Clustering-property Matters: A Cluster-aware Network for Large Scale Multivariate Time Series Forecasting
PROCEEDINGS OF THE 32ND ACM INTERNATIONAL CONFERENCE ON INFORMATION AND KNOWLEDGE MANAGEMENT, CIKM 2023(2023)
摘要
Large-scale Multivariate Time Series (MTS) widely exist in various real-world systems, imposing significant demands on model efficiency. A recent work, STID, addressed the high complexity issue of popular Spatial-Temporal Graph Neural Networks (STGNNs). Despite its success, when applied to large-scale MTS data, the number of parameters of STID for modeling spatial dependencies increases substantially, leading to over-parameterization issues and suboptimal performance. These observations motivate us to explore new approaches for modeling spatial dependencies in a parameter-friendly manner. In this paper, we argue that the spatial properties of variables are essentially the superposition of multiple cluster centers. Accordingly, we propose a Cluster-Aware Network (CANet), which effectively captures spatial dependencies by mining the implicit cluster centers of variables. CANet solely optimizes the cluster centers instead of the spatial information of all nodes, thereby significantly reducing the parameter amount. Extensive experiments on two large-scale datasets validate our motivation and demonstrate the superiority of CANet.
更多查看译文
关键词
large-scale,multivariate time series forecasting,cluster centers
AI 理解论文
溯源树
样例
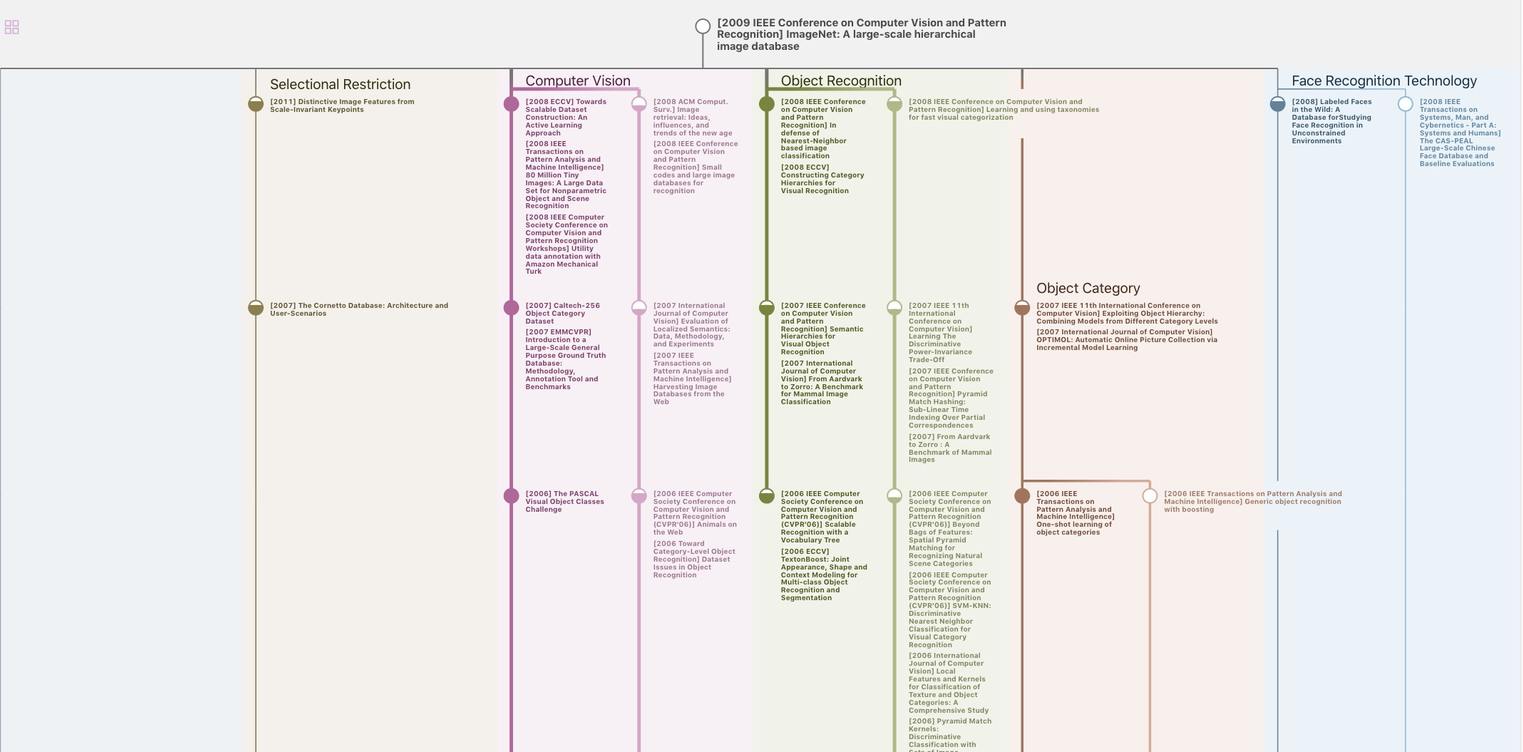
生成溯源树,研究论文发展脉络
Chat Paper
正在生成论文摘要