Epistemic Injustice in Online Communities: Unpacking the Values of Knowledge Creation and Curation Within CSCW Applications
Conference on Computer Supported Cooperative Work (CSCW)(2023)CCF A
University of Minnesota | Georgia Institute of Technology | Northeastern University | UC Davis | Wikimedia Foundation
Abstract
Information flows are pervasive on the internet and often have a low entry barrier. From an epistemological perspective, information evolves into knowledge. For example, information about mental health on TikTok can act as actionable knowledge for someone seeking to improve their mental health. However, social computing has long known that people do not interact with knowledge cleanly, especially in digital environments. While knowledge curation is essential for targeting irrelevant, biased, or even harmful information, it is value-laden; in choosing how to present information, we undermine non-traditional information such as personal experiences. In this workshop, we will bring together researchers from academia, industry, and marginalized communities to discuss how current CSCW applications contribute to the systemic silencing, exclusion, or delegitimization of certain knowledge contributions (i.e., epistemic injustice). We will diagram our own mental models of how knowledge is created and curated and reflect on critical questions to orient the design of inclusive knowledge spaces online, particularly with topics that blend personal experience with factual information, such as mental health.
MoreTranslated text
求助PDF
上传PDF
View via Publisher
AI Read Science
AI Summary
AI Summary is the key point extracted automatically understanding the full text of the paper, including the background, methods, results, conclusions, icons and other key content, so that you can get the outline of the paper at a glance.
Example
Background
Key content
Introduction
Methods
Results
Related work
Fund
Key content
- Pretraining has recently greatly promoted the development of natural language processing (NLP)
- We show that M6 outperforms the baselines in multimodal downstream tasks, and the large M6 with 10 parameters can reach a better performance
- We propose a method called M6 that is able to process information of multiple modalities and perform both single-modal and cross-modal understanding and generation
- The model is scaled to large model with 10 billion parameters with sophisticated deployment, and the 10 -parameter M6-large is the largest pretrained model in Chinese
- Experimental results show that our proposed M6 outperforms the baseline in a number of downstream tasks concerning both single modality and multiple modalities We will continue the pretraining of extremely large models by increasing data to explore the limit of its performance
Upload PDF to Generate Summary
Must-Reading Tree
Example
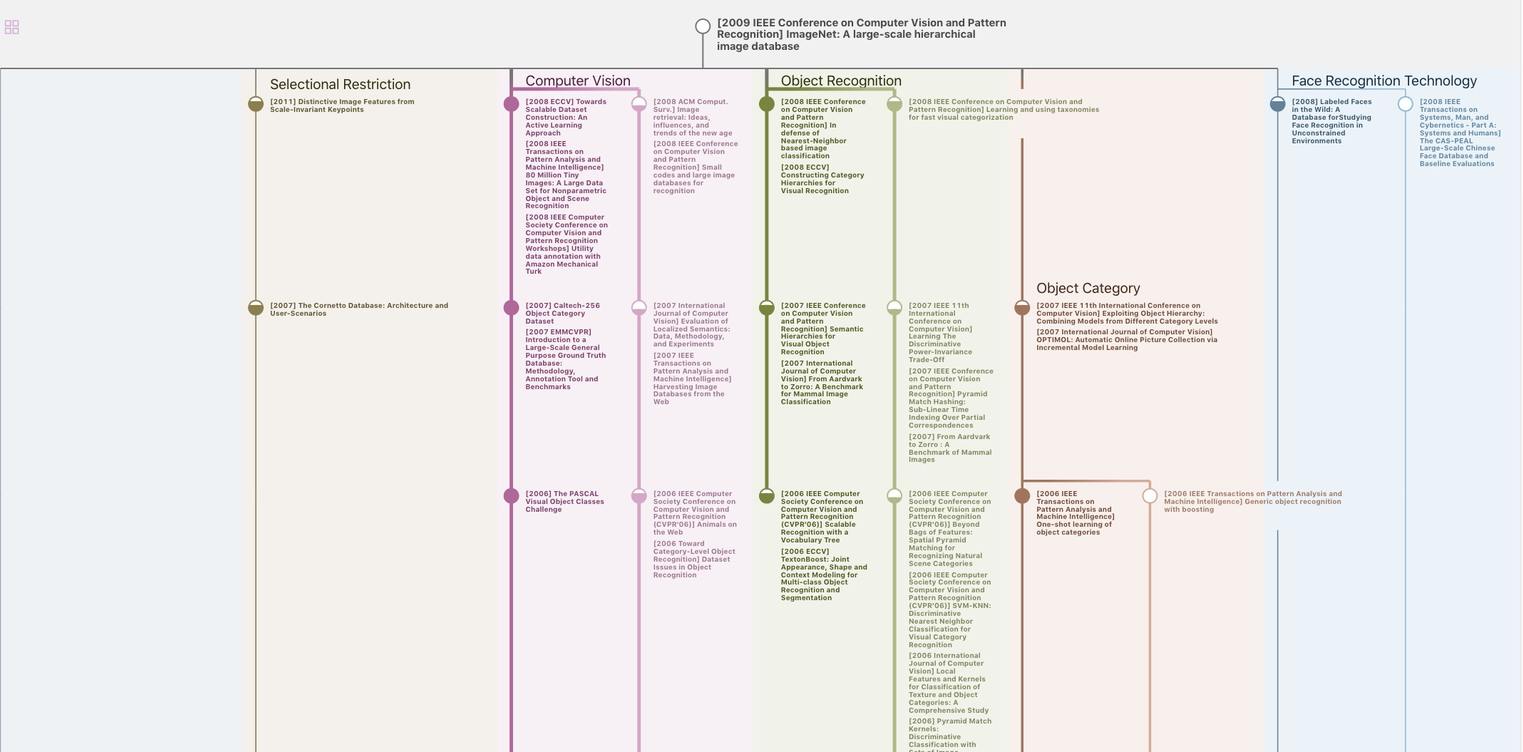
Generate MRT to find the research sequence of this paper
Data Disclaimer
The page data are from open Internet sources, cooperative publishers and automatic analysis results through AI technology. We do not make any commitments and guarantees for the validity, accuracy, correctness, reliability, completeness and timeliness of the page data. If you have any questions, please contact us by email: report@aminer.cn
Chat Paper