Graph Neural Networks with polynomial activations have limited expressivity
CoRR(2023)
摘要
The expressivity of Graph Neural Networks (GNNs) can be entirely
characterized by appropriate fragments of the first order logic. Namely, any
query of the two variable fragment of graded modal logic (GC2) interpreted over
labeled graphs can be expressed using a GNN whose size depends only on the
depth of the query. As pointed out by [Barcelo Al., 2020, Grohe, 2021], this
description holds for a family of activation functions, leaving the
possibibility for a hierarchy of logics expressible by GNNs depending on the
chosen activation function. In this article, we show that such hierarchy indeed
exists by proving that GC2 queries cannot be expressed by GNNs with polynomial
activation functions. This implies a separation between polynomial and popular
non polynomial activations (such as Rectified Linear Units) and answers an open
question formulated by [Grohe, 21].
更多查看译文
关键词
polynomial activations,neural networks,graph
AI 理解论文
溯源树
样例
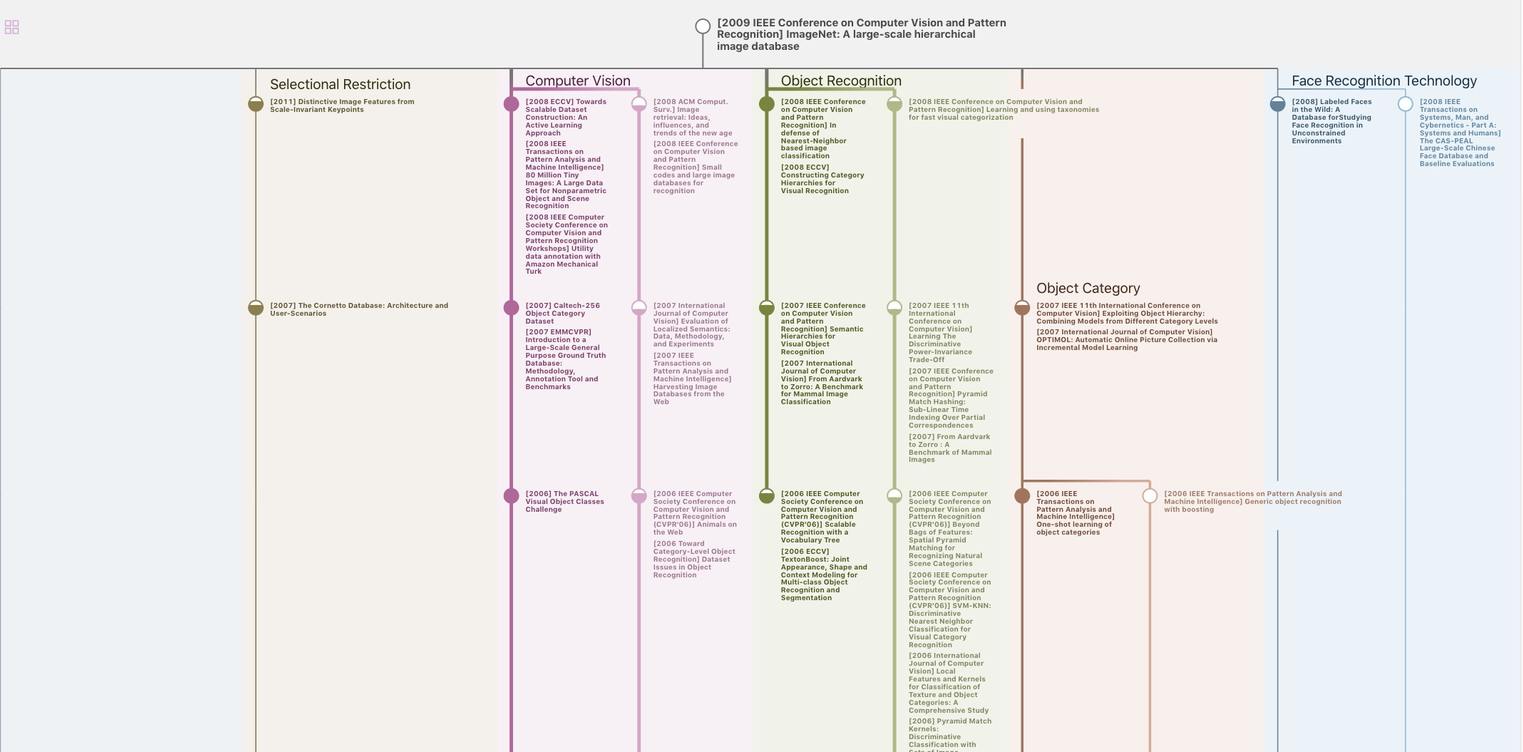
生成溯源树,研究论文发展脉络
Chat Paper
正在生成论文摘要