Towards Fair Financial Services for All: A Temporal GNN Approach for Individual Fairness on Transaction Networks
PROCEEDINGS OF THE 32ND ACM INTERNATIONAL CONFERENCE ON INFORMATION AND KNOWLEDGE MANAGEMENT, CIKM 2023(2023)
摘要
Discrimination against minority groups within the banking sector has long resulted in unequal treatment in financial services. Recent works in the general machine learning domain can promote group fairness for predictions on static tabular data, but their direct application in finance often proves ineffective. Financial losses of banks may arise from inaccurate predictions due to the overlooked dynamic nature of data, and illegal discrimination against some individual clients could still occur since fairness is promoted on the subgroup level. Therefore, we model the data as a dynamic or temporal transaction network for better utility and investigate individual fairness on this dynamic graph for the loan approval task. We define two novel individual fairness properties on temporal graphs with a theoretical analysis of their respective regret. Using these notions, we design a temporally fair graph neural network (TF-GNN) approach under a new real-time evaluation scheme for dynamic transaction networks. Experiments on real-world datasets demonstrate the superiority of the proposed method for both utility improvement in accuracy and fairness promotion in NDCG@k.
更多查看译文
关键词
temporal GNNs,individual fairness,transaction networks
AI 理解论文
溯源树
样例
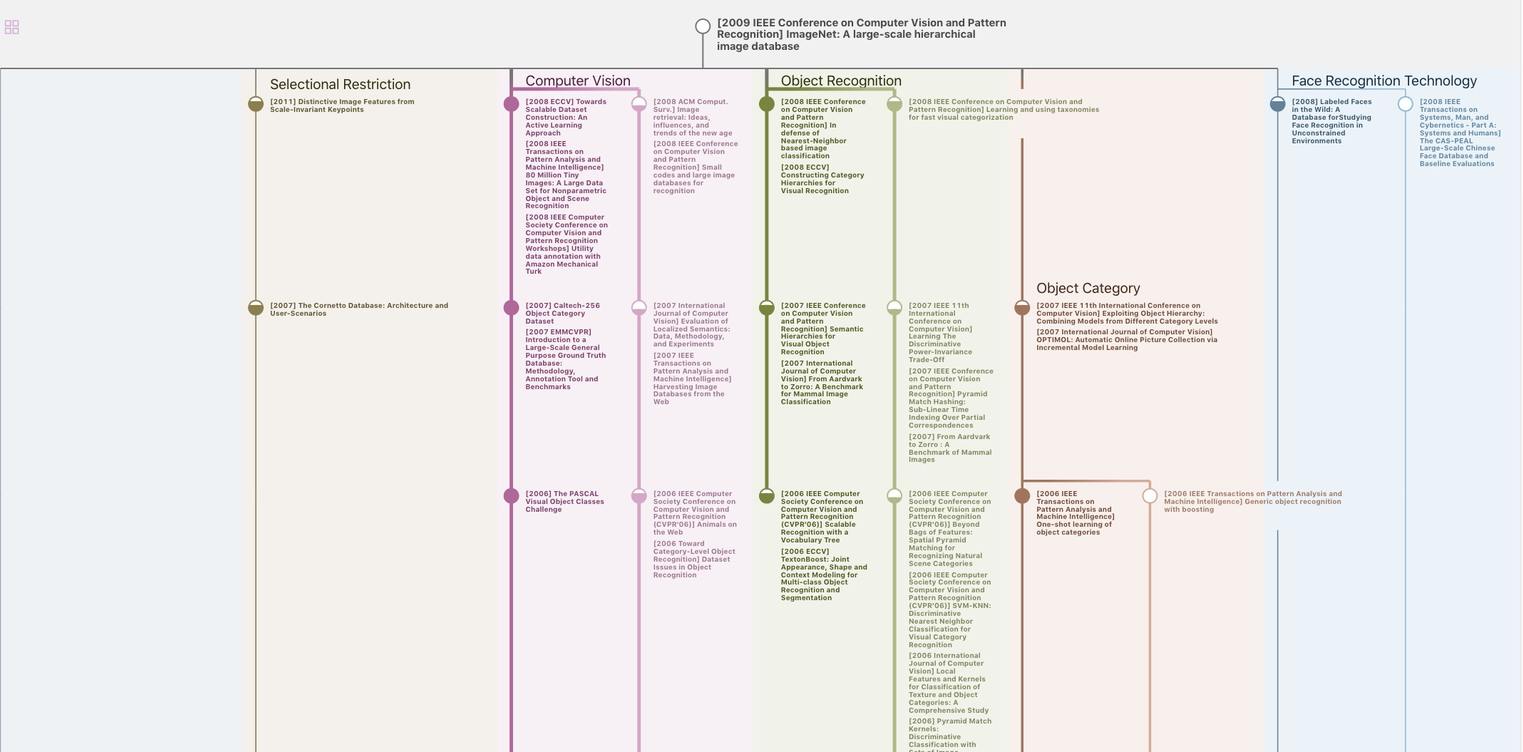
生成溯源树,研究论文发展脉络
Chat Paper
正在生成论文摘要