Evaluating Physically Motivated Loss Functions for Photometric Redshift Estimation
arXiv (Cornell University)(2023)
摘要
Physical constraints have been suggested to make neural network models more generalizable, act scientifically plausible, and be more data-efficient over unconstrained baselines. In this report, we present preliminary work on evaluating the effects of adding soft physical constraints to computer vision neural networks trained to estimate the conditional density of redshift on input galaxy images for the Sloan Digital Sky Survey. We introduce physically motivated soft constraint terms that are not implemented with differential or integral operators. We frame this work as a simple ablation study where the effect of including soft physical constraints is compared to an unconstrained baseline. We compare networks using standard point estimate metrics for photometric redshift estimation, as well as metrics to evaluate how faithful our conditional density estimate represents the probability over the ensemble of our test dataset. We find no evidence that the implemented soft physical constraints are more effective regularizers than augmentation.
更多查看译文
关键词
photometric redshift
AI 理解论文
溯源树
样例
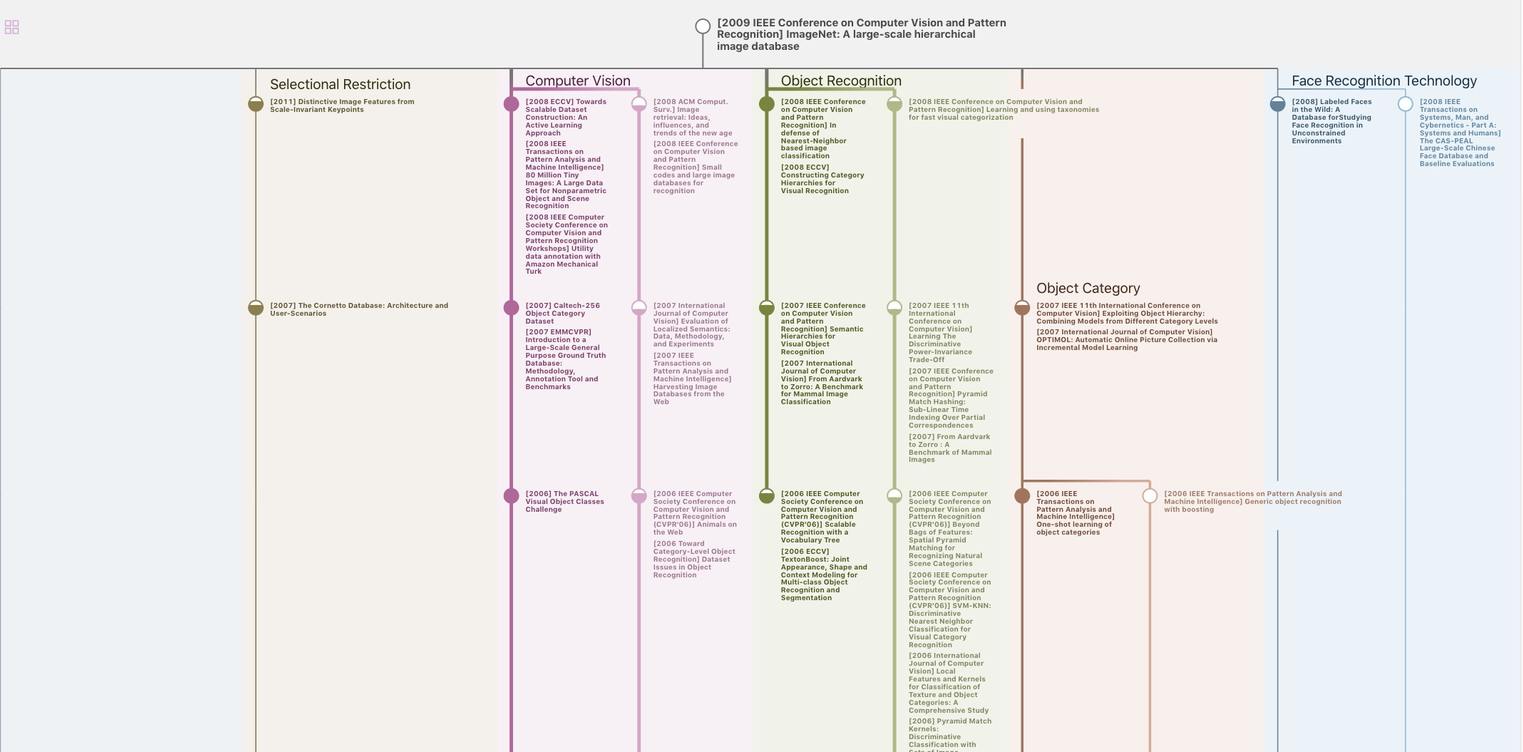
生成溯源树,研究论文发展脉络
Chat Paper
正在生成论文摘要