The Hidden Adversarial Vulnerabilities of Medical Federated Learning
CoRR(2023)
摘要
In this paper, we delve into the susceptibility of federated medical image analysis systems to adversarial attacks. Our analysis uncovers a novel exploitation avenue: using gradient information from prior global model updates, adversaries can enhance the efficiency and transferability of their attacks. Specifically, we demonstrate that single-step attacks (e.g. FGSM), when aptly initialized, can outperform the efficiency of their iterative counterparts but with reduced computational demand. Our findings underscore the need to revisit our understanding of AI security in federated healthcare settings.
更多查看译文
关键词
medical federated learning,federated learning,hidden adversarial vulnerabilities
AI 理解论文
溯源树
样例
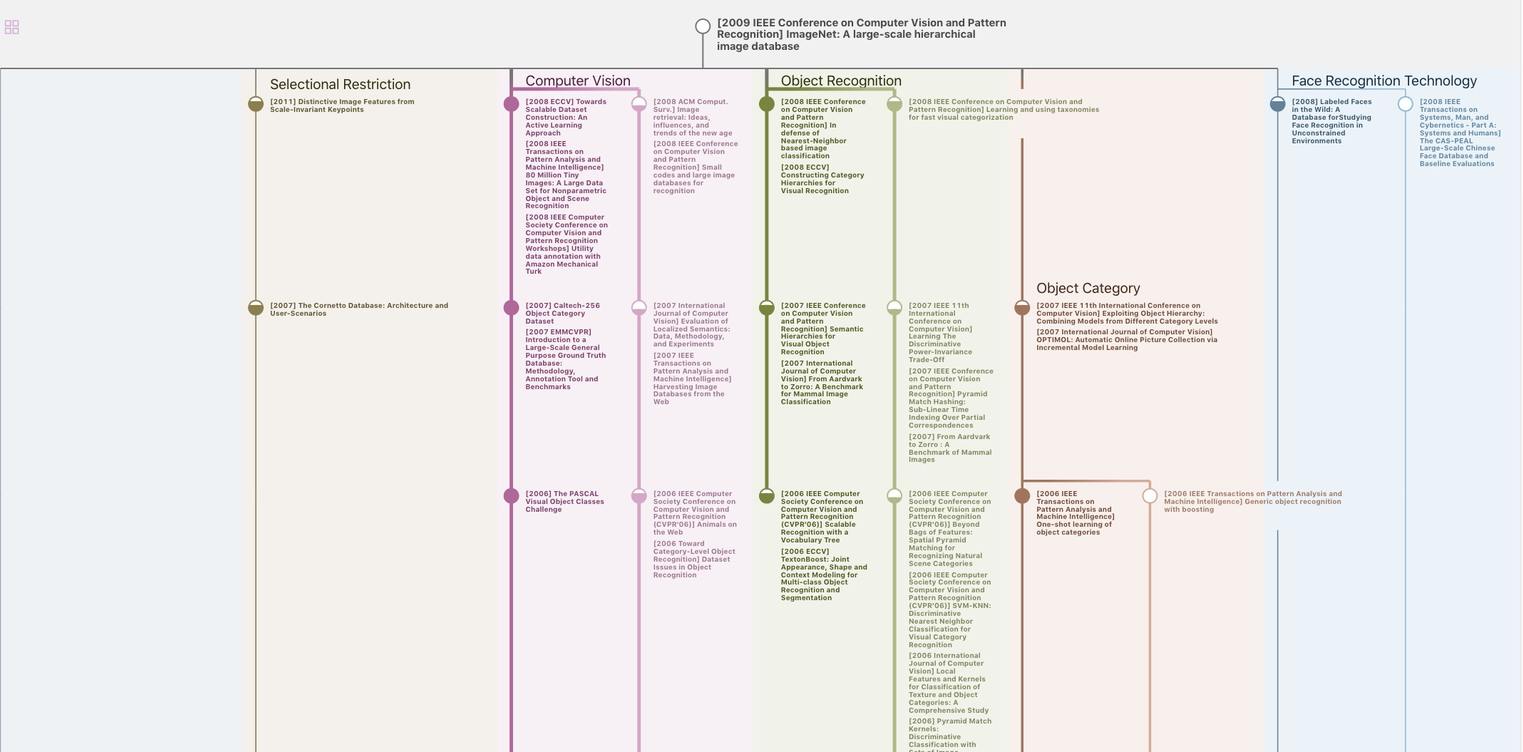
生成溯源树,研究论文发展脉络
Chat Paper
正在生成论文摘要