Enhancing Energy Efficiency and Reliability in Floating LNG Operation: A Hydrofluoroolefin-Based SMR Cycle with Thermo-Economic Assessment and Uncertainty Analysis
Thermal Science and Engineering Progress(2023)
摘要
Natural gas liquefaction is the most energy-intensive technique in floating liquefied natural gas (FLNG) operations. Hence, a hydrofluoroolefin-based single mixed refrigerant (SMR) technology was proposed to achieve energy-efficient and eco-friendly operations. The study was perfomed to enhance the SMR process by substituting expansion valves with hydraulic turbines to improve process reversibility and enhance energy efficiency. The proposed process was optimized using a sine-cosine concept-based algorithm that tuned the design variables which yielded an overall compression energy consumption of 0.2501 (kWh/kg-LNG), resulting in a 21.43% saving as compared to the conventionally used mixed refrigerant-based process. This resulted in a reduction of about 38.81% in overall exergy destruction. The economic analysis results draw curtains that the process saves about 26.02% of total annualized cost as compared to conventional study. Owing to the appropriate mixed refrigerant component mixture ratio and process design improvement. To determine the process reliability under uncertain conditions the optimal compression power and minimum approach temperature were determined. Further, uncertainty analysis reveals that the methane and butane refrigerant flow rates along with evaporation, and condensation pressure, were among the most influential parameters that influence the process most that may affect the whole operation in uncertain process inlet conditions. In summary, our approach presents immense promise for achieving energy efficiency, environmental responsibility, and economic viability in FLNG operations, offering a robust solution for the challenges of offshore plants.
更多查看译文
关键词
Natural gas liquefaction,Hydrofluoroolefin,SMR process,Exergy destruction,Economic analysis,Sensitivity analysis
AI 理解论文
溯源树
样例
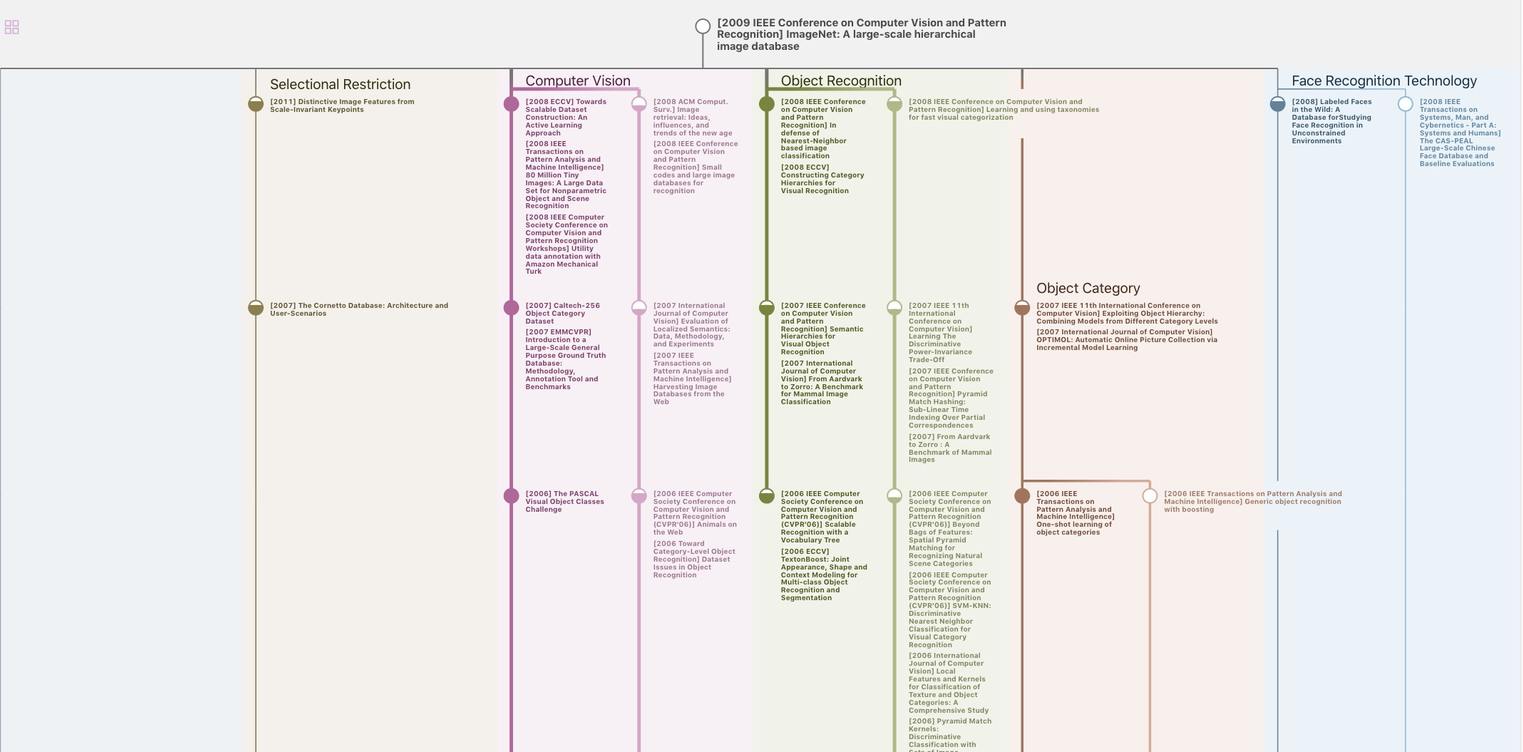
生成溯源树,研究论文发展脉络
Chat Paper
正在生成论文摘要