Learning to Simulate Complex Physical Systems: A Case Study
PROCEEDINGS OF THE 32ND ACM INTERNATIONAL CONFERENCE ON INFORMATION AND KNOWLEDGE MANAGEMENT, CIKM 2023(2023)
摘要
Complex physical system simulation is important in many real world applications. We study the general simulation scenario to generate the response result when a physical object is applied by external factors. Traditional solvers on Partial Differential Equations (PDEs) suffer from significantly high computational cost. Many recent learning-based approaches focus on multivariate time series alike simulation prediction problem and do not work for our case. In this paper, we propose a novel two-level graph neural networks (GNNs) to learn the simulation result of a physical object applied by external factors. The key is a two-level graph structure where one fine mesh graph is mapped to multiple coarse one. Our preliminary evaluation on both synthetic and real datasets demonstrates that our work outperforms three state-of-the-arts by much lower errors.
更多查看译文
关键词
Graph Neural Networks,Complex Physical Systems,Simulation
AI 理解论文
溯源树
样例
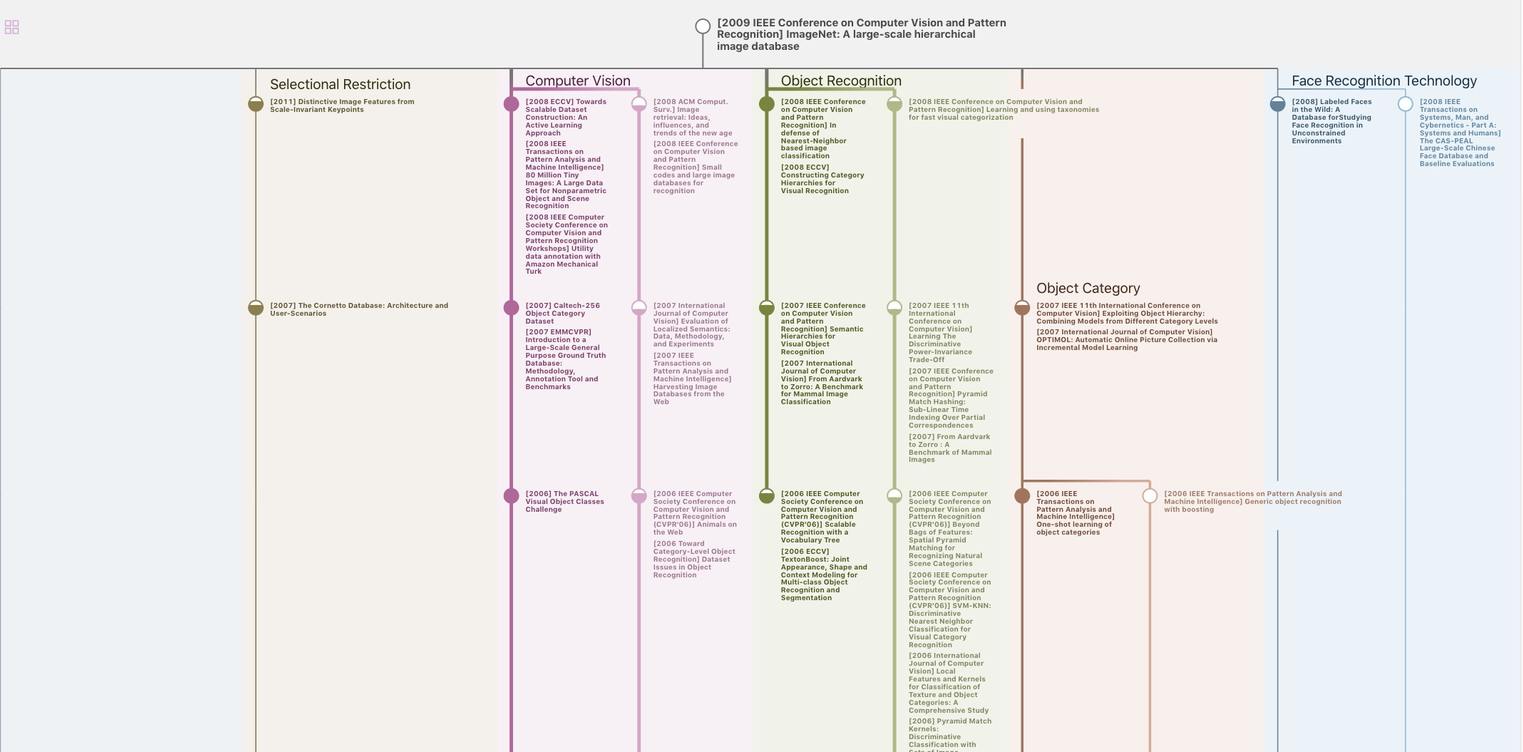
生成溯源树,研究论文发展脉络
Chat Paper
正在生成论文摘要