Hierarchical Multi-Label Classification with Partial Labels and Unknown Hierarchy
PROCEEDINGS OF THE 32ND ACM INTERNATIONAL CONFERENCE ON INFORMATION AND KNOWLEDGE MANAGEMENT, CIKM 2023(2023)
摘要
Hierarchical multi-label classification aims at learning a multi-label classifier from a dataset whose labels are organized into a hierarchical structure. To the best of our knowledge, we propose for the first time the problem of finding a multi-label classifier given a partially labeled hierarchical multi-label dataset. We also assume the situation where the classifier cannot access hierarchical information during training. This work proposes an iterative framework for learning both multi-labels and a hierarchical structure of classes. When training a multi-label classifier from partial labels, our model extracts a class hierarchy from the classifier output using our hierarchy extraction algorithm. Then, our proposed loss exploits the extracted hierarchy to train the classifier. Theoretically, we show that our hierarchy extraction algorithm correctly finds the unknown hierarchy under a mild condition, and we prove that our loss function of multi-label classification with such hierarchy becomes an unbiased estimator of true multi-label classification risk. Our experiments show that our model obtains a class hierarchy close to the ground-truth dataset hierarchy, and simultaneously, our method outperforms previous methods for hierarchical multi-label classification and multi-label classification from partial labels.
更多查看译文
关键词
Multi-label classification,Hierarchical multi-label classification,Multi-label learning from partial labels,Weakly supervised learning,Semi-supervised learning
AI 理解论文
溯源树
样例
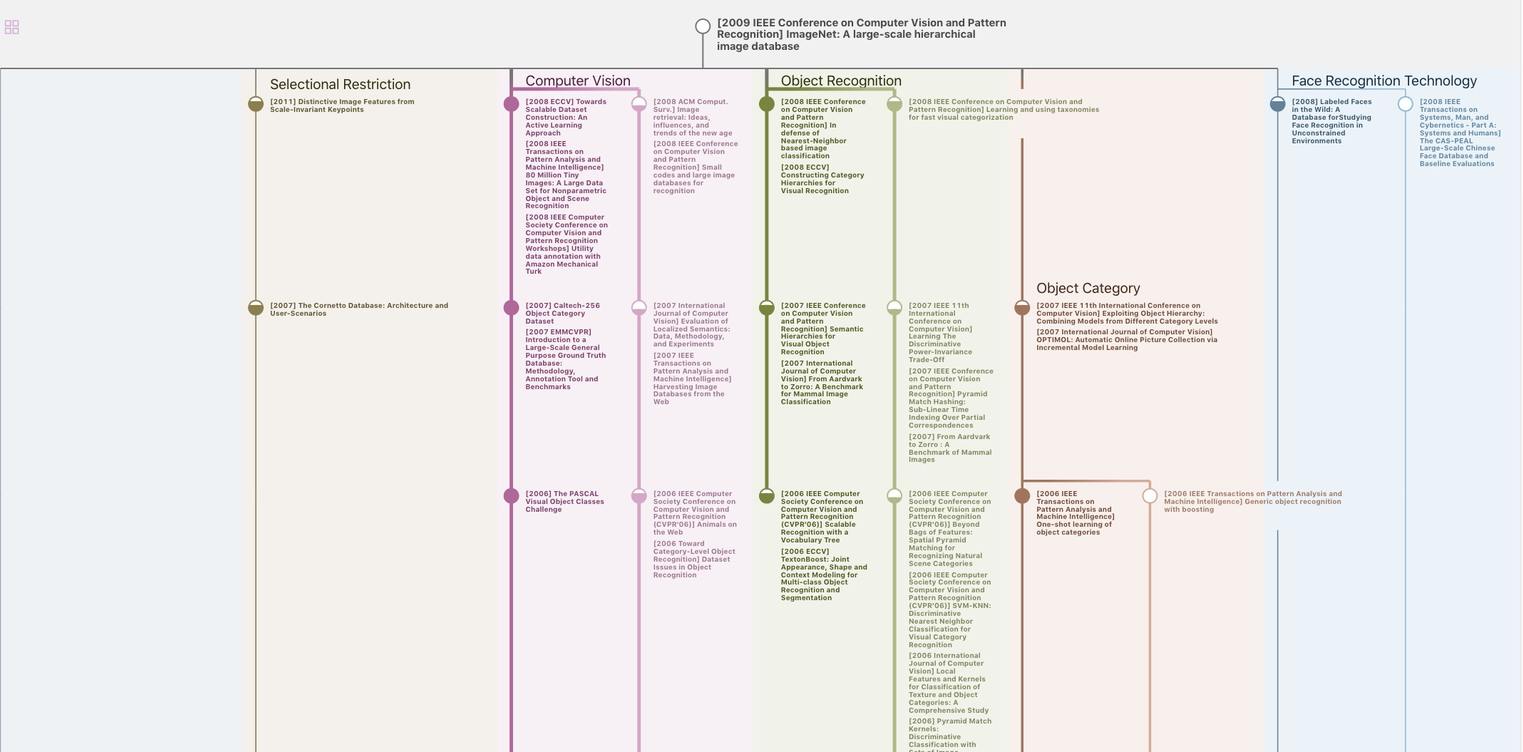
生成溯源树,研究论文发展脉络
Chat Paper
正在生成论文摘要