A Hierarchical Imitation Learning-based Decision Framework for Autonomous Driving
PROCEEDINGS OF THE 32ND ACM INTERNATIONAL CONFERENCE ON INFORMATION AND KNOWLEDGE MANAGEMENT, CIKM 2023(2023)
摘要
In this paper, we focus on the decision-making challenge in autonomous driving, a central and intricate problem influencing the safety and practicality of autonomous vehicles. We propose an innovative hierarchical imitation learning framework that effectively alleviates the complexity of learning in autonomous driving decision-making problems by decoupling decision-making tasks into sub-problems. Specifically, the decision-making process is divided into two levels of sub-problems: the upper level directs the vehicle's lane selection and qualitative speed management, while the lower level implements precise control of the driving speed and direction. We harness Transformer-based models for solving each sub-problem, enabling overall hierarchical framework to comprehend and navigate diverse and various road conditions, ultimately resulting in improved decision-making. Through an evaluation in several typical driving scenarios within the SMARTS autonomous driving simulation environment, our proposed hierarchical decision-making framework significantly outperforms end-to-end reinforcement learning algorithms and behavior cloning algorithm, achieving an average pass rate of over 90%. Our framework's effectiveness is substantiated by its commendable achievements at the NeurIPS 2022 Driving SMARTS competition, where it secures dual track championships.
更多查看译文
关键词
Machine Learning,Autonomous Driving,Imitation Learning
AI 理解论文
溯源树
样例
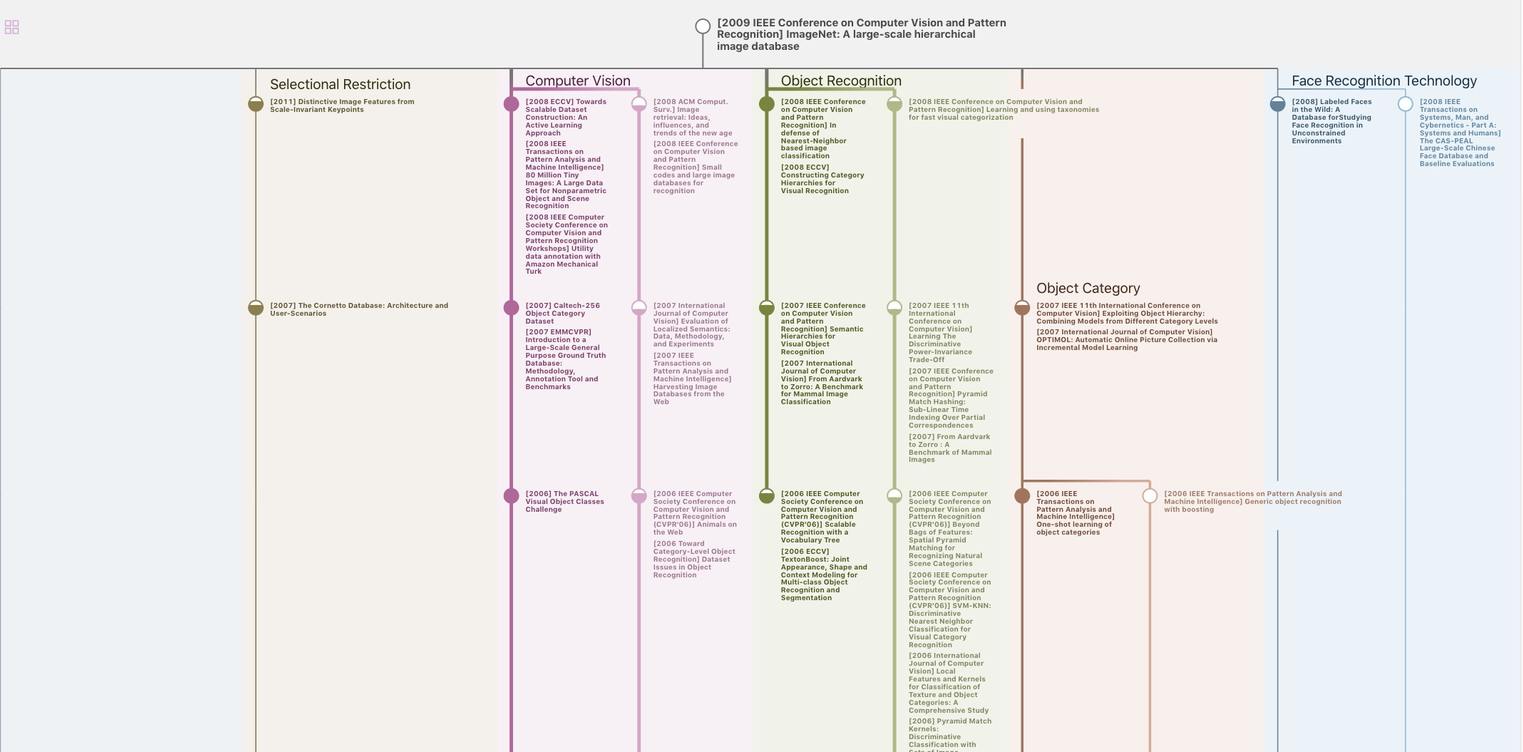
生成溯源树,研究论文发展脉络
Chat Paper
正在生成论文摘要