Foundation Model's Embedded Representations May Detect Distribution Shift
arxiv(2023)
摘要
Sampling biases can cause distribution shifts between train and test datasetsfor supervised learning tasks, obscuring our ability to understand thegeneralization capacity of a model. This is especially important consideringthe wide adoption of pre-trained foundational neural networks – whose behaviorremains poorly understood – for transfer learning (TL) tasks. We present acase study for TL on the Sentiment140 dataset and show that many pre-trainedfoundation models encode different representations of Sentiment140's manuallycurated test set M from the automatically labeled training set P,confirming that a distribution shift has occurred. We argue training on P andmeasuring performance on M is a biased measure of generalization. Experimentson pre-trained GPT-2 show that the features learnable from P do not improve(and in fact hamper) performance on M. Linear probes on pre-trained GPT-2'srepresentations are robust and may even outperform overall fine-tuning,implying a fundamental importance for discerning distribution shift intrain/test splits for model interpretation.
更多查看译文
AI 理解论文
溯源树
样例
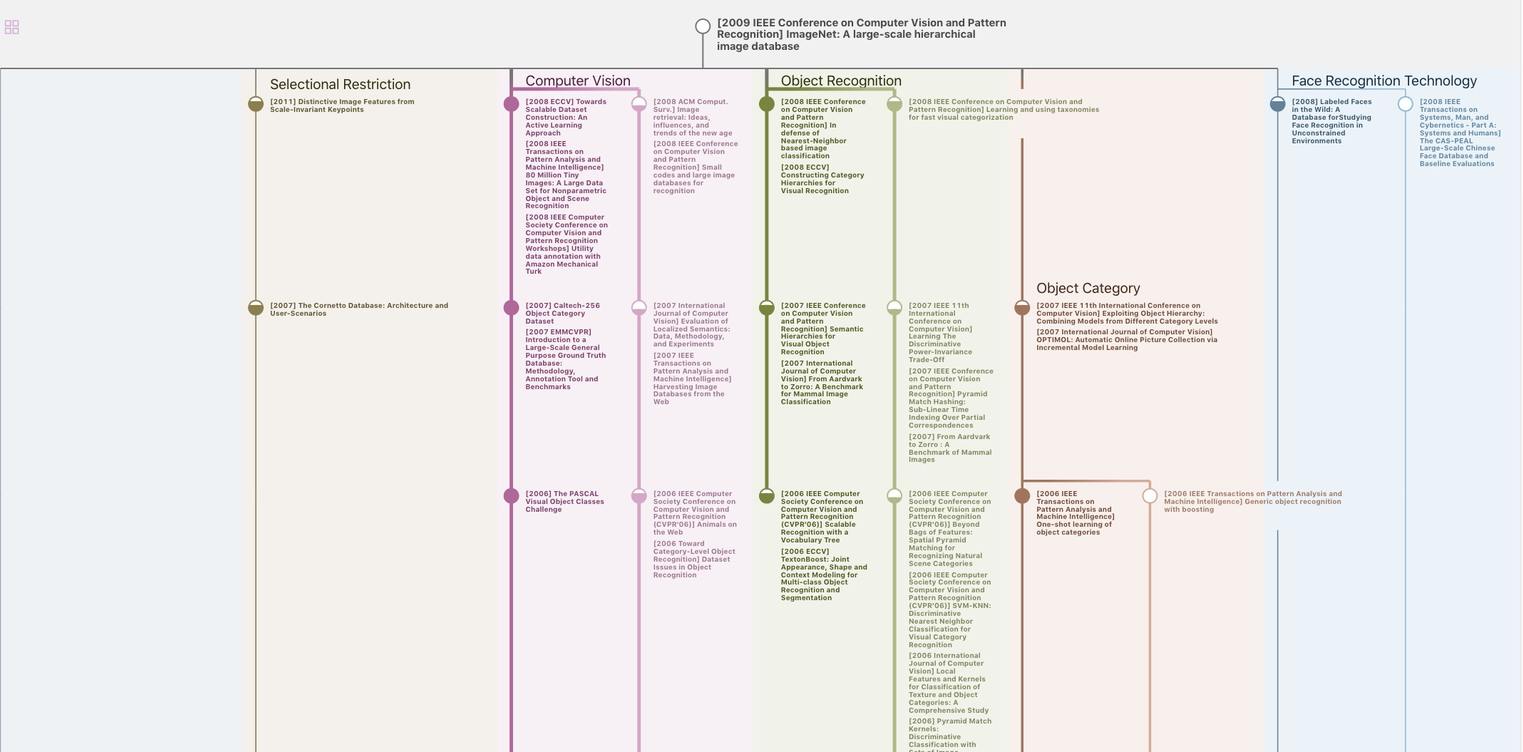
生成溯源树,研究论文发展脉络
Chat Paper
正在生成论文摘要