Structural generalization in COGS: Supertagging is (almost) all you need.
CoRR(2023)
摘要
In many Natural Language Processing applications, neural networks have been found to fail to generalize on out-of-distribution examples. In particular, several recent semantic parsing datasets have put forward important limitations of neural networks in cases where compositional generalization is required. In this work, we extend a neural graph-based semantic parsing framework in several ways to alleviate this issue. Notably, we propose: (1) the introduction of a supertagging step with valency constraints, expressed as an integer linear program; (2) a reduction of the graph prediction problem to the maximum matching problem; (3) the design of an incremental early-stopping training strategy to prevent overfitting. Experimentally, our approach significantly improves results on examples that require structural generalization in the COGS dataset, a known challenging benchmark for compositional generalization. Overall, our results confirm that structural constraints are important for generalization in semantic parsing.
更多查看译文
关键词
supertagging,structural generalization,cogs
AI 理解论文
溯源树
样例
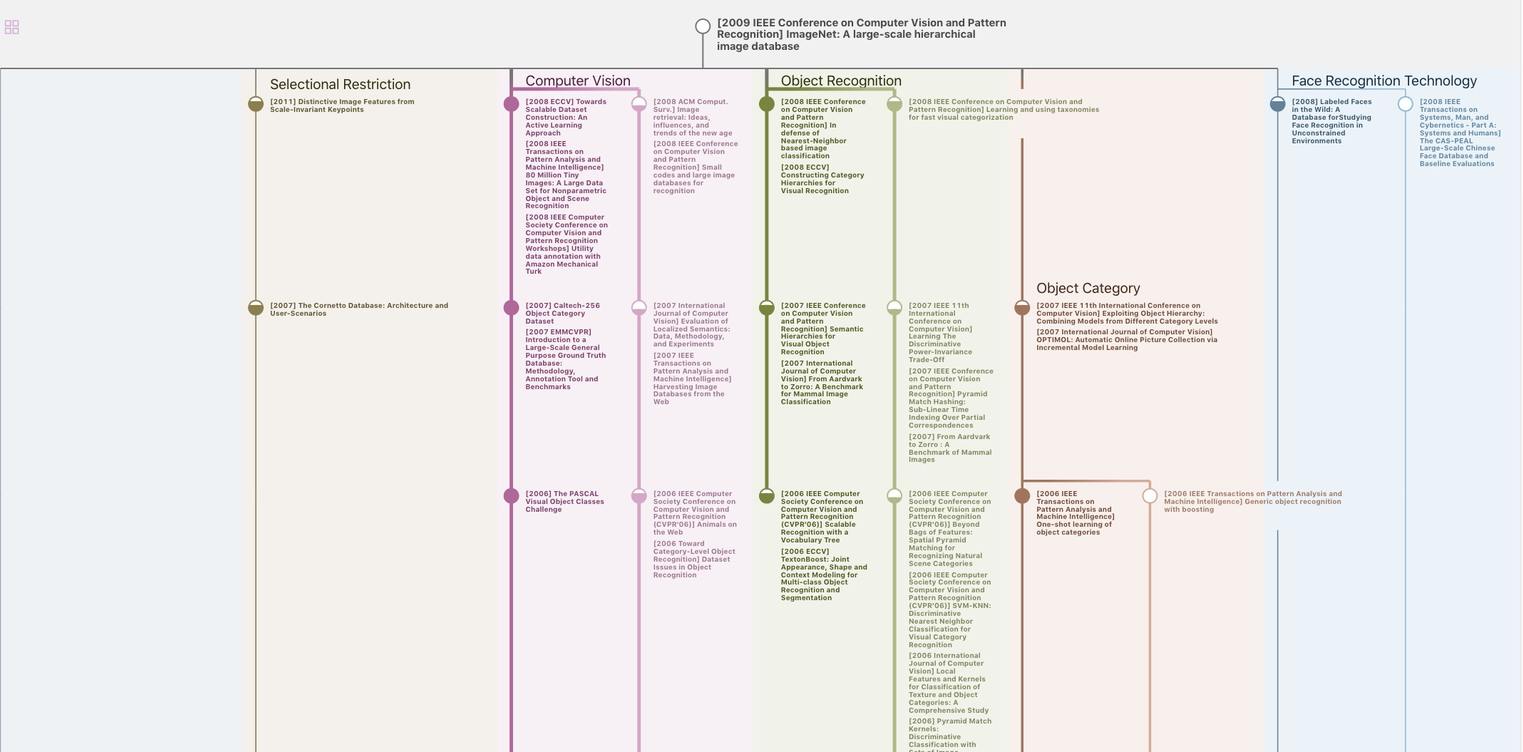
生成溯源树,研究论文发展脉络
Chat Paper
正在生成论文摘要