GraphMaker: Can Diffusion Models Generate Large Attributed Graphs?
CoRR(2023)
Abstract
Large-scale graphs with node attributes are fundamental in real-world scenarios, such as social and financial networks. The generation of synthetic graphs that emulate real-world ones is pivotal in graph machine learning, aiding network evolution understanding and data utility preservation when original data cannot be shared. Traditional models for graph generation suffer from limited model capacity. Recent developments in diffusion models have shown promise in merely graph structure generation or the generation of small molecular graphs with attributes. However, their applicability to large attributed graphs remains unaddressed due to challenges in capturing intricate patterns and scalability. This paper introduces GraphMaker, a novel diffusion model tailored for generating large attributed graphs. We study the diffusion models that either couple or decouple graph structure and node attribute generation to address their complex correlation. We also employ node-level conditioning and adopt a minibatch strategy for scalability. We further propose a new evaluation pipeline using models trained on generated synthetic graphs and tested on original graphs to evaluate the quality of synthetic data. Empirical evaluations on real-world datasets showcase GraphMaker's superiority in generating realistic and diverse large-attributed graphs beneficial for downstream tasks.
MoreTranslated text
Key words
large attributed graphs,diffusion models
AI Read Science
Must-Reading Tree
Example
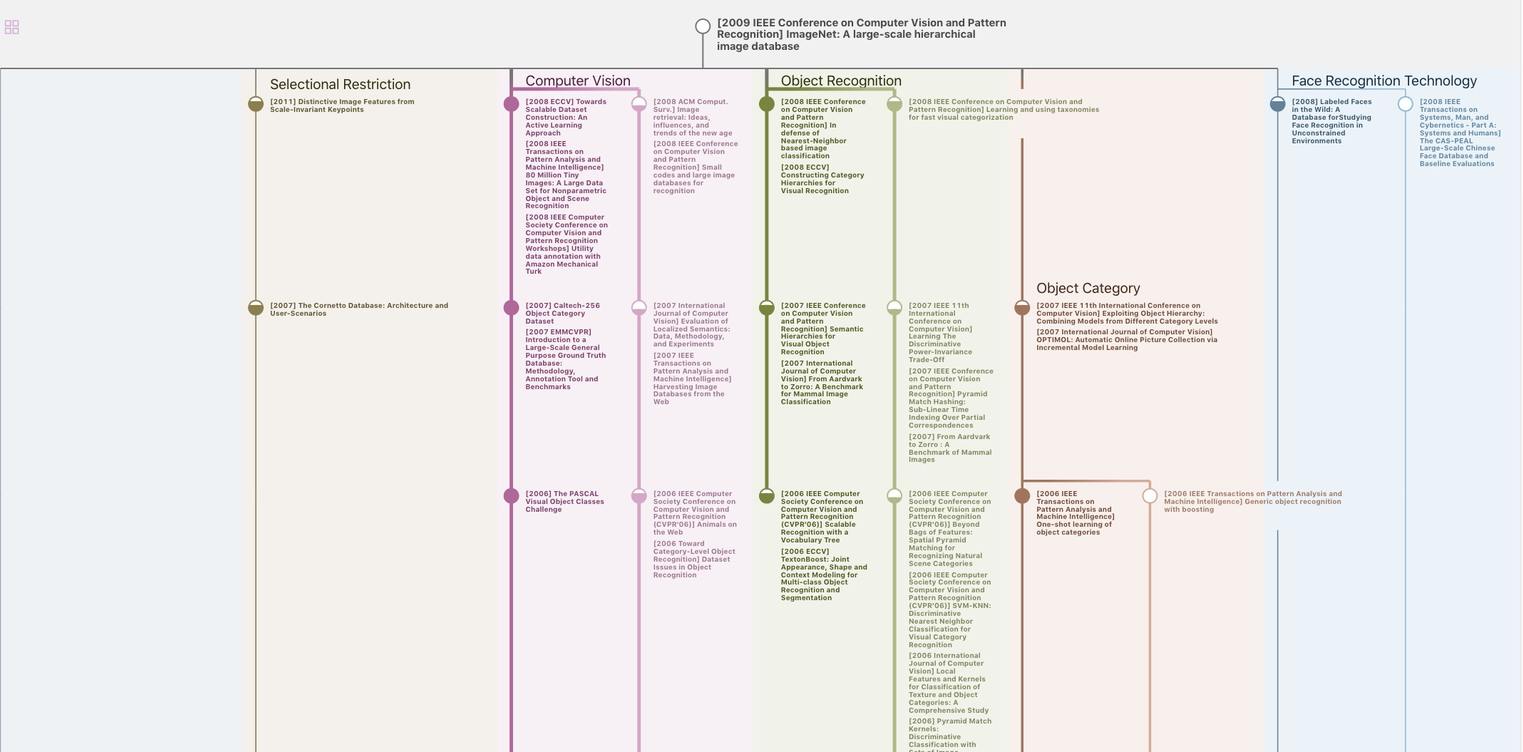
Generate MRT to find the research sequence of this paper
Chat Paper
Summary is being generated by the instructions you defined