Parallel Metaheuristic Algorithms for Solving Imbalanced Data Classification Problems
IEEE Access(2023)
摘要
An imbalanced classification problem is one in which the distribution of instances across defined classes is uneven or biased in one direction or another. In data mining, the probabilistic neural network (PNN) classifier is a well-known technology that has been successfully used to solve a variety of classification difficulties. On the other hand, metaheuristic optimization approaches offer an excellent means by which to deal with this problem. Therefore, this work combines two metaheuristic algorithms–the Ali Baba and the Forty Thieves (AFT) algorithm and the Water Strider Algorithm (WSA)–in order to alter the weights of a PNN classifier for imbalanced datasets. This article introduces a self-contained multiple-search approach for parallel metaheuristics that may be used in a variety of situations. Most implementations begin many search processes, all of which utilize the same search algorithm, with a set of starting parameters that are all generated separately. Most implementations pick a processor to collect data and verify the data for compliance with some stopping criteria, with the latter being the default. In the proposed AFT-WSA parallel method, the two algorithms begin simultaneously, and the fitness value is communicated in each iteration to find the best classification accuracy in the smallest number of iterations, thereby allowing the weight of the PNN classifier to be adjusted. In this study, ten imbalanced public datasets were used to test the performance of the proposed approach in terms of classification accuracy, standard deviation, and F-measure.
更多查看译文
关键词
Ali Baba and the Forty Thieves algorithm,imbalanced data,parallel metaheuristic,PNN classifier,water strider algorithm
AI 理解论文
溯源树
样例
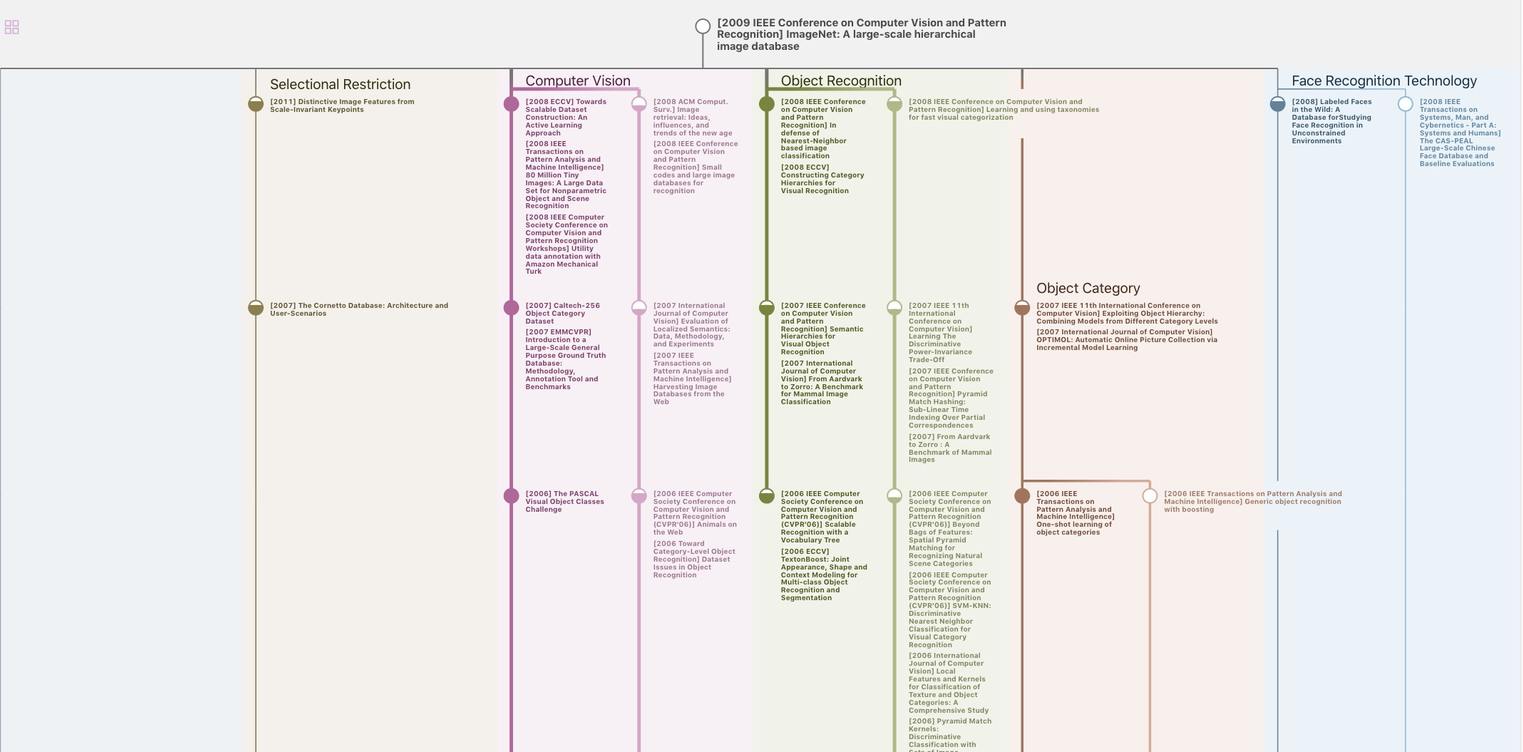
生成溯源树,研究论文发展脉络
Chat Paper
正在生成论文摘要