Task-Difficulty-Aware Meta-Learning with Adaptive Update Strategies for User Cold-Start Recommendation
PROCEEDINGS OF THE 32ND ACM INTERNATIONAL CONFERENCE ON INFORMATION AND KNOWLEDGE MANAGEMENT, CIKM 2023(2023)
摘要
User cold-start recommendation is one of the most challenging problems that limit the effectiveness of recommender systems. Meta-learning-based methods are introduced to address this problem by learning initialization parameters for cold-start tasks. Recent studies attempt to enhance the initialization methods. They first represent each task by the cold-start user and interacted items. Then they distinguish tasks based on the task relevance to learn adaptive initialization. However, this manner is based on the assumption that user preferences can be reflected by the interacted items saliently, which is not always true in reality. In addition, we argue that previous approaches suffer from their adaptive framework (e.g., adaptive initialization), which reduces the adaptability in the process of transferring meta-knowledge to personalized RSs. In response to the issues, we propose a task-difficulty-aware meta-learning with adaptive update strategies (TDAS) for user cold-start recommendation. First, we design a task difficulty encoder, which can represent user preference salience, task relevance, and other task characteristics by modeling task difficulty information. Second, we adopt a novel framework with task-adaptive local update strategies by optimizing the initialization parameters with task-adaptive per-step and per-layer hyperparameters. Extensive experiments based on three real-world datasets demonstrate that our TDAS outperforms the state-of-the-art methods. The source code is available at https://github.com/XuHao-bit/TDAS.
更多查看译文
关键词
Recommendation,User cold-start problem,Meta-learning
AI 理解论文
溯源树
样例
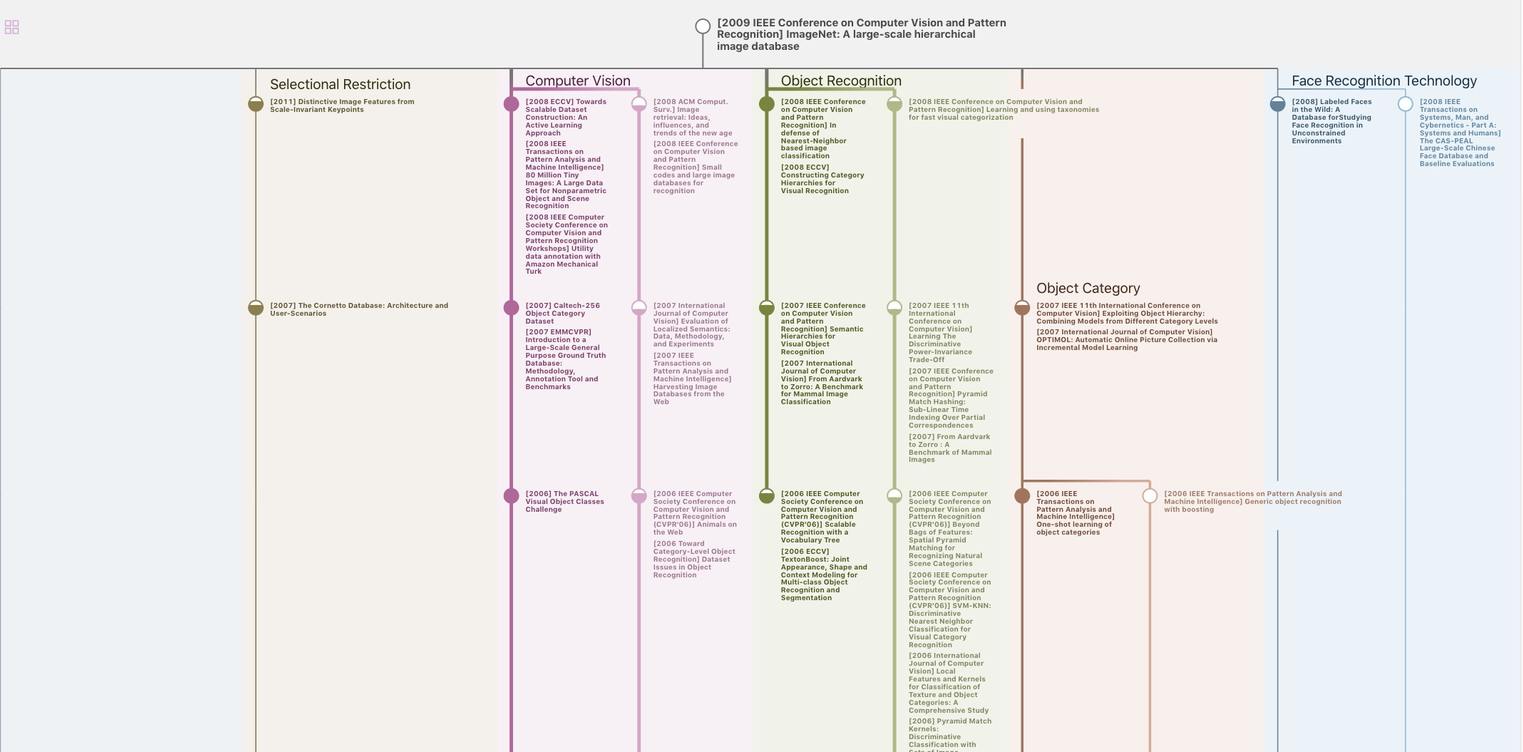
生成溯源树,研究论文发展脉络
Chat Paper
正在生成论文摘要