On the Trade-off between Over-smoothing and Over-squashing in Deep Graph Neural Networks
PROCEEDINGS OF THE 32ND ACM INTERNATIONAL CONFERENCE ON INFORMATION AND KNOWLEDGE MANAGEMENT, CIKM 2023(2023)
摘要
Graph Neural Networks (GNNs) have succeeded in various computer science applications, yet deep GNNs underperform their shallow counterparts despite deep learning's success in other domains. Over-smoothing and over-squashing are key challenges when stacking graph convolutional layers, hindering deep representation learning and information propagation from distant nodes. Our work reveals that over-smoothing and over-squashing are intrinsically related to the spectral gap of the graph Laplacian, resulting in an inevitable trade-off between these two issues, as they cannot be alleviated simultaneously. To achieve a suitable compromise, we propose adding and removing edges as a viable approach. We introduce the Stochastic Jost and Liu Curvature Rewiring (SJLR) algorithm, which is computationally efficient and preserves fundamental properties compared to previous curvature-based methods. Unlike existing approaches, SJLR performs edge addition and removal during GNN training while maintaining the graph unchanged during testing. Comprehensive comparisons demonstrate SJLR's competitive performance in addressing over-smoothing and over-squashing.
更多查看译文
关键词
Graph neural networks,over-smoothing,over-squashing,curvature
AI 理解论文
溯源树
样例
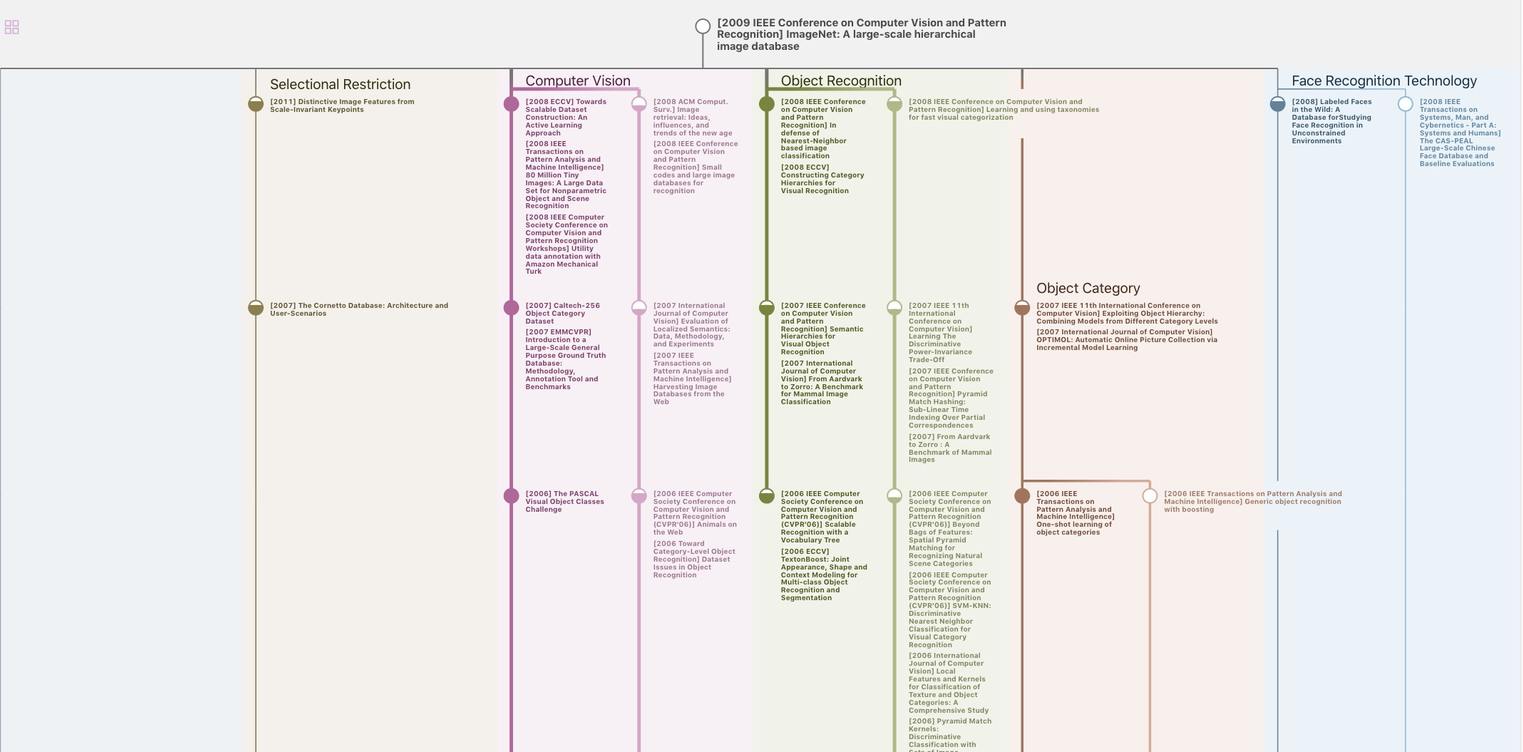
生成溯源树,研究论文发展脉络
Chat Paper
正在生成论文摘要