SISG-Net: Simultaneous instance segmentation and grasp detection for robot grasp in clutter
ADVANCED ENGINEERING INFORMATICS(2023)
摘要
Robots have always found it challenging to grasp in cluttered scenes because of the complex background in-formation and changing operating environment. Therefore, in order to enable robots to perform multi-object grasping tasks in a wider range of application scenarios, such as object sorting on industrial production lines and object manipulation by home service robots, we innovatively integrated segmentation and grasp detection into the same framework, and designed a simultaneous instance segmentation and grasp detection network (SISG-Net). By using the network, robots can better interact with complex environments and perform grasp tasks. In order to solve the problem of insufficient fusion of the existing RGBD fusion strategy in the robot field, we propose a lightweight RGB-D fusion module called SMCF to make modal fusion more efficient. In order to solve the problem of inaccurate perception of small objects in different scenes, we propose the FFASP module. Finally, we use the AFF module to adaptively fuse multi-scale features. Segmentation can remove noise information from the background, enabling the robot to grasp in different backgrounds with robustness. Using the segmentation result, we refine grasp detection and find the best grasp pose for robot grasping in complex scenes. Our grasp detection model performs similarly to state-of-the-art grasp detection algorithms on the Cornell Dataset. Our model achieves state-of-the-art performance on the OCID Dataset. We show that the method is stable and robust in real-world grasping experiments. The code and video of our experiment used in this paper can be found at: https://github.com/meiguiz/SISG-Net.
更多查看译文
关键词
Robot vision,Grasp detection,Instance segmentation,Artificial intelligence
AI 理解论文
溯源树
样例
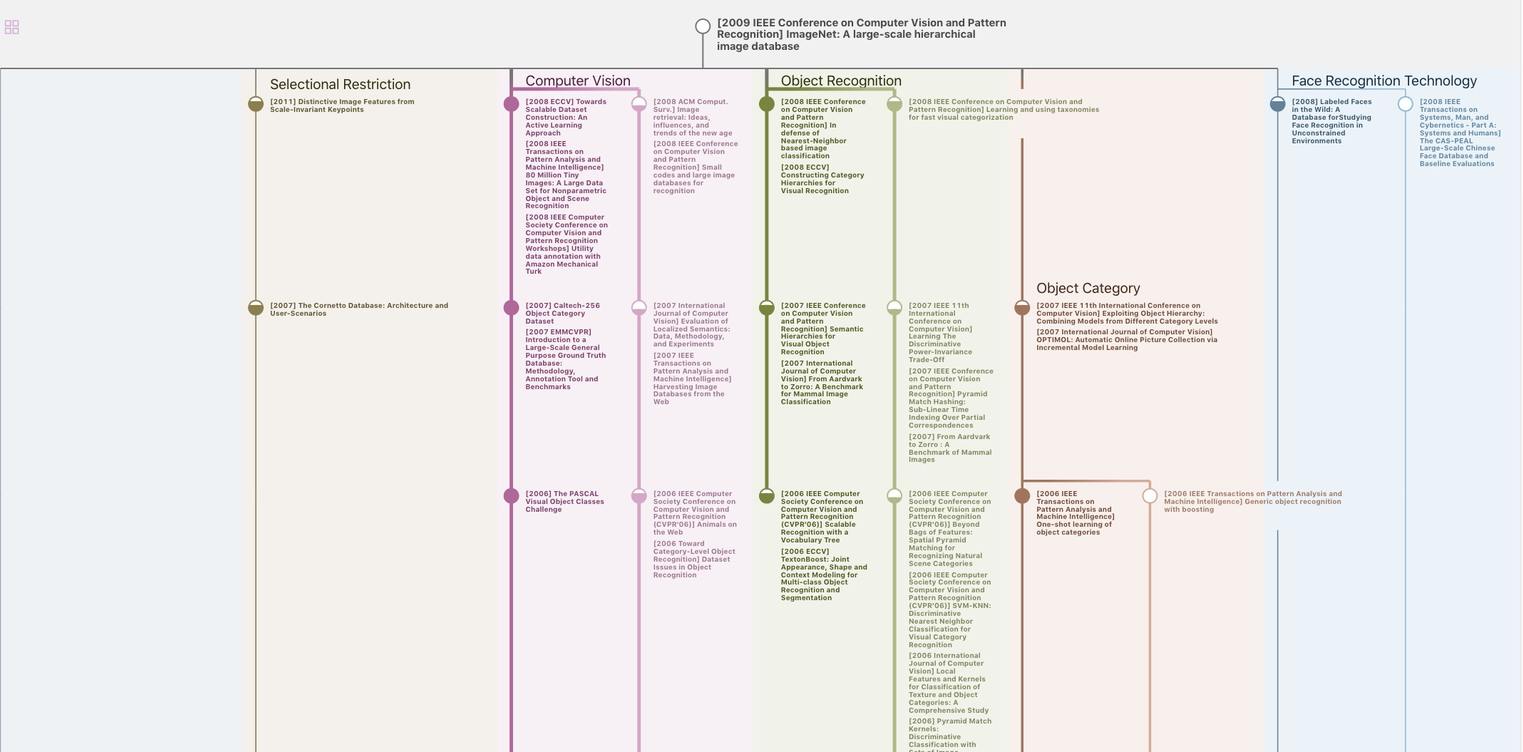
生成溯源树,研究论文发展脉络
Chat Paper
正在生成论文摘要