Dual-view Contrastive Learning for Auction Recommendation
PROCEEDINGS OF THE 32ND ACM INTERNATIONAL CONFERENCE ON INFORMATION AND KNOWLEDGE MANAGEMENT, CIKM 2023(2023)
摘要
Recommendation systems in auction platforms like eBay function differently in comparison to those found in traditional trading platforms. The bidding process involves multiple users competing for a product, with the highest bidder winning the item. As a result, each transaction is independent and characterized by varying transaction prices. The individual nature of auction items means that users cannot purchase identical items, adding to the uniqueness of the purchasing history. Bidders in auction systems rely on their judgment to determine the value of a product, as bidding prices reflect preferences rather than cost-free actions like clicking or collecting. Conventional methodologies that heavily rely on user-item purchase history are ill-suited to handle these unique and extreme product features. Unfortunately, prior recommendation approaches have failed to give due attention to the contextual intricacies of auction items, thereby missing out on the full potential of the invaluable bidding record at hand. This paper introduces a novel contrastive learning approach for auction recommendation, addressing the challenges of data sparsity and uniqueness in auction recommendation. Our method focuses on capturing multiple behavior relations and item context through contrastive pairs construction, contrastive embedding, and contrastive optimization techniques from both user and item perspectives. By overcoming the limitations of previous approaches, our method delivers promising results on two auction datasets, highlighting the practicality and effectiveness of our model.
更多查看译文
关键词
Auction Recommendation,Contrastive Learning,Multiple Relations,Semantic Commonality
AI 理解论文
溯源树
样例
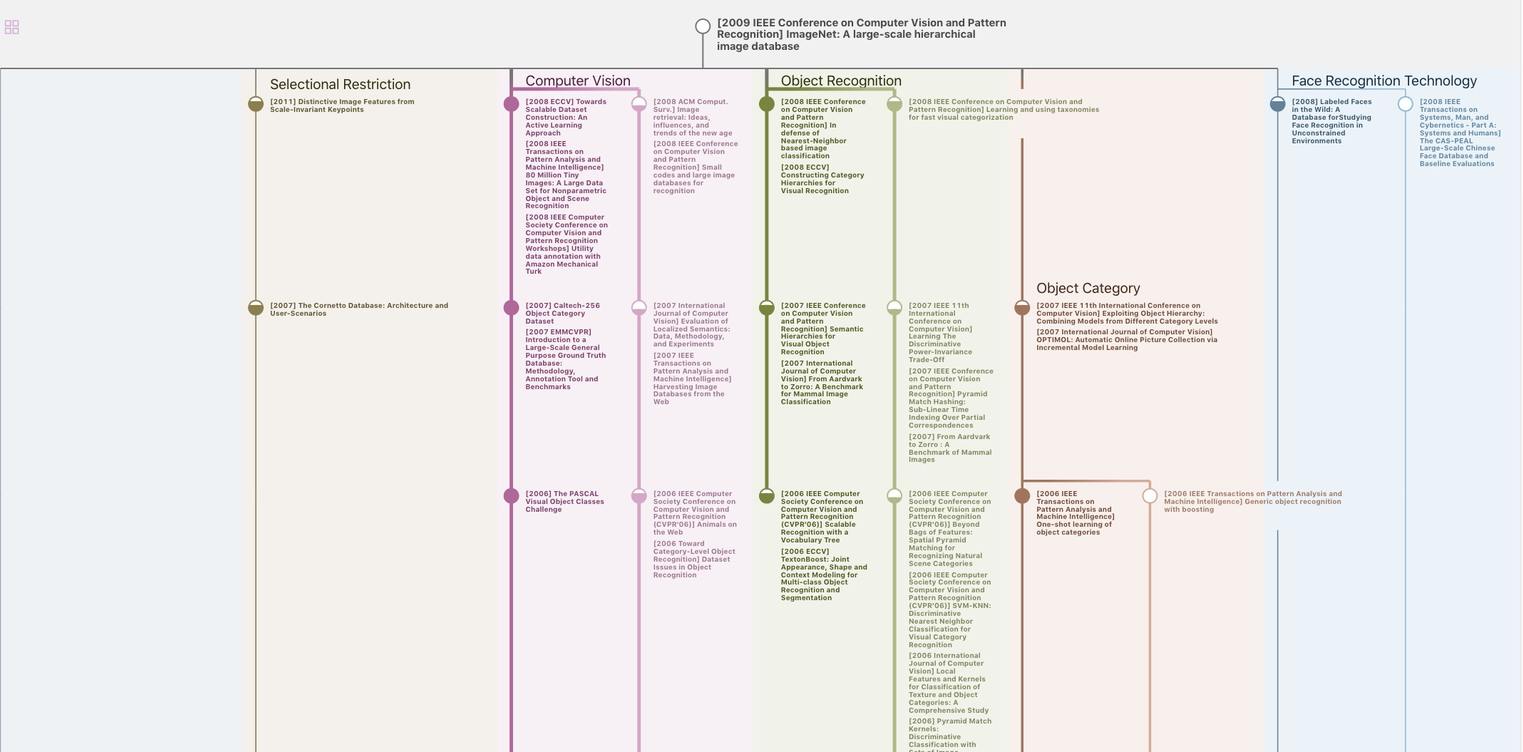
生成溯源树,研究论文发展脉络
Chat Paper
正在生成论文摘要