Randomized Forward Mode of Automatic Differentiation For Optimization Algorithms
CoRR(2023)
摘要
We present a randomized forward mode gradient (RFG) as an alternative to
backpropagation. RFG is a random estimator for the gradient that is constructed
based on the directional derivative along a random vector. The forward mode
automatic differentiation (AD) provides an efficient computation of RFG. The
probability distribution of the random vector determines the statistical
properties of RFG. Through the second moment analysis, we found that the
distribution with the smallest kurtosis yields the smallest expected relative
squared error. By replacing gradient with RFG, a class of RFG-based
optimization algorithms is obtained. By focusing on gradient descent (GD) and
Polyak's heavy ball (PHB) methods, we present a convergence analysis of
RFG-based optimization algorithms for quadratic functions. Computational
experiments are presented to demonstrate the performance of the proposed
algorithms and verify the theoretical findings.
更多查看译文
关键词
automatic differentiation,optimization
AI 理解论文
溯源树
样例
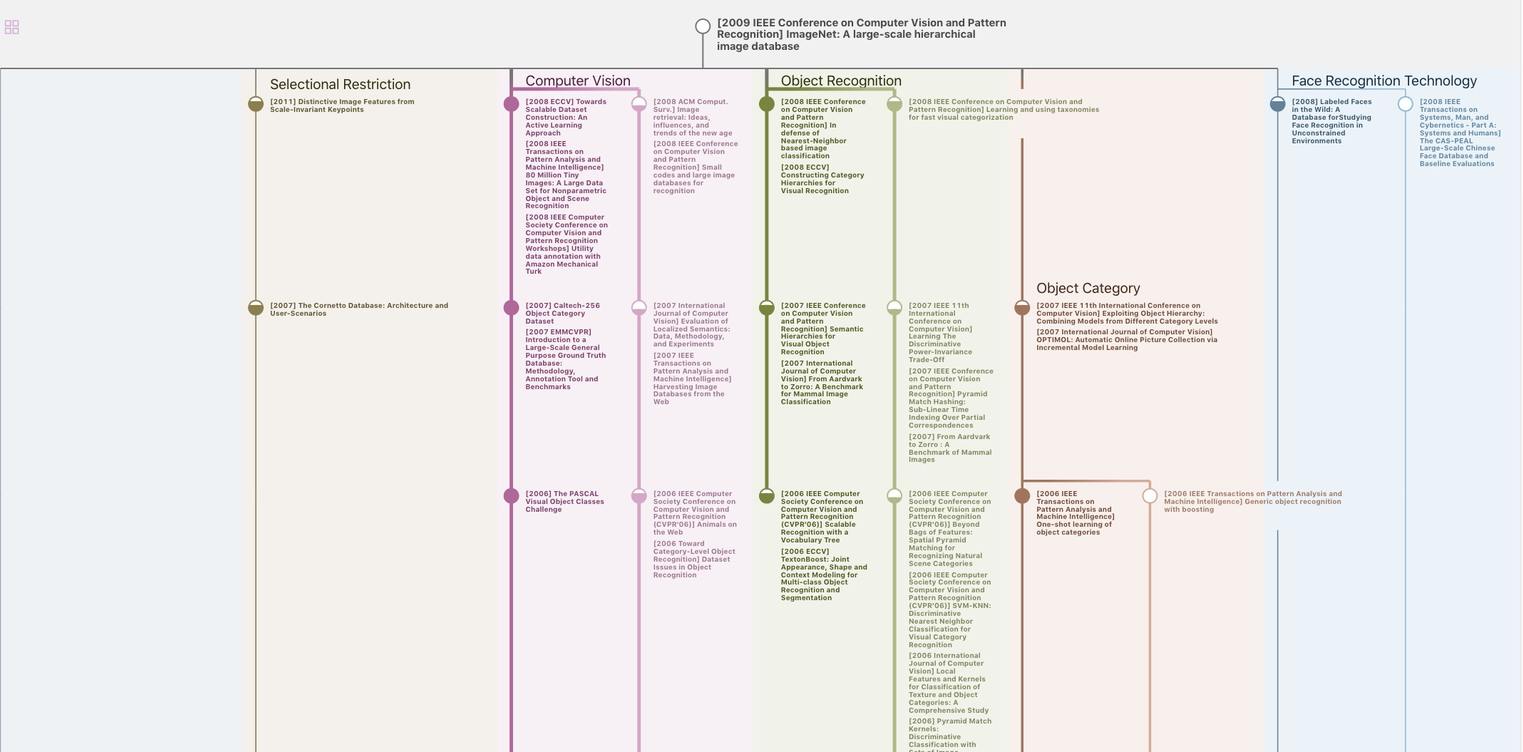
生成溯源树,研究论文发展脉络
Chat Paper
正在生成论文摘要