Multitask Learning Using Feature Extraction Network for Smart Tourism Applications
IEEE INTERNET OF THINGS JOURNAL(2023)
摘要
Recently around half of the world's current population resides in urban areas and benefit from rich services in the smart city. The majority of smart city services are recommendation-related services, and with the development of Internet, most recommendation services in smart economy are online recommendations. Online travel platforms (OTPs) (like Booking, Airbnb, Ctrip, and Fliggy) provide people sufficient resources and convenient approaches to plan and enjoy their trips in smart city. Hotel recommendation is essential for the success of OTPs. However, it is more challenging compared to item recommendation in typical E-commerce scenarios (e.g., Taobao, Jd, and YouTube). The in-nature characteristics of low-frequency and high unit-price lead to more severe sparse and long-tail data distributions. Moreover, for enhancing user experience and business returns, the recommender system seeks to improve both click-through rate (CTR) and conversion rate (CVR) where the seesaw phenomenon may occur. In order to address the aforementioned shortages in hotel recommendation, a multitask learning (MTL) method with a novel flexible multilevel extraction network [denoted as flexible MTL (FMTL)] is proposed. Particularly, FMTL takes MTL into consideration in a unified representation learning framework and is divided into feature encoding and task prediction. In the feature encoding phase, we introduce a novel multirepresentation extractor with temperature-adjusted gating mechanism (T-MRE) for each task, producing more flexible representations for sparse and long-tail data. Moreover, we fuse different representations for each task with three strategies during the prediction phase and empirically demonstrate that the simple concatenation strategy is superior than other relatively complex gating approaches. Offline and live experiments with regard to both overall metrics and user group analysis based on the scarcity of user behaviors illustrate that without significantly increasing model parameters, our FMTL model outperforms substantially over several state-of-the-art models.
更多查看译文
关键词
Task analysis,Multitasking,Encoding,Predictive models,Feature extraction,Smart cities,Training,Multitask learning (MTL),parameter sharing,smart service,temperature-adjusted gating,transfer learning
AI 理解论文
溯源树
样例
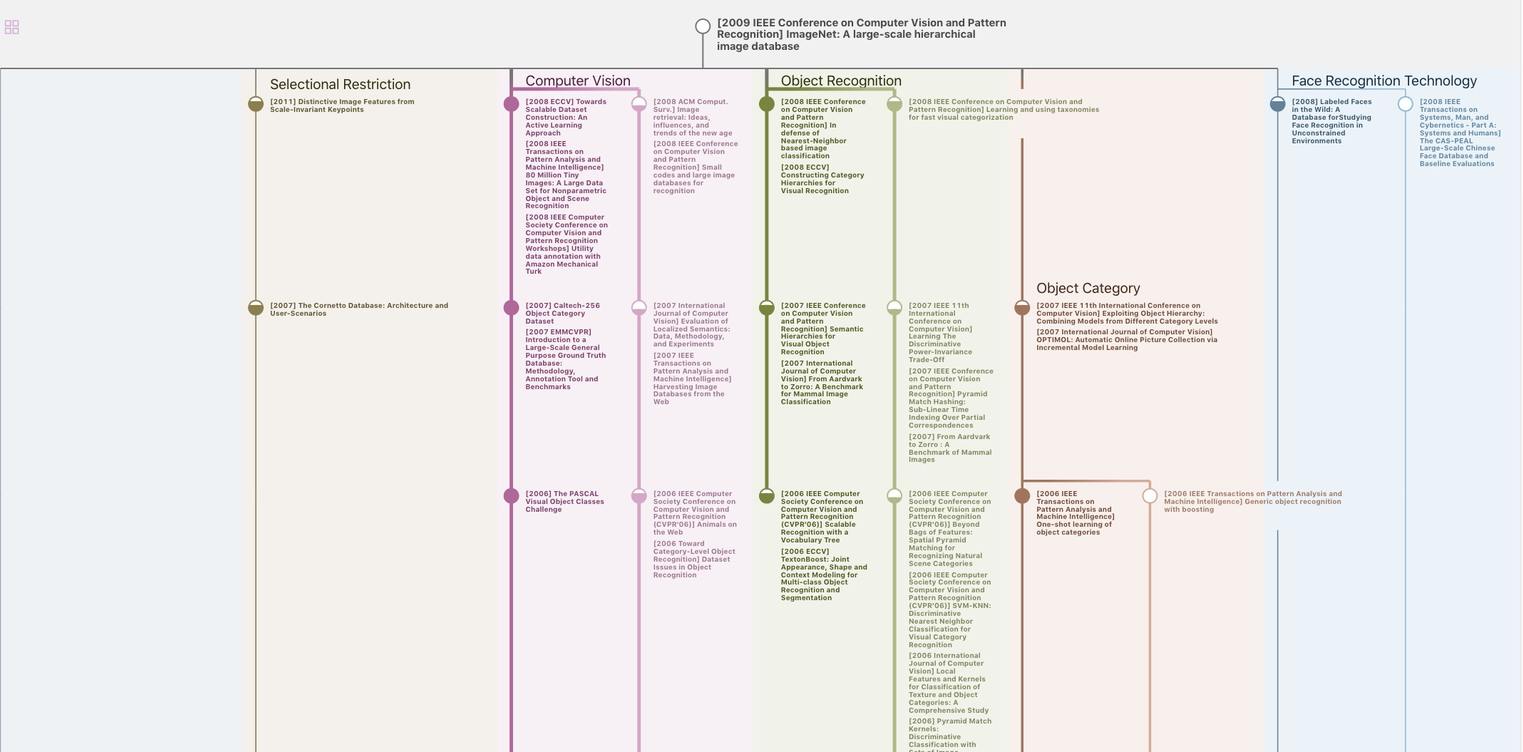
生成溯源树,研究论文发展脉络
Chat Paper
正在生成论文摘要