On the Language Encoder of Contrastive Cross-modal Models
CoRR(2023)
摘要
Contrastive cross-modal models such as CLIP and CLAP aid various vision-language (VL) and audio-language (AL) tasks. However, there has been limited investigation of and improvement in their language encoder, which is the central component of encoding natural language descriptions of image/audio into vector representations. We extensively evaluate how unsupervised and supervised sentence embedding training affect language encoder quality and cross-modal task performance. In VL pretraining, we found that sentence embedding training language encoder quality and aids in cross-modal tasks, improving contrastive VL models such as CyCLIP. In contrast, AL pretraining benefits less from sentence embedding training, which may result from the limited amount of pretraining data. We analyze the representation spaces to understand the strengths of sentence embedding training, and find that it improves text-space uniformity, at the cost of decreased cross-modal alignment.
更多查看译文
关键词
language encoder,contrastive,models,cross-modal
AI 理解论文
溯源树
样例
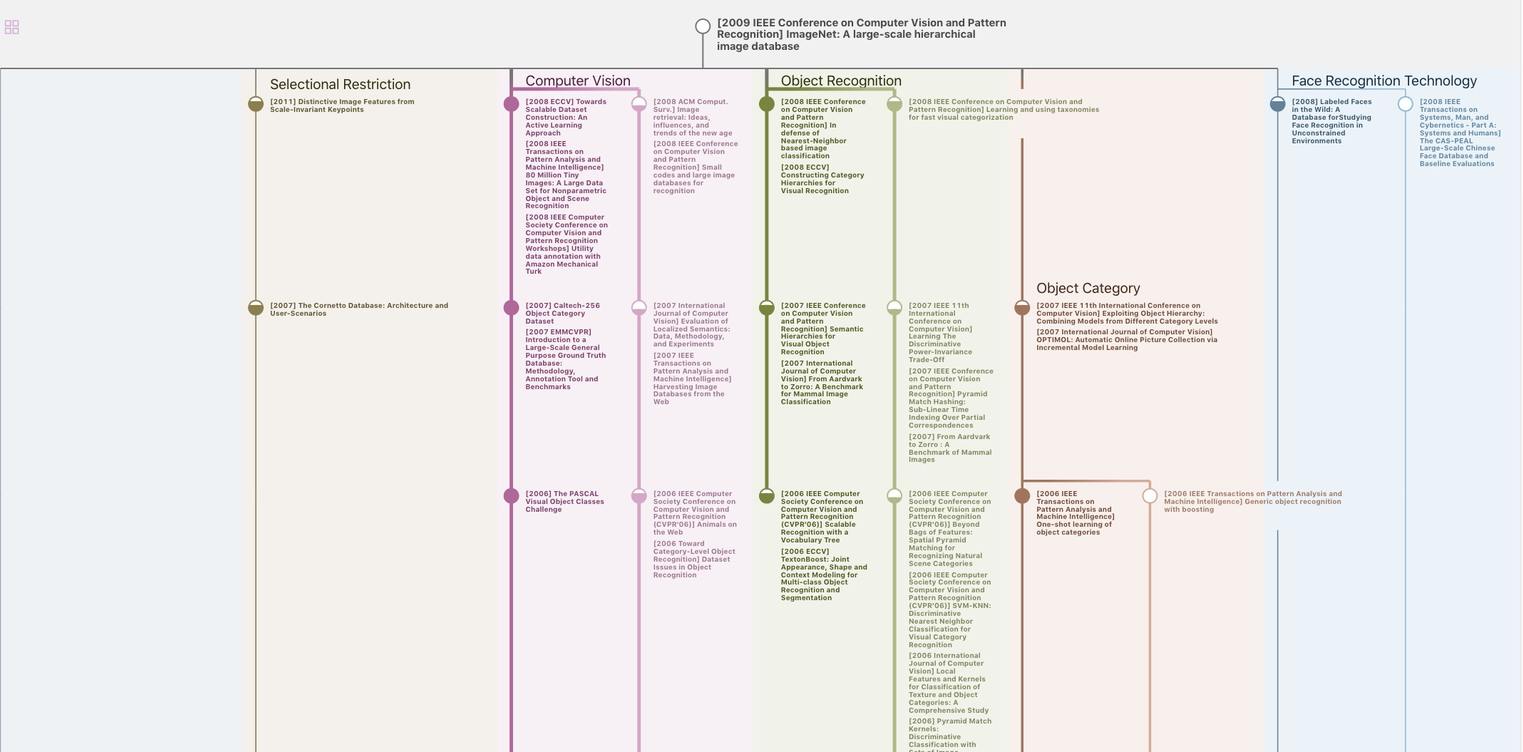
生成溯源树,研究论文发展脉络
Chat Paper
正在生成论文摘要