Bias Invariant Approaches for Improving Word Embedding Fairness
PROCEEDINGS OF THE 32ND ACM INTERNATIONAL CONFERENCE ON INFORMATION AND KNOWLEDGE MANAGEMENT, CIKM 2023(2023)
摘要
Many public pre-trained word embeddings have been shown to encode different types of biases. Embeddings are often obtained from training on large pre-existing corpora, and therefore resulting biases can be a reflection of unfair representations in the original data. Bias, in this scenario, is a challenging problem since current mitigation techniques require knowing and understanding existing biases in the embedding, which is not always possible. In this work, we propose to improve word embedding fairness by borrowing methods from the field of data privacy. The idea behind this approach is to treat bias as if it were a special type of training data leakage. This has the unique advantage of not requiring prior knowledge of potential biases in word embeddings. We investigated two types of privacy algorithms, and measured their effect on bias using four different metrics. To investigate techniques from differential privacy, we applied Gaussian perturbation to public pre-trained word embeddings. To investigate noiseless privacy, we applied vector quantization during training. Experiments show that both approaches improve fairness for commonly used embeddings, and additionally, noiseless privacy techniques reduce the size of the resulting embedding representation.
更多查看译文
关键词
Word Embedding,Fairness,Privacy
AI 理解论文
溯源树
样例
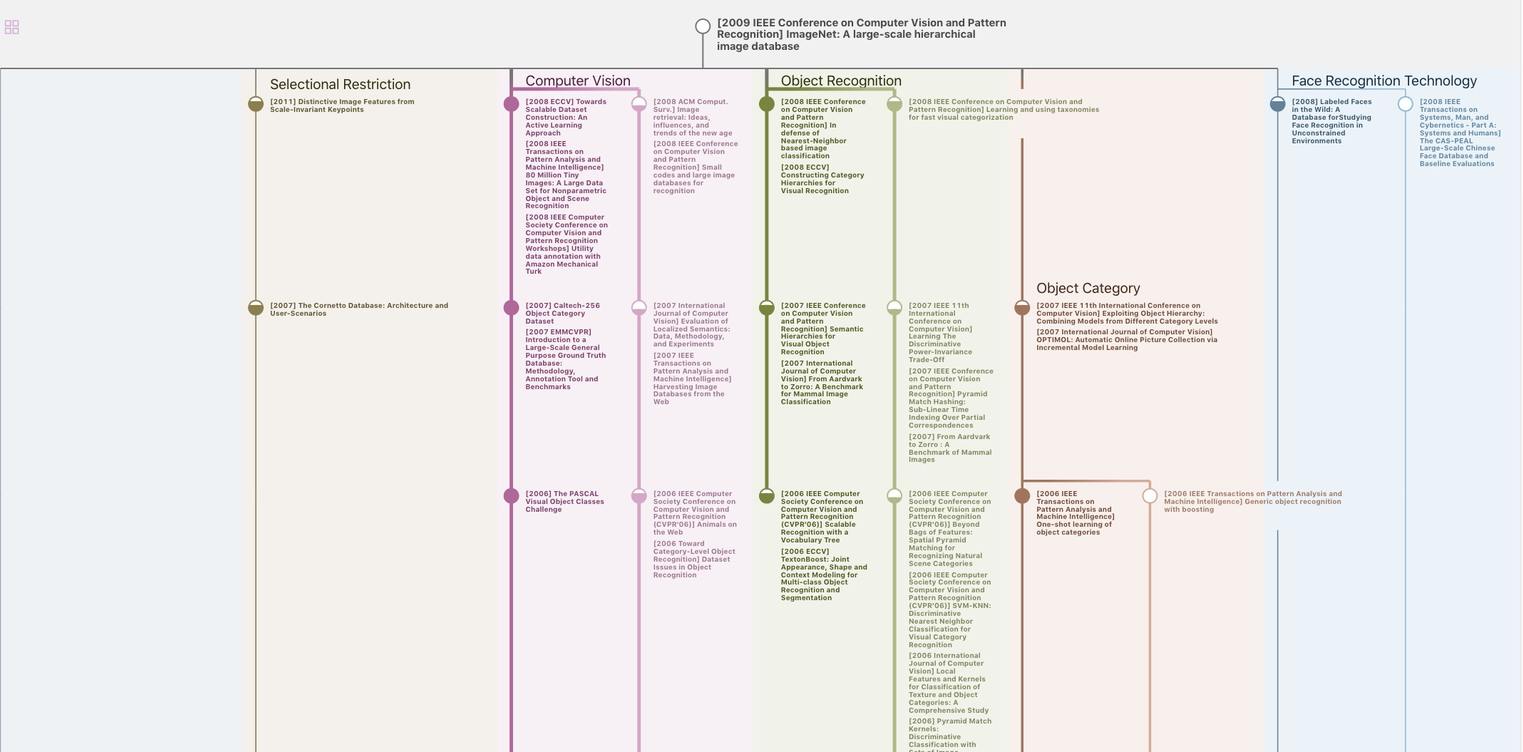
生成溯源树,研究论文发展脉络
Chat Paper
正在生成论文摘要