Achieving a Zero False Negative Rate Unsupervised Detection on Controller Area Network (CAN) Bus with LSTM-Based Autoencoder
2023 International Mobile, Intelligent, and Ubiquitous Computing Conference (MIUCC)(2023)
摘要
The increasing complexity and connectivity of modern automotive systems have introduced new challenges in ensuring the security and reliability of Controller Area Network (CAN) bus communication. Cyber-attacks on CAN bus networks can lead to severe consequences, including vehicle malfunctions and compromise of passenger safety. As a result, there is a pressing need for efficient and effective anomaly detection mechanisms to identify and mitigate potential security breaches. TThis paper presents a practical anomaly detection approach that harnesses the capabilities of autoencoders and Long Short-Term Memory (LSTM) neural networks to fortify CAN bus cybersecurity with a focus on real-world implementation. The proposed method leverages the autoencoder's ability to learn the normal behavior of the CAN bus traffic and identify deviations from it, while the LSTM architecture provides temporal sequence modeling to capture dynamic patterns in the data.
更多查看译文
关键词
anomaly detection,automotive,LSTM,Controller Area Network,autoencoder
AI 理解论文
溯源树
样例
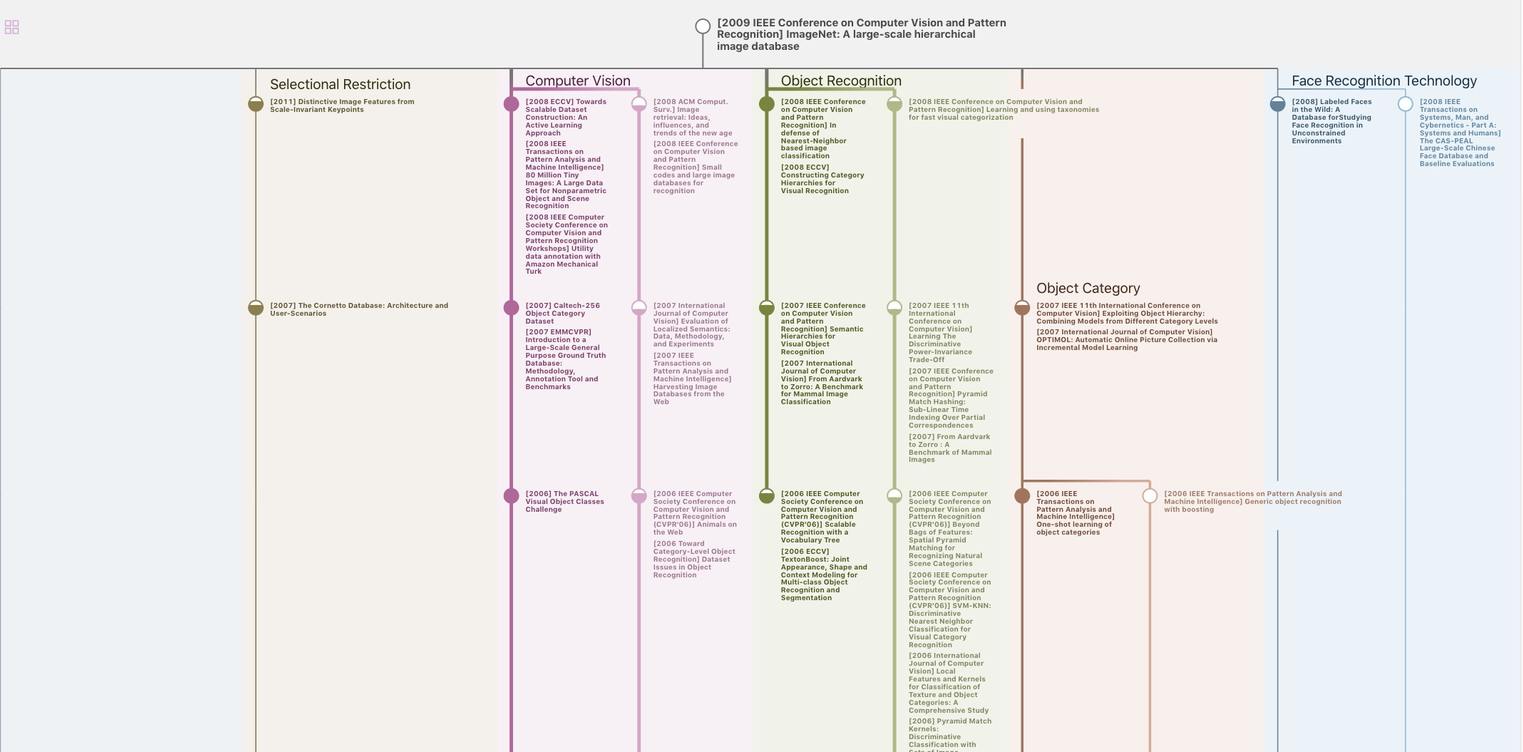
生成溯源树,研究论文发展脉络
Chat Paper
正在生成论文摘要