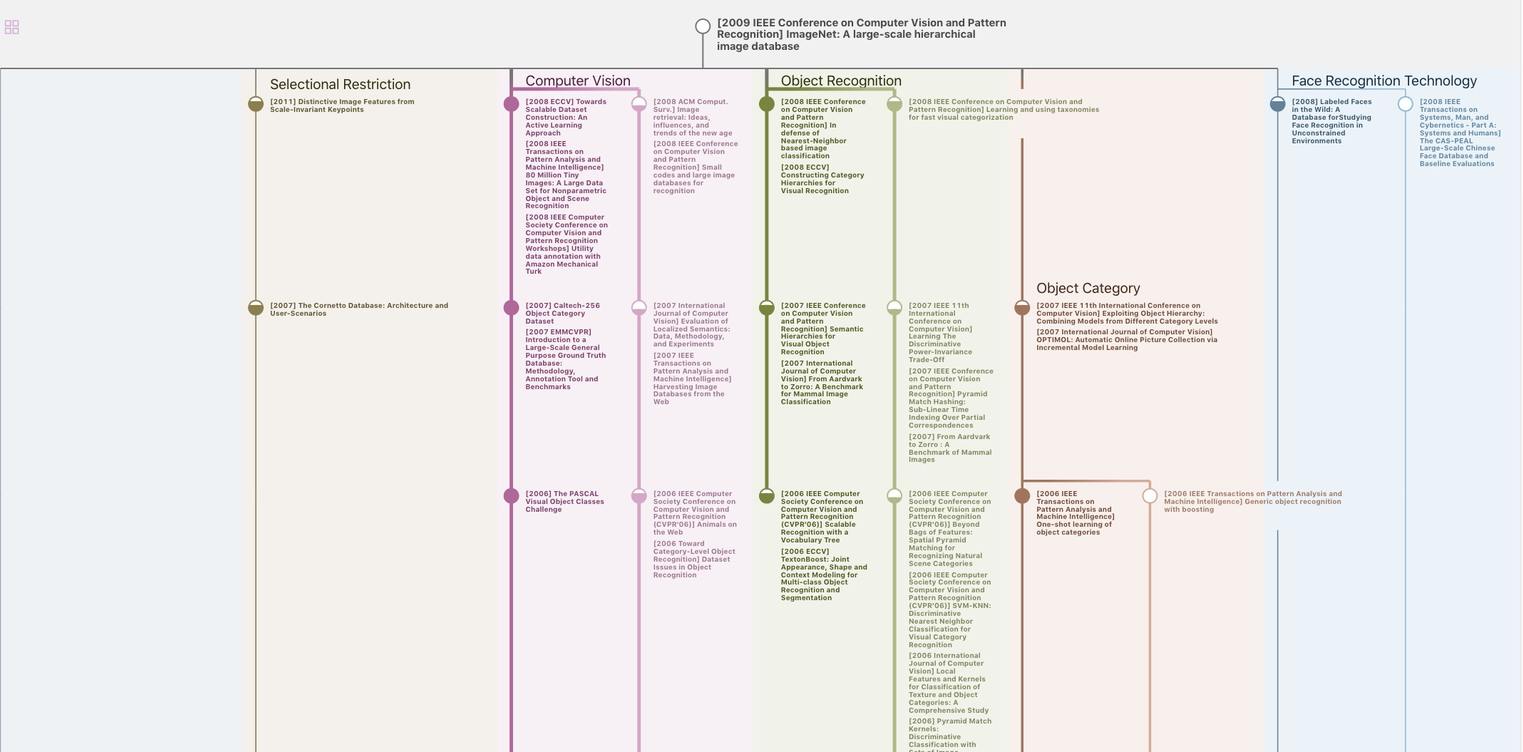
生成溯源树,研究论文发展脉络
Chat Paper
正在生成论文摘要