Improving Graph Domain Adaptation with Network Hierarchy
PROCEEDINGS OF THE 32ND ACM INTERNATIONAL CONFERENCE ON INFORMATION AND KNOWLEDGE MANAGEMENT, CIKM 2023(2023)
Abstract
Graph domain adaptation models have become instrumental in addressing cross-network learning problems due to their ability to transfer abundant label and structural knowledge from source graphs to target graphs. A crucial step in transfer involves measuring domain discrepancy, which refers to distribution shifts between graphs from source and target domains. While conventional models simply provide a node-level measurement, exploiting information from different levels of network hierarchy is intuitive. As each hierarchical level characterizes distinct and meaningful properties or functionalities of the original graph, integrating domain discrepancy based on such hierarchies should contribute to a more precise domain discrepancy measurement. Moreover, class conditional distribution shift is often overlooked in node classification tasks, which could potentially lead to sub-optimal performance. To address the above limitations, we propose a new graph domain adaptation model and apply it to cross-network node classification tasks. Specifically, a hierarchical pooling model to extract meaningful and adaptive hierarchical structures is designed, where both marginal and class conditional distribution shifts on each hierarchical level are jointly minimized. The effectiveness is demonstrated through theoretical analysis and experimental studies across various datasets.
MoreTranslated text
Key words
Graph domain adaptation,Cross-network learning,Graph neural network,Transfer learning
AI Read Science
Must-Reading Tree
Example
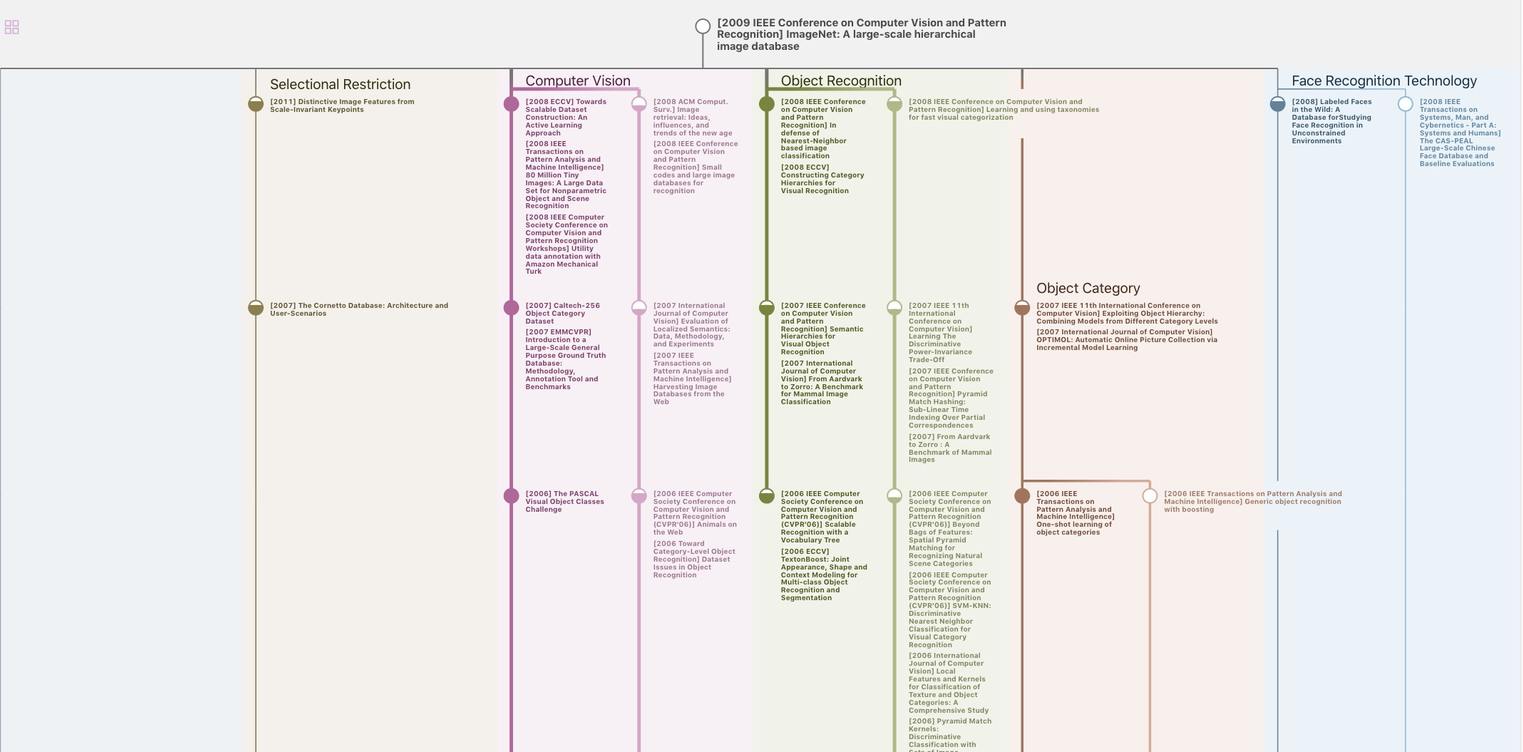
Generate MRT to find the research sequence of this paper
Chat Paper
Summary is being generated by the instructions you defined