Robust RF Fingerprint Extraction Based on Cyclic Shift Characteristic
IEEE INTERNET OF THINGS JOURNAL(2023)
摘要
As a novel solution for identification of Internet of Things (IoT) devices, radio frequency fingerprint (RFF) has excellent features, such as uniqueness, stability, and nonreproducibility. We propose an RFF extraction method for a type of signals with the cyclic shift characteristic (CSC). The extracted RFF is robust in terms of data independence, noise resistance, and channel immunity. A unified RFF representation is derived by the cross-power spectral density (CPSD)-based extraction method, which utilizes all different symbols within one signal frame under the random sending symbol conditions. Theoretical analysis demonstrates that this representation can be superimposed to obtain effective device identification performance under the low signal-to-noise ratio (SNR) conditions. Additionally, a preamble spectrum correlation (PSC) algorithm is proposed to fast estimate and compensate the large carrier frequency offset (CFO) in the preprocessing process of CPSD-based feature extraction. Our method is verified by conducting extensive experiments with over 34000 frames from 60 ZigBee devices in various scenarios, including indoor/outdoor and line-of-sight/non-LOS (LOS/NLOS). The identification accuracy reaches 96.66% for 60 devices in the LOS scenario and 98.81% for 36 devices in the NLOS scenario both with the shortest frame compared to the existing methods in practice. Thanks to the robust RFF extraction, experimental results show that our method adopting a simple linear discriminant analysis (LDA) classifier outperforms the state-of-the-art RFF identification methods using deep learning-based neural networks.
更多查看译文
关键词
Symbols,Internet of Things,Feature extraction,Zigbee,Object recognition,Signal to noise ratio,Wireless communication,Carrier frequency offset (CFO) estimation,cyclic shift characteristic (CSC),physical layer security,radio frequency fingerprint (RFF)
AI 理解论文
溯源树
样例
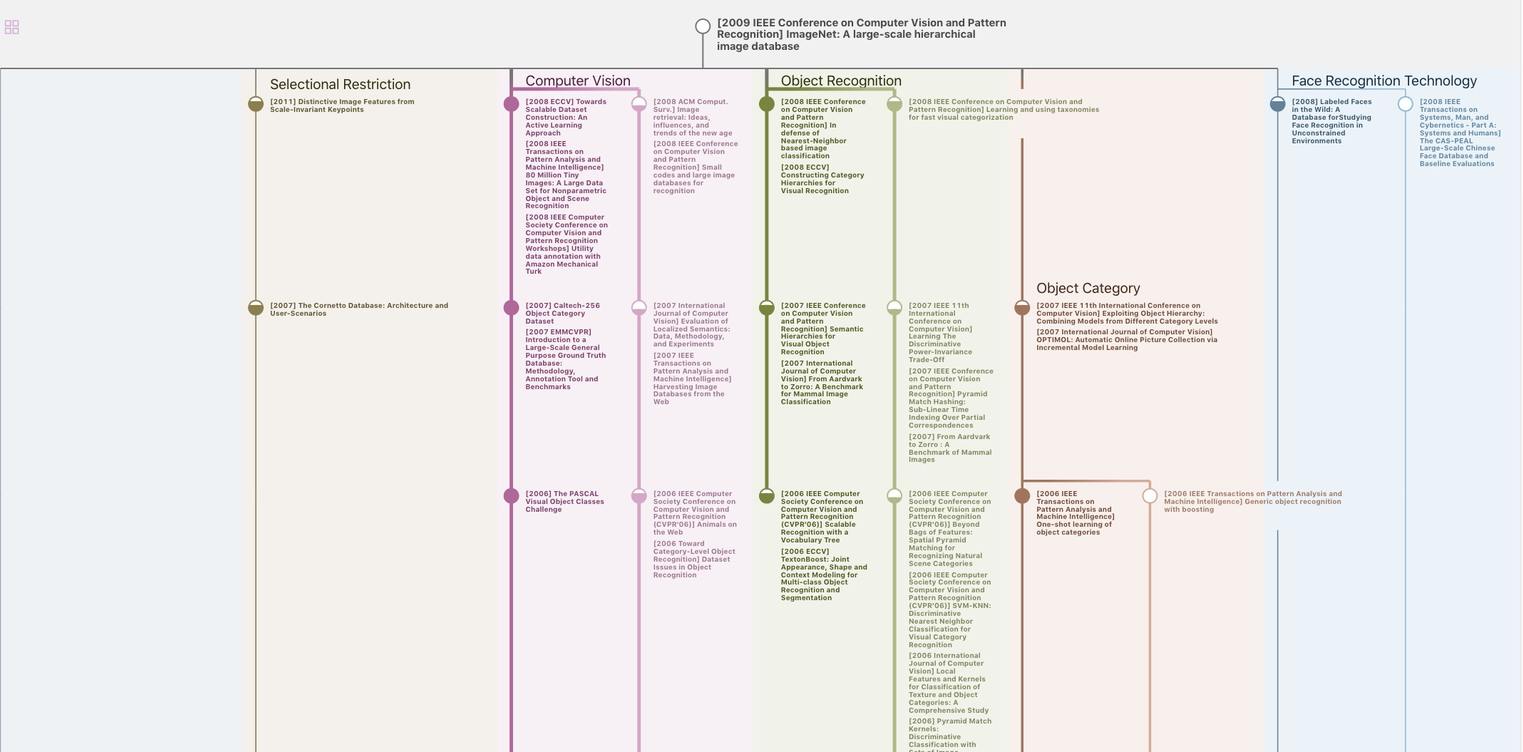
生成溯源树,研究论文发展脉络
Chat Paper
正在生成论文摘要