MG-ASTN: Multigraph Framework With Attentive SpatialCTemporal Networks for Crowd Mobility Prediction
IEEE Internet of Things Journal(2023)
摘要
Predicting urban crowd patterns/flows is a challenging task due to complex spatial-temporal (ST) dependencies. In this article, we aim to examine and report the capability and effectiveness of the current most widely used graph convolutional networks (GCNs) on mobile data analysis in ST networks for crowd flow forecasting. Specifically, we propose a novel dual-stream framework leveraging multigraph with attentive ST networks (MG-ASTN) to simultaneously predict crowd in-out flow and origin-destination (OD) flow based on the trajectory data collected by on-board devices (e.g., GPS). MG-ASTN utilizes multi-GCNs encoding non-Euclidean correlations to explore pairwise relationships among regions. In addition, we further apply a cross-channel attention mechanism with 3-D temporal convolutional network to address the heterogeneity of ST features and capture more meaningful data representations for multitask learning. In the evaluation, we conduct experiments based on two real-world data sets and verify most well-known state-of-the-art methods for crowd flow prediction. The results demonstrate that MG-ASTN could outperform other solutions-in-out flow prediction with lowest RMSE and MAE, and OD flow prediction beyond others in most cases, thus it has great potential in modeling the complex correlations among regions in ST networks and enabling accurate prediction in urban computing.
更多查看译文
关键词
Correlation,Task analysis,Convolutional neural networks,Computer science,Trajectory,Predictive models,Encoding,Flow prediction,graph convolutional networks (GCNs),mobile data analysis,urban computing
AI 理解论文
溯源树
样例
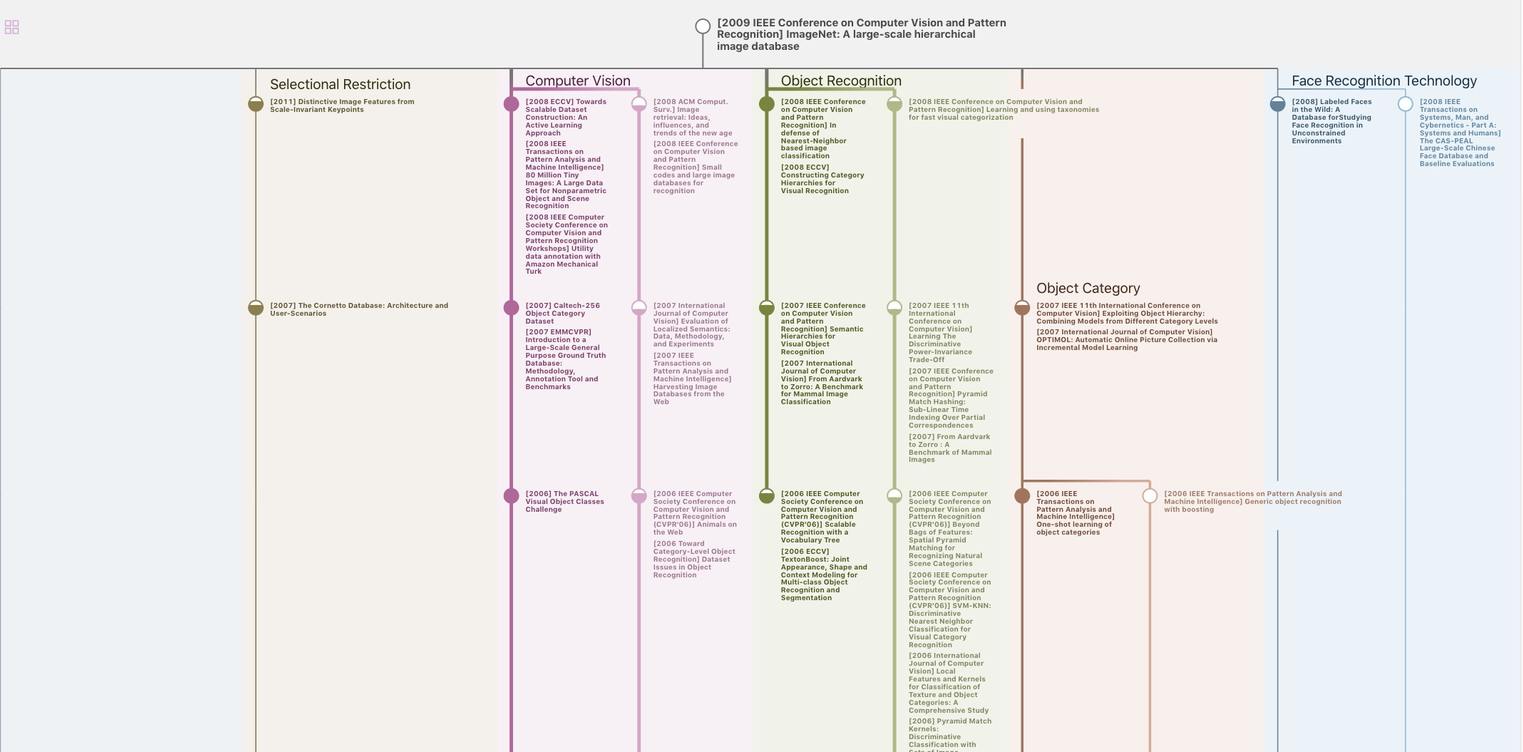
生成溯源树,研究论文发展脉络
Chat Paper
正在生成论文摘要