Controlled Randomness Improves the Performance of Transformer Models
2023 International Conference on Machine Learning and Applications (ICMLA)(2023)
摘要
During the pre-training step of natural language models, the main objective is to learn a general representation of the pre-training dataset, usually requiring large amounts of textual data to capture the complexity and diversity of natural language. Contrasting this, in most cases, the size of the data available to solve the specific downstream task is often dwarfed by the aforementioned pre-training dataset, especially in domains where data is scarce. We introduce controlled randomness, i.e. noise, into the training process to improve fine-tuning language models and explore the performance of targeted noise in addition to the parameters of these models. We find that adding such noise can improve the performance in our two downstream tasks of joint named entity recognition and relation extraction and text summarization.
更多查看译文
关键词
Natural Language Processing,Regularization,Transformer,Machine Learning
AI 理解论文
溯源树
样例
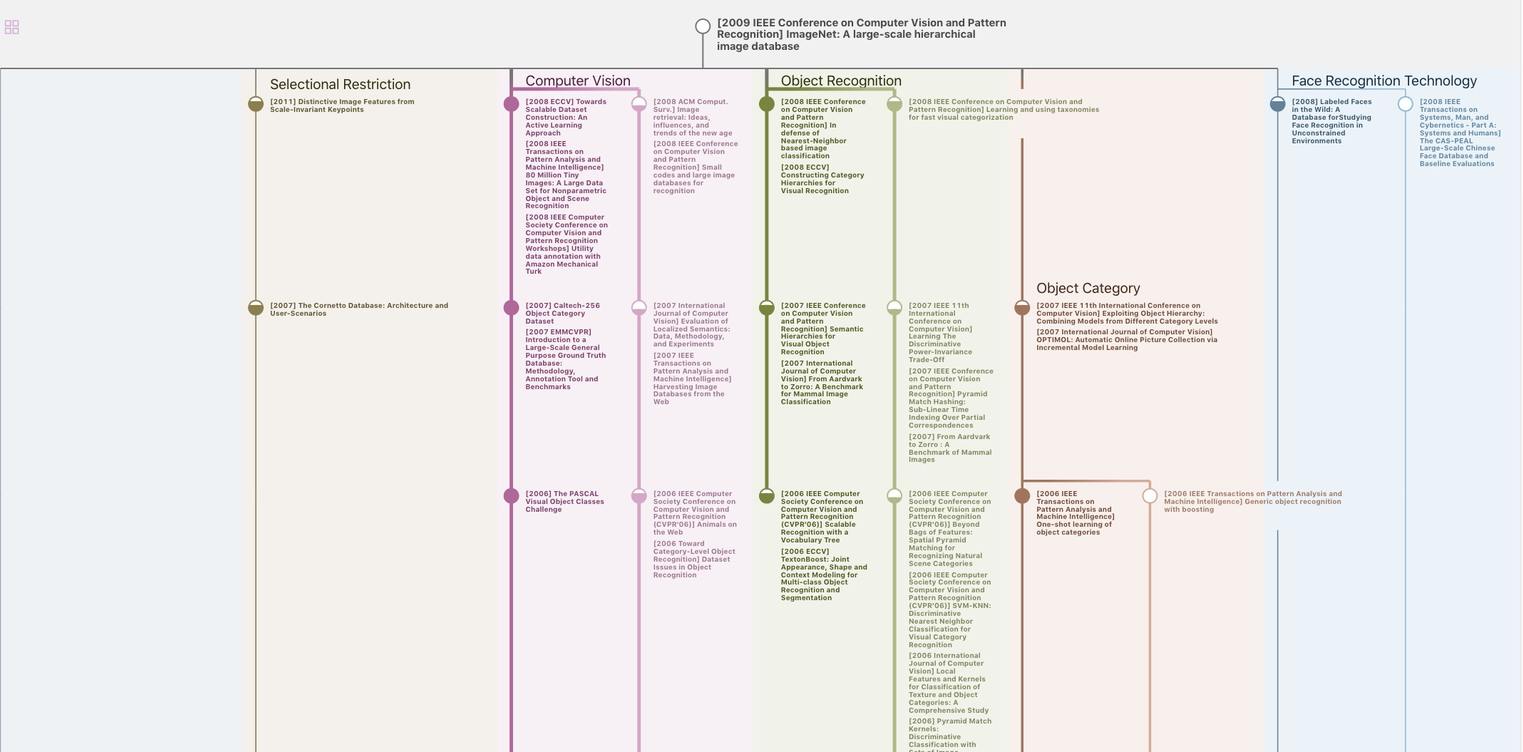
生成溯源树,研究论文发展脉络
Chat Paper
正在生成论文摘要