Predicting protein and pathway associations for understudied dark kinases using pattern-constrained knowledge graph embedding
PeerJ(2023)
摘要
The 534 protein kinases encoded in the human genome constitute a large druggable class of proteins that include both well-studied and understudied "dark"members. Accurate prediction of dark kinase functions is a major bioinformatics challenge. Here, we employ a graph mining approach that uses the evolutionary and functional context encoded in knowledge graphs (KGs) to predict protein and pathway associations for understudied kinases. We propose a new scalable graph embedding approach, RegPattern2Vec, which employs regular pattern constrained random walks to sample diverse aspects of node context within a KG flexibly. RegPattern2Vec learns functional representations of kinases, interacting partners, post-translational modifications, pathways, cellular localization, and chemical interactions from a kinase-centric KG that integrates and conceptualizes data from curated heterogeneous data resources. By contextualizing information relevant to prediction, RegPattern2Vec improves accuracy and efficiency in comparison to other random walk-based graph embedding approaches. We show that the predictions produced by our model overlap with pathway enrichment data produced using experimentally validated Protein-Protein Interaction (PPI) data from both publicly available databases and experimental datasets not used in training. Our model also has the advantage of using the collected random walks as biological context to interpret the predicted protein-pathway associations. We provide high-confidence pathway predictions for 34 dark kinases and present three case studies in which analysis of meta-paths associated with the prediction enables biological interpretation. Overall, RegPattern2Vec efficiently samples multiple node types for link prediction on biological knowledge graphs and the predicted associations between understudied kinases, pseudokinases, and known pathways serve as a conceptual starting point for hypothesis generation and testing.
更多查看译文
关键词
understudied dark kinases,knowledge graph,pathway associations,pattern-constrained
AI 理解论文
溯源树
样例
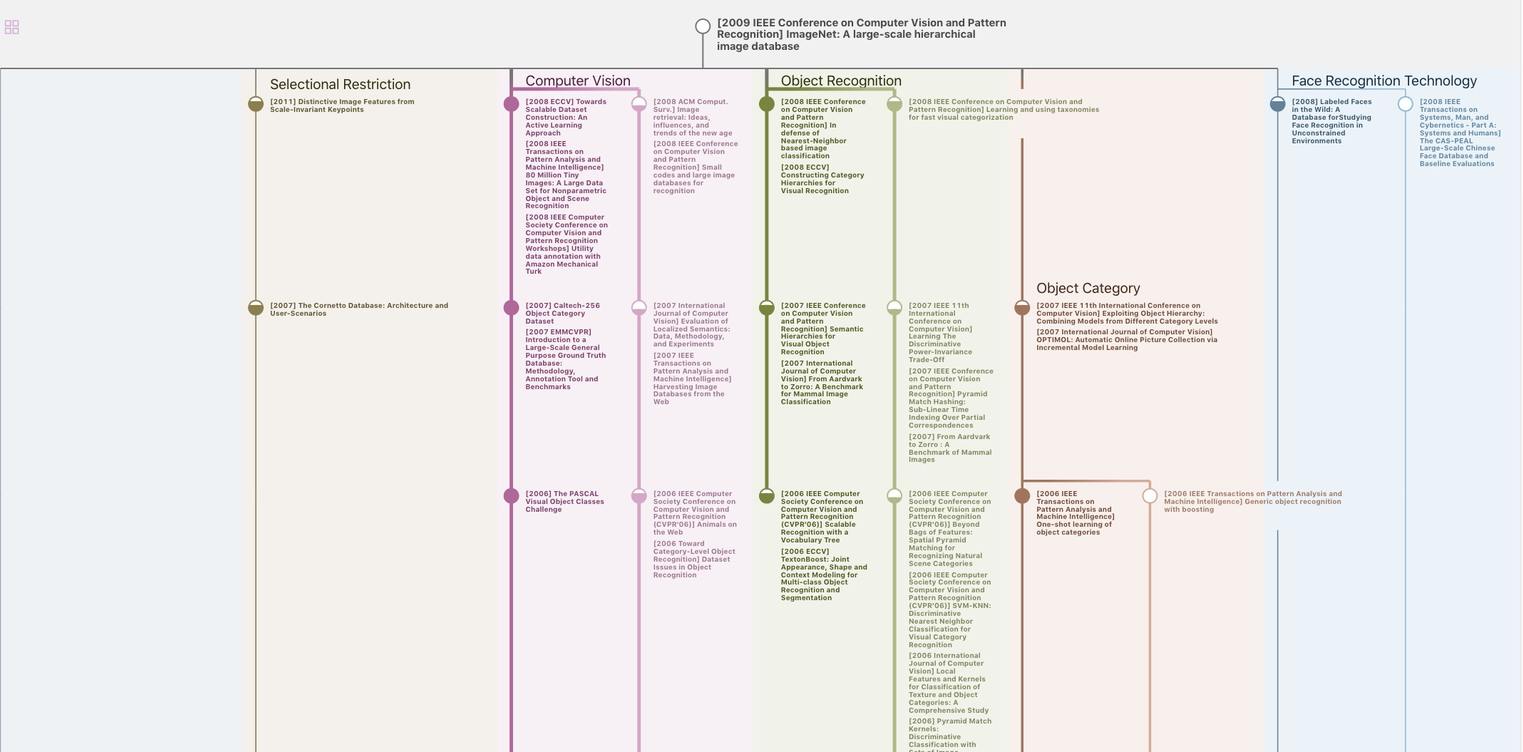
生成溯源树,研究论文发展脉络
Chat Paper
正在生成论文摘要