Multi-modal Mixture of Experts Represetation Learning for Sequential Recommendation
PROCEEDINGS OF THE 32ND ACM INTERNATIONAL CONFERENCE ON INFORMATION AND KNOWLEDGE MANAGEMENT, CIKM 2023(2023)
摘要
Within online platforms, it is critical to capture the dynamic user preference from the sequential interaction behaviors for making accurate recommendation over time. Recently, significant progress has been made in sequential recommendation with deep learning. However, existing neural sequential recommender often suffer from the data sparsity issue in real-world applications. To tackle this problem, we propose a Multi-Modal Mixture of experts model for Sequential Recommendation, named M3SRec, which leverage rich multi-modal interaction data for improving sequential recommendation. Different from existing multi-modal recommendation models, our approach jointly considers reducing the semantic gap across modalities and adapts multi-modal semantics to fit recommender systems. For this purpose, we make two important technical contributions in architecture and training. Firstly, we design a novel multi-modal mixture-of-experts (MoE) fusion network, which can deeply fuse the across-modal semantics and largely enhance the modeling capacity of complex user intents. For training, we design specific pre-training tasks that can mimic the goal of the recommendation, which help model learn the semantic relatedness between the multi-modal sequential context and the target item. Extensive experiments conducted on both public and industry datasets demonstrate the superiority of our proposed method over existing state-of-the-art methods, especially when only limited training data is available.
更多查看译文
关键词
User Behavior Modeling,Multi-modal Recommendation
AI 理解论文
溯源树
样例
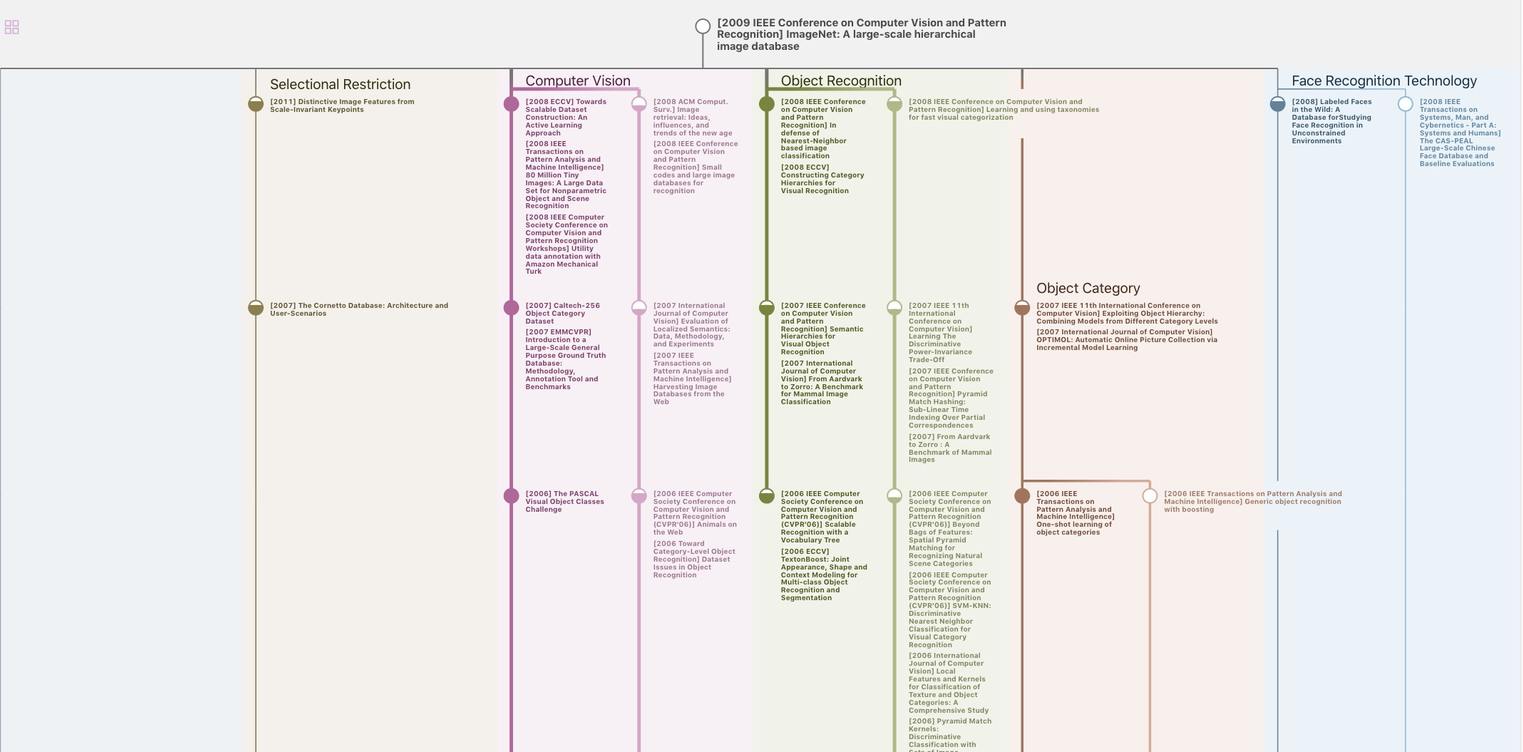
生成溯源树,研究论文发展脉络
Chat Paper
正在生成论文摘要