Build Faster with Less: A Journey to Accelerate Sparse Model Building for Semantic Matching in Product Search
PROCEEDINGS OF THE 32ND ACM INTERNATIONAL CONFERENCE ON INFORMATION AND KNOWLEDGE MANAGEMENT, CIKM 2023(2023)
Abstract
The semantic matching problem in product search seeks to retrieve all semantically relevant products given a user query. Recent studies have shown that extreme multi-label classification (XMC) model enjoys both low inference latency and high recall in real-world scenarios. These XMC semantic matching models adopt TF-IDF vectorizers to extract query text features and use mainly sparse matrices for the model weights. However, limited availability of libraries for efficient parallel sparse modules may lead to tediously long model building time when the problem scales to hundreds of millions of labels. This incurs significant hardware cost and renders the semantic model stale even before it is deployed. In this paper, we investigate and accelerate the model building procedures in a tree-based XMC model. On a real-world semantic matching task with 100M labels, our enhancements achieve over 10 times acceleration (from 3.1 days to 6.7 hours) while reducing hardware cost by 25%.
MoreTranslated text
Key words
extreme multi-label classification,product search
AI Read Science
Must-Reading Tree
Example
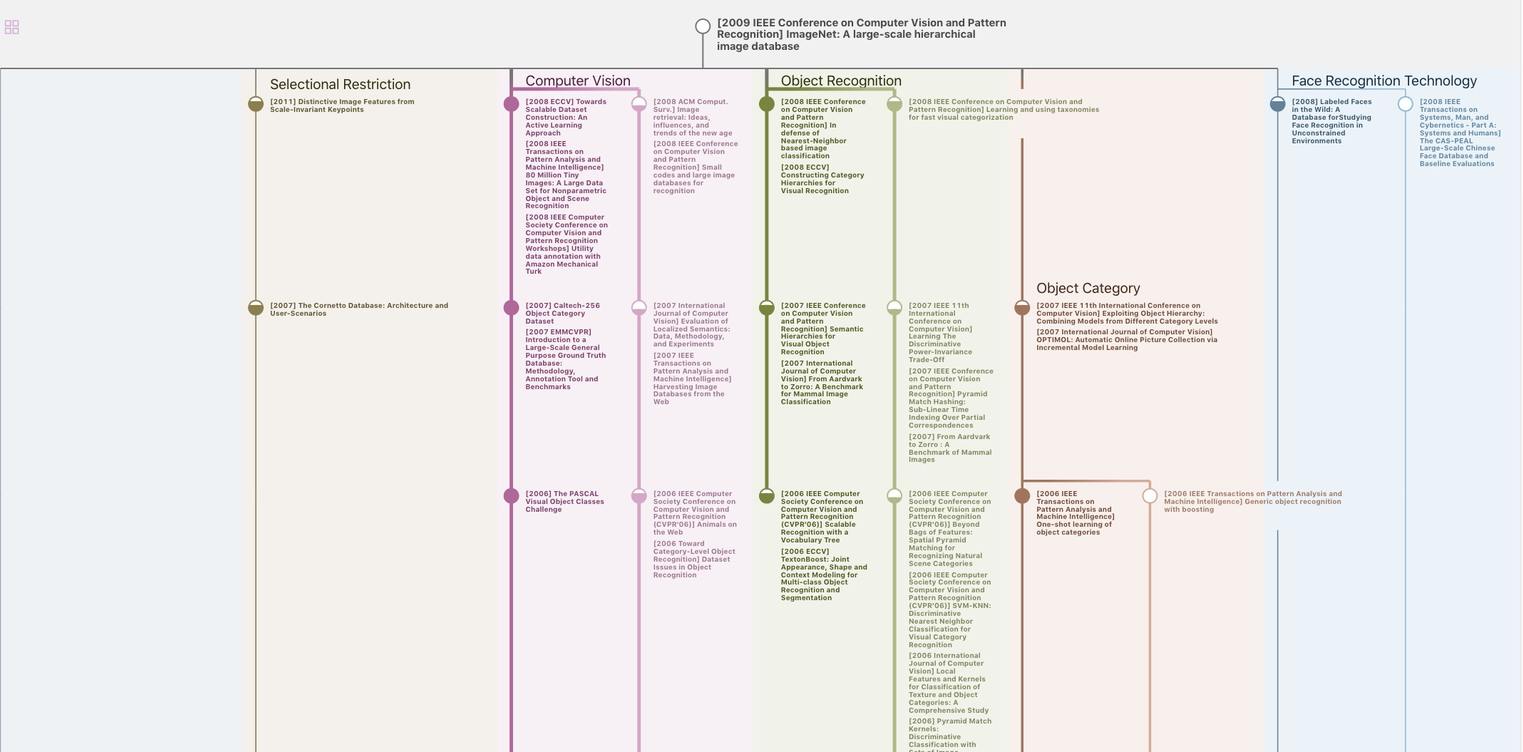
Generate MRT to find the research sequence of this paper
Chat Paper
Summary is being generated by the instructions you defined